Software Escalation Prediction Based on Deep Learning in the Cognitive Internet of Vehicles
IEEE TRANSACTIONS ON INTELLIGENT TRANSPORTATION SYSTEMS(2022)
摘要
In the Cognitive Internet of Vehicles (CIoV), vehicles, road side units (RSU) and other key nodes have been equipped with more and more software to support intelligent transportation system (ITS), vehicle automatic control and intelligent road information services. Additionally, technological innovation forces the software in the CIoV to update and upgrade in time. However, escalation is critical to the safety, stability, and maintenance cost of transportation systems. It can be assumed that when the intelligent services supporting CIoV can realize self-perception and escalation, the cognitive ability and coordination ability of the entire CIoV will be greatly improved. To address this, we first propose a deep learning-based method for Software Escalation Prediction (SEP) in CIoV. Specifically, the pretraining mechanism of transformers in the field of natural language processing is combined with software upgrade-related events to dynamically model software sequence activities. To capture the event association in the software activities, we use graph modeling software's state log and utilize a graph neural network (GNN) to learn the complex life activity rule of software. Finally, the above characteristics are deeply integrated. The proposed method has a 6%-8% improvement over the RoBERTa methods.
更多查看译文
关键词
Software, Maintenance engineering, Sensors, Vehicular ad hoc networks, Deep learning, Internet of Things, Computer architecture, Internet of Vehicles, software maintenance, cognitive, deep learning
AI 理解论文
溯源树
样例
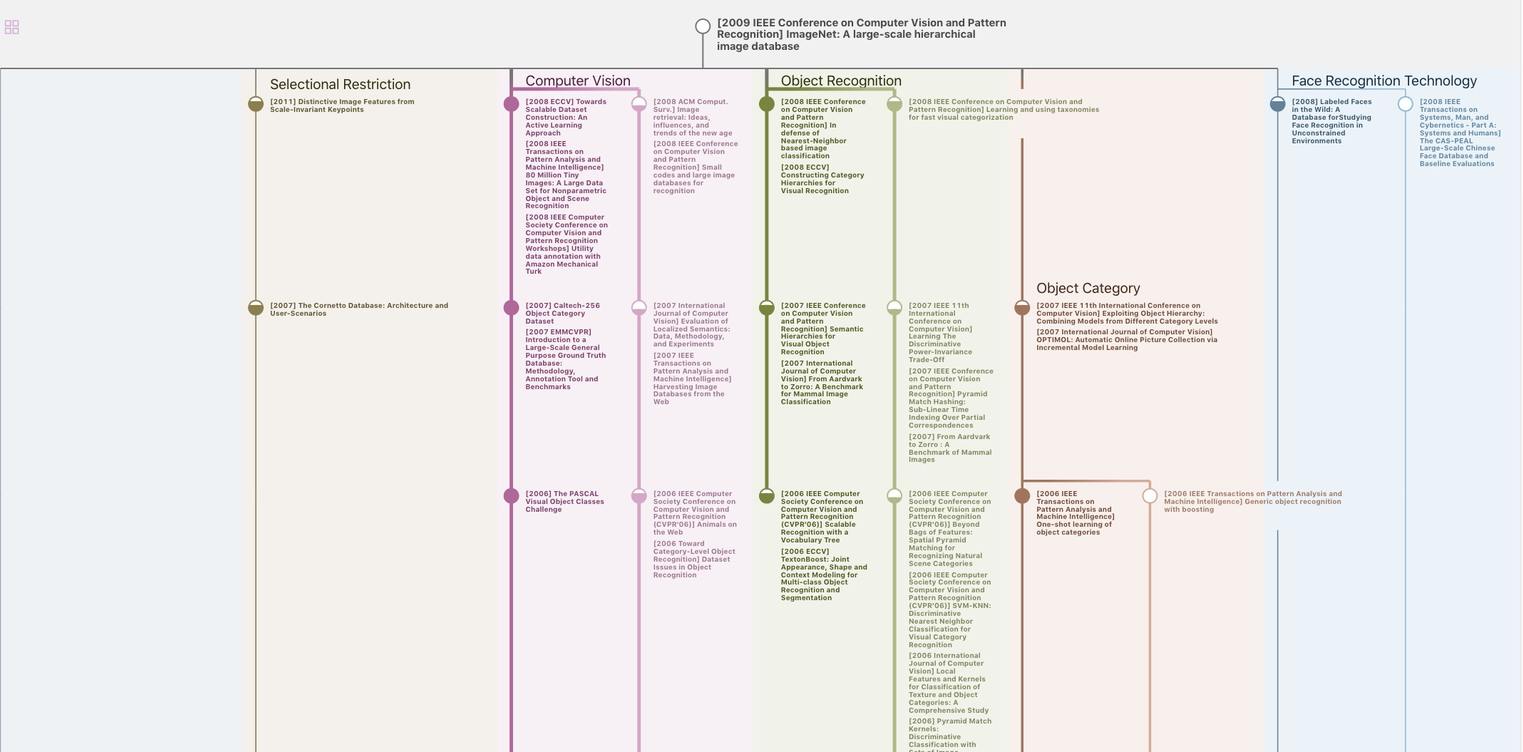
生成溯源树,研究论文发展脉络
Chat Paper
正在生成论文摘要