Deep Neural Network Based Channel Estimation for Massive MIMO-OFDM Systems With Imperfect Channel State Information
IEEE SYSTEMS JOURNAL(2022)
摘要
Massive multi-input multi-output (MIMO) has aroused extensive interest in communication industry, as it can effectively increase communication system capacity and reduce transmit power. For massive MIMO-orthogonal frequency division multiplexing (OFDM) systems, traditional pilot assisted channel estimation methods need to obtain the channel response of data subcarriers by interpolation. However, when the channel response of continuous data subcarriers changes dramatically, the accuracy of channel estimation will be decreased. In this article, therefore, a novel channel prediction framework that integrates the imperfect channel estimation of the massive MIMO-OFDM into the deep neural network scheme is proposed, thus replacing the interpolation method and achieving higher accuracy of channel estimation. From algorithm analysis and simulation results, the performance of proposed deep neural network channel prediction method based on the imperfect channel estimation outperforms the conventional least square method based on interpolation. Furthermore, compared with minimum mean square error, there are no need for channel autocorrelation matrix, prior statistics of noise variance, and the complex matrix inversion operations. In summary, deep neural network is an efficient method for the imperfect channel estimation in massive MIMO-OFDM systems.
更多查看译文
关键词
Channel estimation, Interpolation, Neural networks, Massive MIMO, Deep learning, Transmitting antennas, Training, Bit error rate (BER), channel estimation, channel state information (CSI), deep neural network (DNN), massive multi-input multioutput (MIMO)
AI 理解论文
溯源树
样例
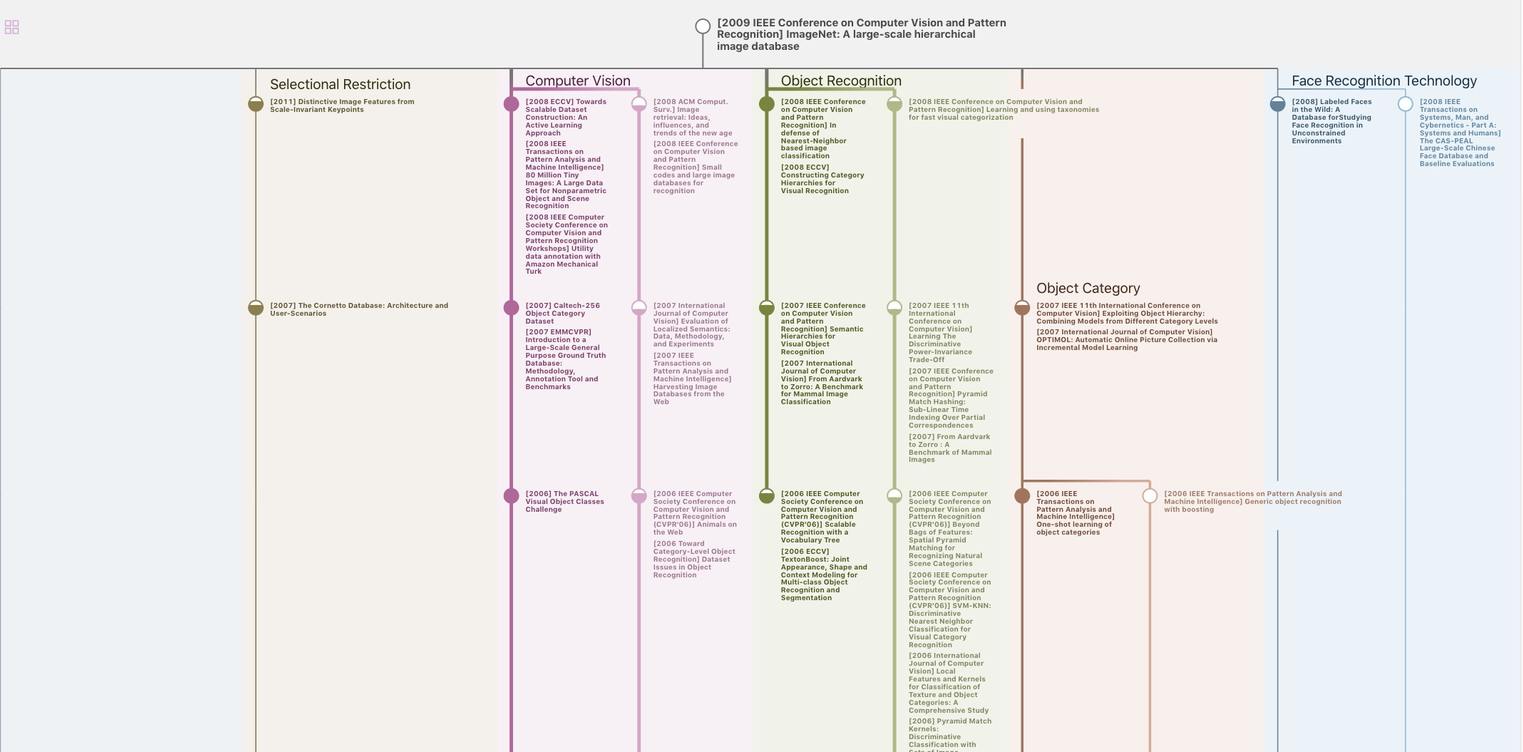
生成溯源树,研究论文发展脉络
Chat Paper
正在生成论文摘要