DLDFD: Recurrence Free 2D Convolution Approach for Deep Fake Detection
PROCEEDINGS OF THE 17TH INTERNATIONAL JOINT CONFERENCE ON COMPUTER VISION, IMAGING AND COMPUTER GRAPHICS THEORY AND APPLICATIONS (VISAPP), VOL 4(2022)
摘要
Deep Fake images, which are digitally generated either through computer graphics or deep learning techniques, pose an increasing risk to existing face recognition systems. This paper presents a Deep-Learning-based Deep Fake Detection (DLDFD) architecture consisting of augmented convolutional layers followed by Resnet-50 architecture. We train DLDFD end-to-end with low-resolution images from the FaceForensics++ dataset. The number of images used during different phases includes approximately 1.68 million during training, 315k during validation, and 340k during testing. We train DLDFD in three different scenarios, combined image manipulation where we achieve an accuracy of 96.07% compared to 85.14% of state-of-the-art (SOTA), single image manipulation techniques where we get 100% accuracy for neural textures, and finally, cross-image manipulation techniques where we achieve an accuracy of 94.28% on the unseen category of face swap much higher than SOTA. Our approach requires only 2D convolutions without recurrence as compared to SOTA.
更多查看译文
关键词
DeepFakes Detection, Resnet, Deep Learning Architecture
AI 理解论文
溯源树
样例
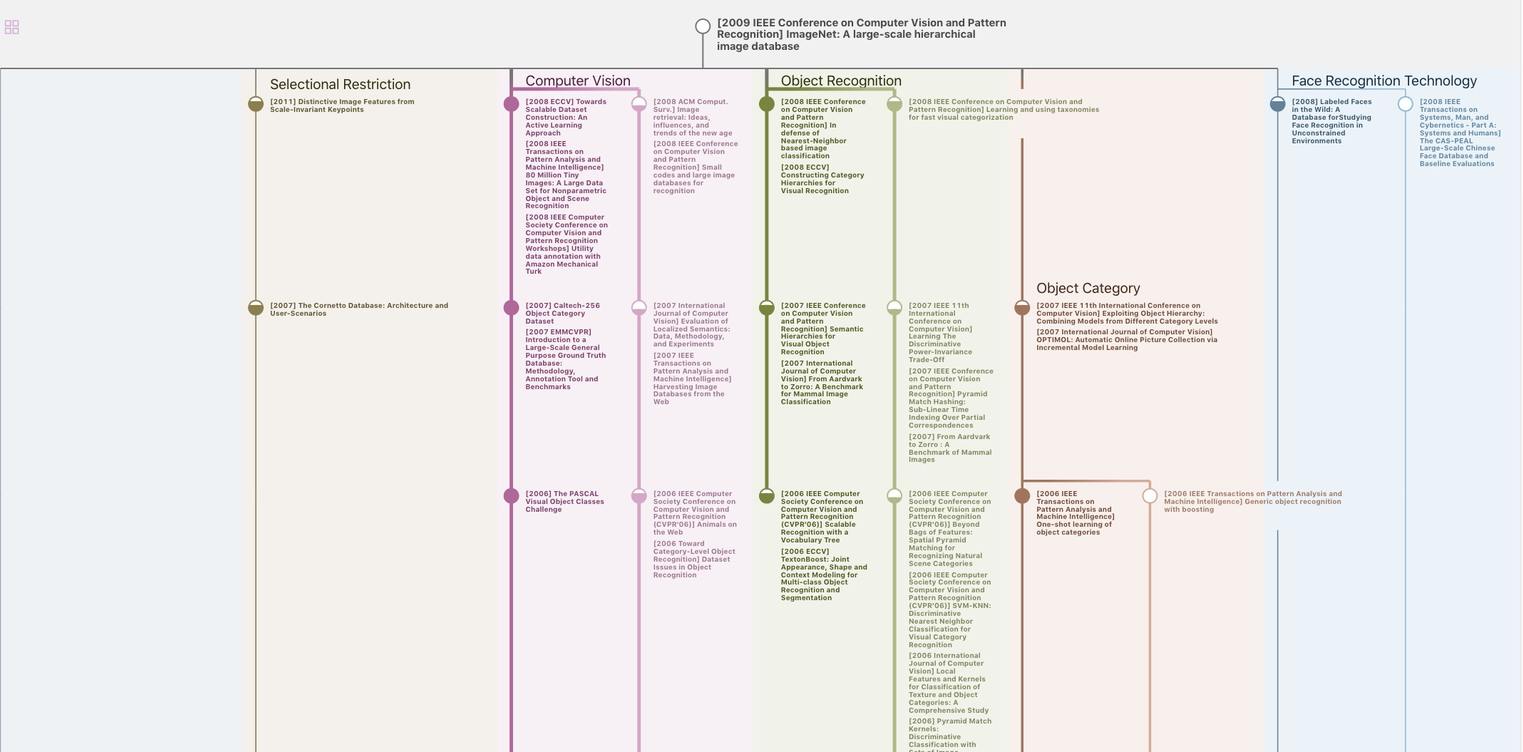
生成溯源树,研究论文发展脉络
Chat Paper
正在生成论文摘要