RanPaste: Paste Consistency and Pseudo Label for Semisupervised Remote Sensing Image Semantic Segmentation
IEEE Transactions on Geoscience and Remote Sensing(2022)
摘要
With the development of deep learning, remote sensing (RS) image segmentation has been applied with marked success. However, in the process of model training, the large number of labeled images required more expensive annotation. A key challenge is how to make full use of extensive unlabeled images available to improve the segmentation model. In this article, we propose a semisupervised remote sensing image semantic segmentation method defined as RanPaste, which combines labeled images with unlabeled images to improve segmentation performance. First, we obtain pseudo label by randomly pasting part of the ground truth label into the predicted segmentation map. Then, we combine the labeled and unlabeled images to generate rough predictions after strong augmentation. Finally, by using the semisupervised loss, we achieve better performance on remote sensing image segmentation. Our method combines consistency regularization and pseudo label and then utilizes thresholds to gradually improve the model performance. RanPaste enables the model to learn more underlying information in the unlabeled data. Experimental results on six datasets show that RanPaste can learn more latent information from unlabeled data to improve segmentation performance. Besides, our approach achieves better segmentation results on different network structures and datasets.
更多查看译文
关键词
Convolutional neural network,remote sensing (RS),semantic segmentation,semisupervised learning
AI 理解论文
溯源树
样例
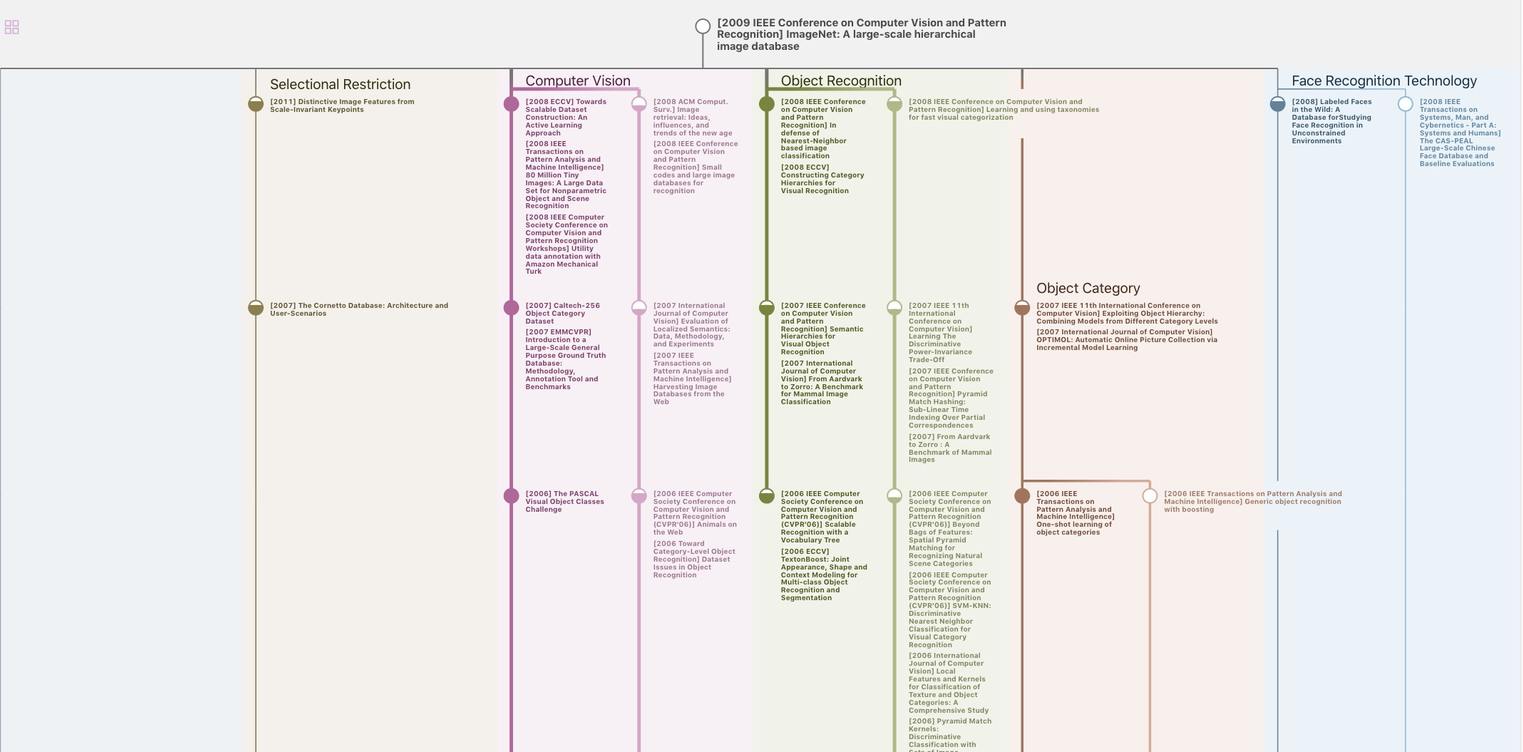
生成溯源树,研究论文发展脉络
Chat Paper
正在生成论文摘要