Security Analysis of Poisoning Attacks Against Multi-agent Reinforcement Learning
ALGORITHMS AND ARCHITECTURES FOR PARALLEL PROCESSING, ICA3PP 2021, PT I(2022)
摘要
As the closest machine learning method to general artificial intelligence, multi-agent reinforcement learning (MARL) has shown great potential. However, there are few security studies on MARL, and related security problems also appear, especially the serious misleading caused by the poisoning attack on the model. The current research on poisoning attacks for reinforcement learning mainly focuses on single-agent setting, while there are few such studies for multiagent RL. Hence, we propose an analysis framework for the poisoning attack in the MARL system, taking the multi-agent soft actor-critic algorithm, which has the best performance at present, as the target of the poisoning attack. In the framework, we conduct extensive poisoning attacks on the agent's state signal and reward signal from three different aspects: the modes of poisoning attacks, the impact of the timing of poisoning, and the mitigation ability of the MARL system. Experiment results in our framework indicate that 1) compared to the baseline, the random poisoning against state signal reduces the average reward by as high as -65.73%; 2) the timing of poisoning has completely opposite effects on reward-based and state-based attacks; and 3) the agent can completely alleviate the toxicity when the attack interval is 10000 episodes.
更多查看译文
关键词
Reinforcement learning, Multi-agent system, Soft actor-critic, Poisoning attack, Security analysis
AI 理解论文
溯源树
样例
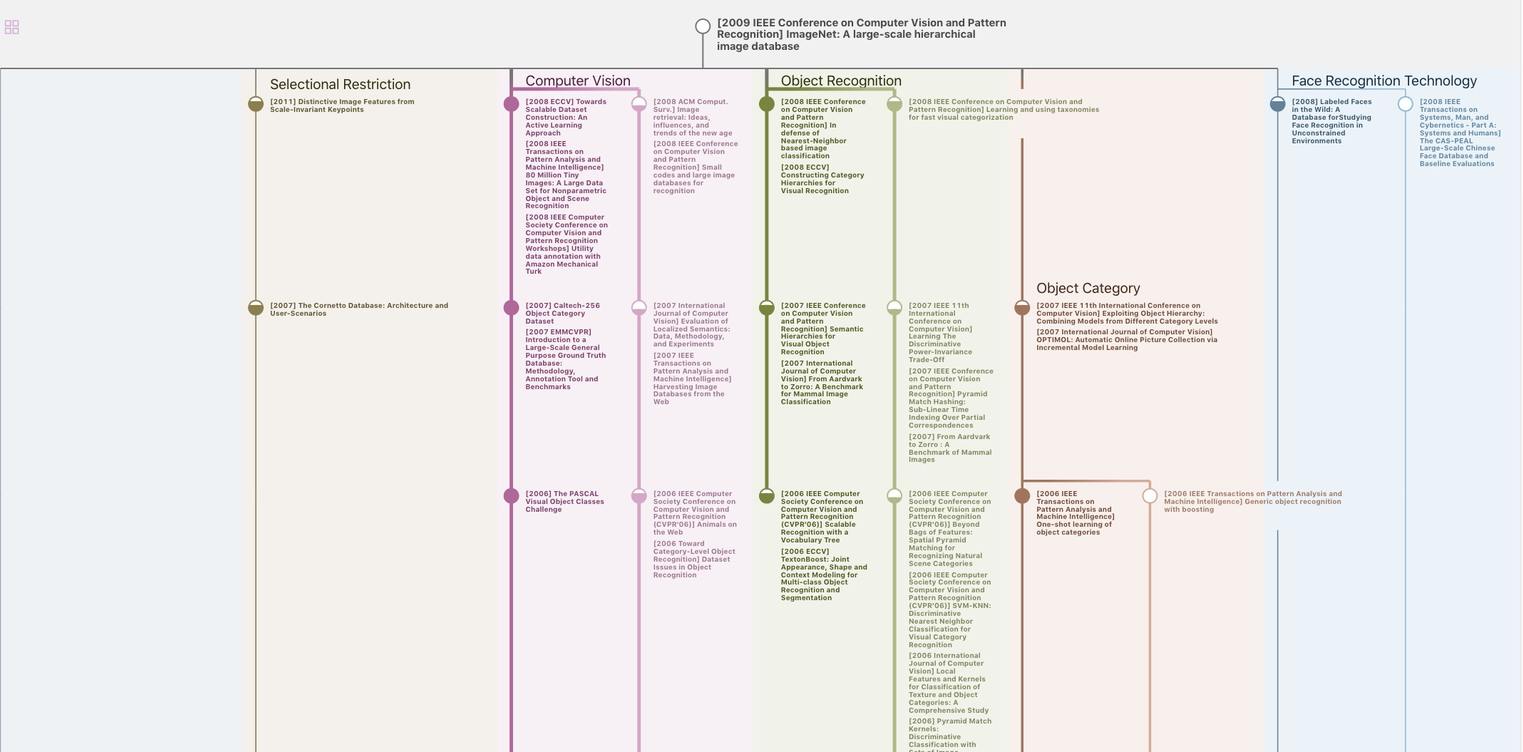
生成溯源树,研究论文发展脉络
Chat Paper
正在生成论文摘要