Machine learning in solid heterogeneous catalysis: Recent developments, challenges and perspectives
CHEMICAL ENGINEERING SCIENCE(2022)
摘要
Recently, the availability of extensive catalysis-related data generated by experimental data and theoret-ical calculations has promoted the development of machine learning (ML) techniques for novel heteroge-neous catalyst development. ML is an effective tool in automating the generation, processing, and interpretation of large catalyst datasets with superior properties than the conventional statistical approaches. Also, ML have enabled the identification of accurate data-driven models that have been used to establish key relationships between the features of materials and targeted catalytic performance, such as activity, selectivity, and stability. These advances have resulted in the development of efficient design or screening guidelines for solid-state catalysts with targeted properties. However, extending the existing ML approaches to obtain accurate predictions of catalyst performance or design strategies for high -performance catalysts still poses several challenges. In this review, we discuss the recent milestones on the application of ML for solid heterogeneous catalysis and present the limitations and challenges of ML in this field. We also discuss potential future directions for the effective use of ML in solid hetero-geneous catalyst design. (c) 2021 Elsevier Ltd. All rights reserved.
更多查看译文
关键词
Machine learning,Heterogeneous catalysis,Catalyst database,Catalysis descriptors
AI 理解论文
溯源树
样例
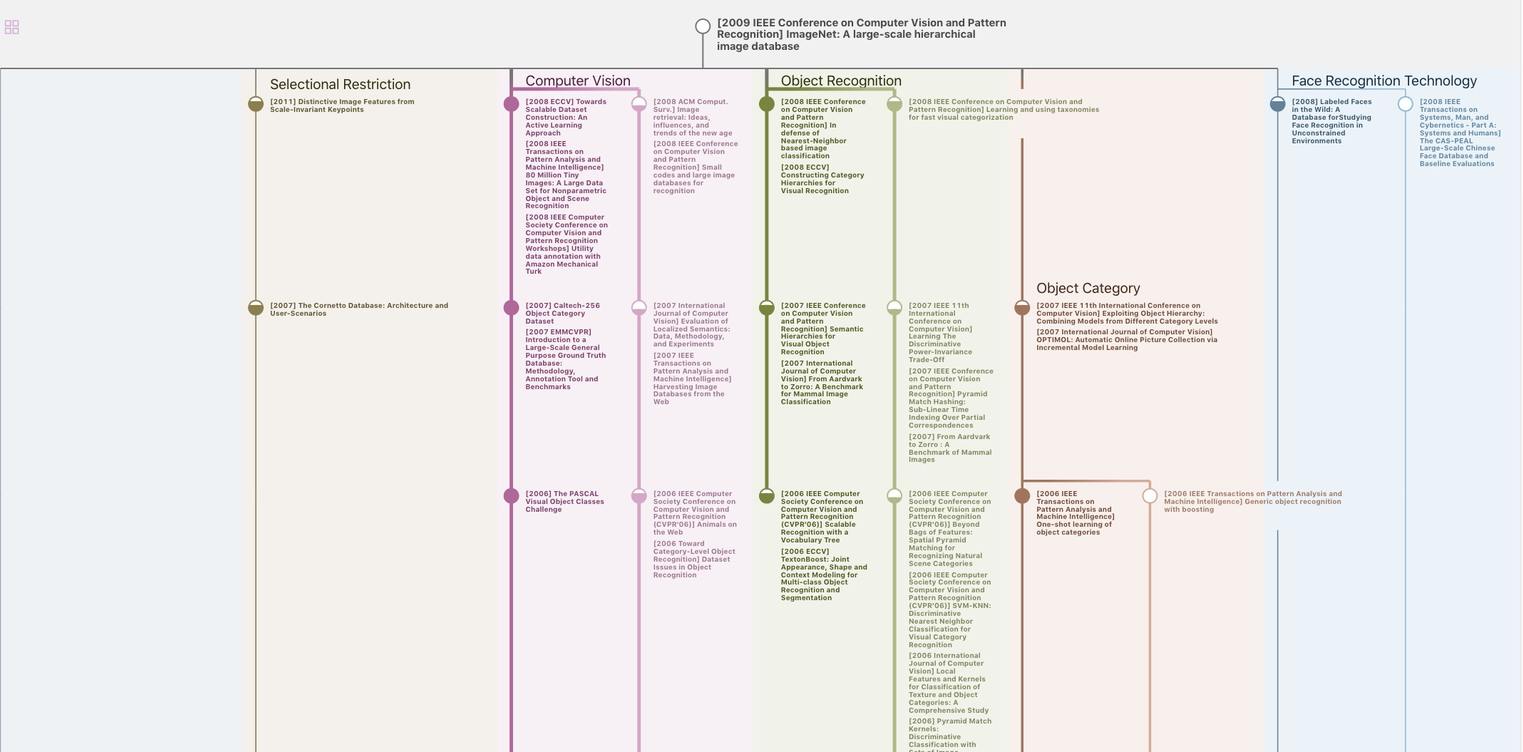
生成溯源树,研究论文发展脉络
Chat Paper
正在生成论文摘要