Surface-Enhanced Raman Scattering Spectroscopy Combined With Chemical Imaging Analysis for Detecting Apple Valsa Canker at an Early Stage
FRONTIERS IN PLANT SCIENCE(2022)
摘要
Apple Valsa canker (AVC) with early incubation characteristics is a severe apple tree disease, resulting in significant orchards yield loss. Early detection of the infected trees is critical to prevent the disease from rapidly developing. Surface-enhanced Raman Scattering (SERS) spectroscopy with simplifies detection procedures and improves detection efficiency is a potential method for AVC detection. In this study, AVC early infected detection was proposed by combining SERS spectroscopy with the chemometrics methods and machine learning algorithms, and chemical distribution imaging was successfully applied to the analysis of disease dynamics. Results showed that the samples of healthy, early disease, and late disease sample datasets demonstrated significant clustering effects. The adaptive iterative reweighted penalized least squares (air-PLS) algorithm was used as the best baseline correction method to eliminate the interference of baseline shifts. The BP-ANN, ELM, Random Forest, and LS-SVM machine learning algorithms incorporating optimal spectral variables were utilized to establish discriminative models to detect of the AVC disease stage. The accuracy of these models was above 90%. SERS chemical imaging results showed that cellulose and lignin were significantly reduced at the phloem disease-health junction under AVC stress. These results suggested that SERS spectroscopy combined with chemical imaging analysis for early detection of the AVC disease was feasible and promising. This study provided a practical method for the rapidly diagnosing of apple orchard diseases.
更多查看译文
关键词
apple Valsa canker,early detection,Surface-Enhanced Raman Scattering,chemical imaging,machine learning
AI 理解论文
溯源树
样例
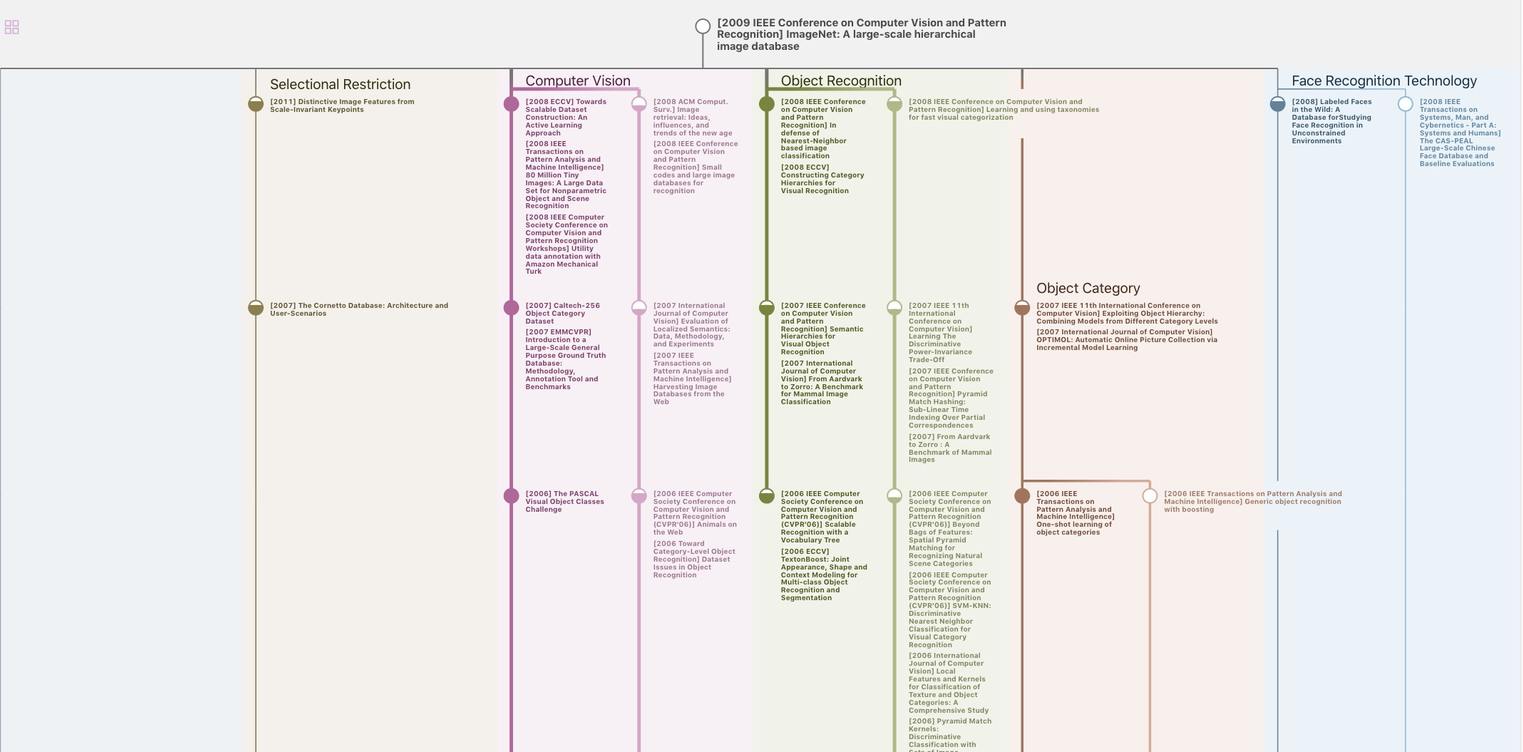
生成溯源树,研究论文发展脉络
Chat Paper
正在生成论文摘要