Uncertainty propagation method for high-dimensional black-box problems via Bayesian deep neural network
STRUCTURAL AND MULTIDISCIPLINARY OPTIMIZATION(2022)
摘要
A high-dimensional uncertainty propagation (UP) method is proposed in this paper, solving UP problems directly in the high-dimensional space. Firstly, a probability density function-based sampling (PDFS) method is developed to generate input samples, which can locate the area determined by the spatial distribution characteristics of input variables efficiently. High-quality training data can thus be obtained by computing the system response of objective black-box problem at those input samples. Secondly, Bayesian deep neural network (BDNN) is trained on the training data to construct surrogate model for objective black-box problem. Thirdly, Monte Carlo sampling is implemented on the trained BDNN to compute the statistical samples of system response. Finally, Gaussian mixture model is utilized to fit the probability density function (PDF) of system response based on the statistical samples. Moreover, because PDFS can generate samples according to the PDF of input variables, it is also suitable for problems involving multimodal distributions. Several numerical examples are utilized to validate the effectiveness of proposed method.
更多查看译文
关键词
High-dimensional uncertainty propagation, Bayesian deep neural network, Probability density function-based sampling, Multimodal distribution, Black-box problems
AI 理解论文
溯源树
样例
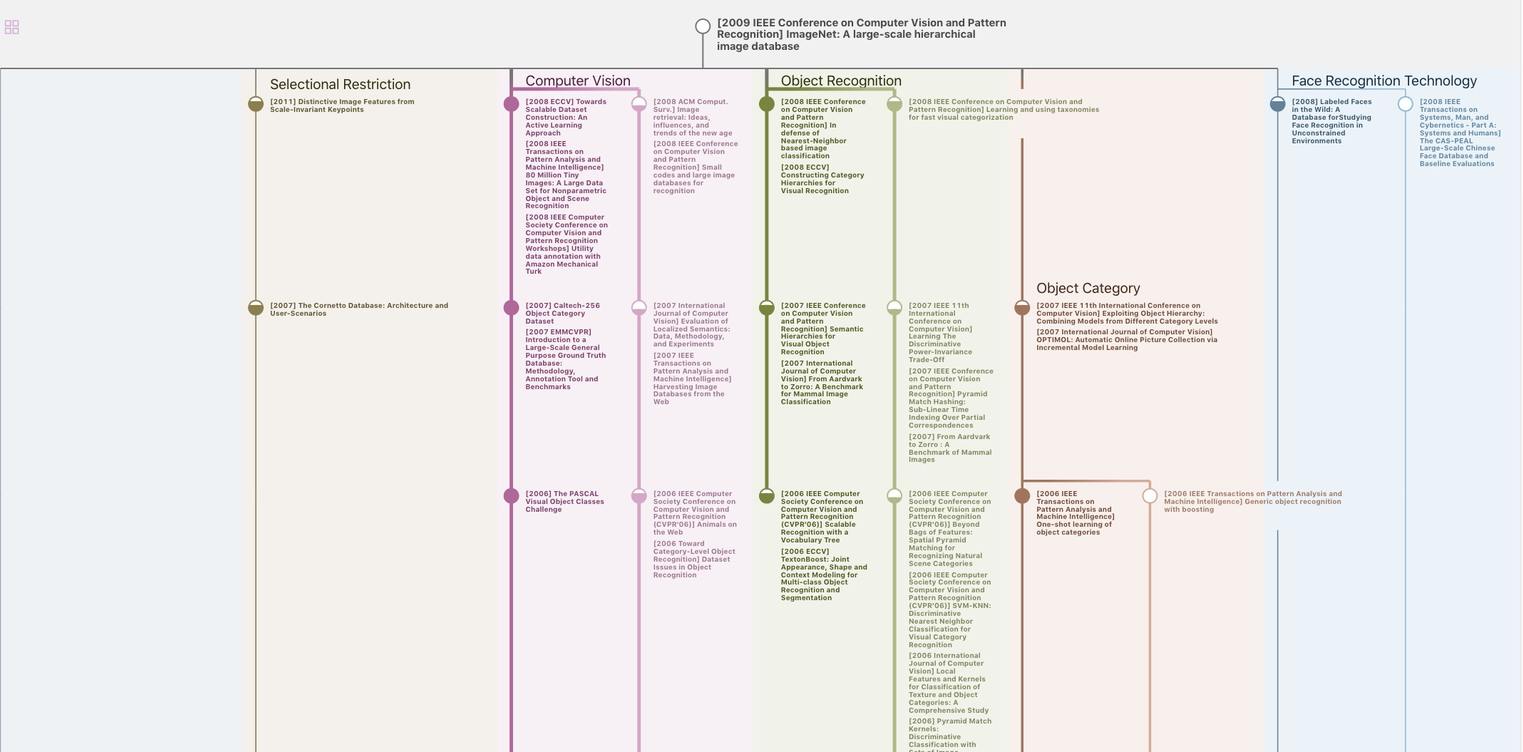
生成溯源树,研究论文发展脉络
Chat Paper
正在生成论文摘要