Semantic SLAM Based on Improved DeepLabv3(+) in Dynamic Scenarios
IEEE ACCESS(2022)
摘要
Simultaneous Localization and Mapping (SLAM) plays an irreplaceable role in the field of artificial intelligence. The traditional visual SLAM algorithm is stable assuming a static environment, but has lower robustness and accuracy in dynamic scenes, which affects its localization accuracy. To address this problem, a semantic SLAM system is proposed that incorporates ORB-SLAM3, semantic segmentation thread and geometric thread, namely DeepLabv3(+)_SLAM. The improved DeepLabv3(+) semantic segmentation network combines context information to segment potential a priori dynamic objects. Then, the geometry thread uses a multi-view geometry method to detect the motion state information of the dynamic object. Finally, a new ant colony strategy is proposed to find the group of all dynamic feature points through the optimal path, and avoids traversing all the feature points to reduce the dynamic object detection time and improve the real-time performance of the system. By conducting experiments on public data sets, the results show that the method proposed in this paper effectively improves the positioning accuracy of the system in a high-dynamic environment compared with similar algorithms, and the real-time performance of the system is improved.
更多查看译文
关键词
DeepLabv3(+)_SLAM, semantic, high-dynamic environment, new ant colony strategy
AI 理解论文
溯源树
样例
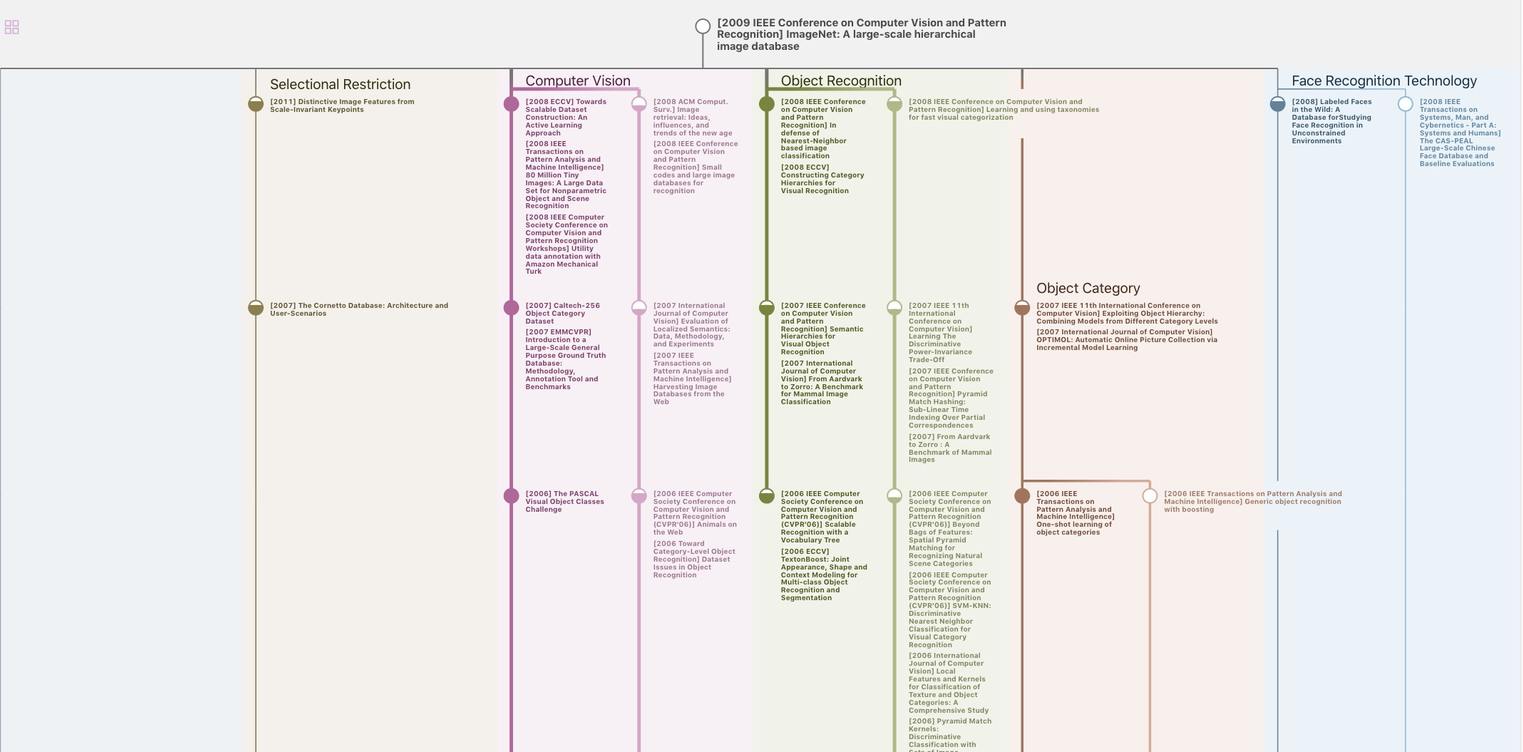
生成溯源树,研究论文发展脉络
Chat Paper
正在生成论文摘要