Deep Depth Completion of Low-cost Sensor Indoor RGB-D using Euclidean Distance-based Weighted Loss and Edge-aware Refinement
PROCEEDINGS OF THE 17TH INTERNATIONAL JOINT CONFERENCE ON COMPUTER VISION, IMAGING AND COMPUTER GRAPHICS THEORY AND APPLICATIONS (VISAPP), VOL 4(2022)
摘要
Low-cost depth-sensing devices can provide real-time depth maps to many applications, such as robotics and augmented reality. However, due to physical limitations in the acquisition process, the depth map obtained can present missing areas corresponding to irregular, transparent, or reflective surfaces. Therefore, when there is more computing power than just the embedded processor in low-cost depth sensors, models developed to complete depth maps can boost the system's performance. To exploit the generalization capability of deep learning models, we propose a method composed of a U-Net followed by a refinement module to complete depth maps provided by Microsoft Kinect. We applied the Euclidean distance transform in the loss function to increase the influence of missing pixels when adjusting our network filters and reduce blur in predictions. We outperform state-of-the-art methods for completed depth maps in a benchmark dataset. Our novel loss function combining the distance transform, gradient and structural similarity measure presents promising results in guiding the model to reduce unnecessary blurring of final depth maps predicted by a convolutional network.
更多查看译文
关键词
Deep Learning, Depth Completion, RGB plus Depth, Depth Sensing, Distance Transforms
AI 理解论文
溯源树
样例
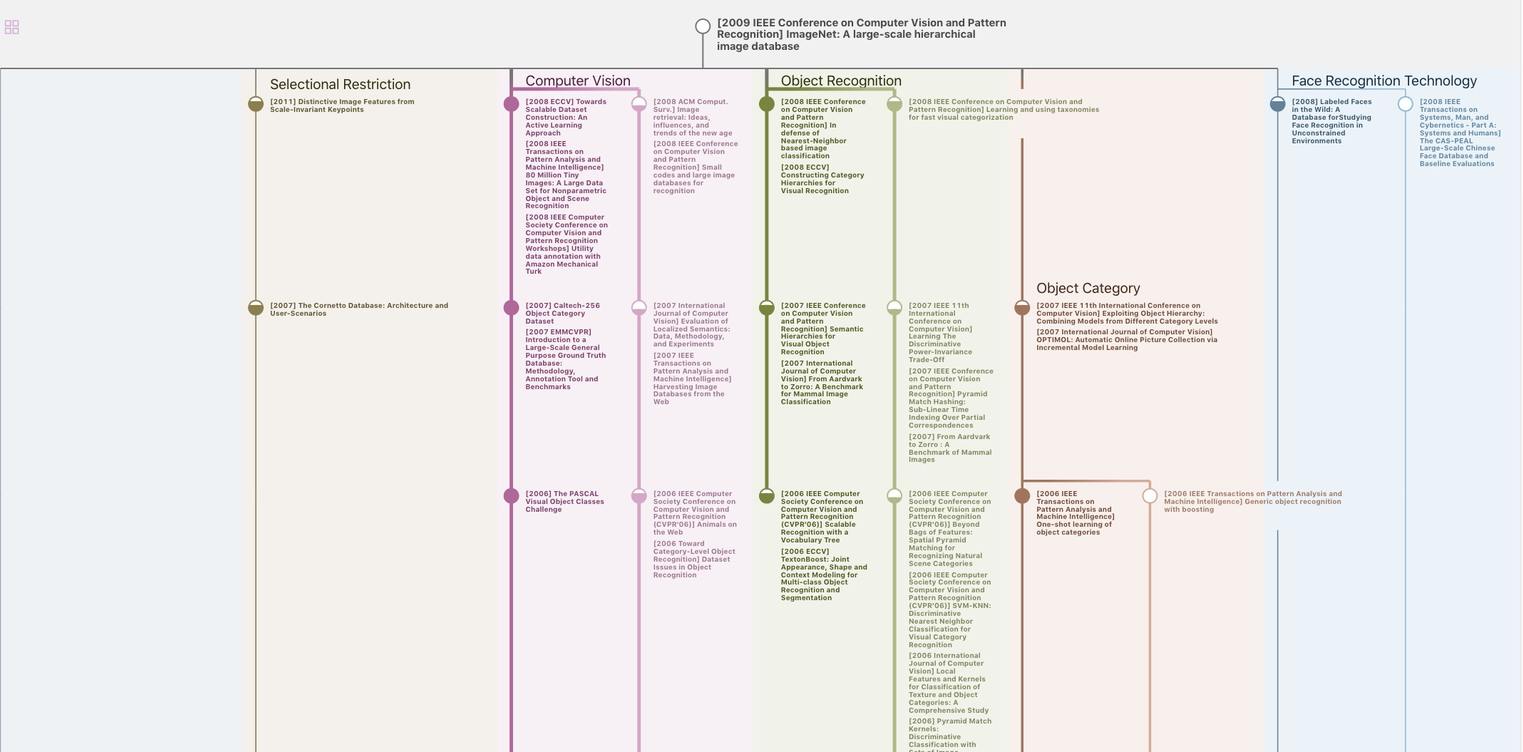
生成溯源树,研究论文发展脉络
Chat Paper
正在生成论文摘要