Threshold Selection in Feature Screening for Error Rate Control
JOURNAL OF THE AMERICAN STATISTICAL ASSOCIATION(2022)
摘要
Hard thresholding rule is commonly adopted in feature screening procedures to screen out unimportant predictors for ultrahigh-dimensional data. However, different thresholds are required to adapt to different contexts of screening problems and an appropriate thresholding magnitude usually varies from the model and error distribution. With an ad-hoc choice, it is unclear whether all of the important predictors are selected or not, and it is very likely that the procedures would include many unimportant features. We introduce a data-adaptive threshold selection procedure with error rate control, which is applicable to most kinds of popular screening methods. The key idea is to apply the sample-splitting strategy to construct a series of statistics with marginal symmetry property and then to utilize the symmetry for obtaining an approximation to the number of false discoveries. We show that the proposed method is able to asymptotically control the false discovery rate and per family error rate under certain conditions and still retains all of the important predictors. Three important examples are presented to illustrate the merits of the new proposed procedures. Numerical experiments indicate that the proposed methodology works well for many existing screening methods. Supplementary materials for this article are available online.
更多查看译文
关键词
Empirical distribution, False discovery rate, Feature screening, Per family error rate, Symmetry
AI 理解论文
溯源树
样例
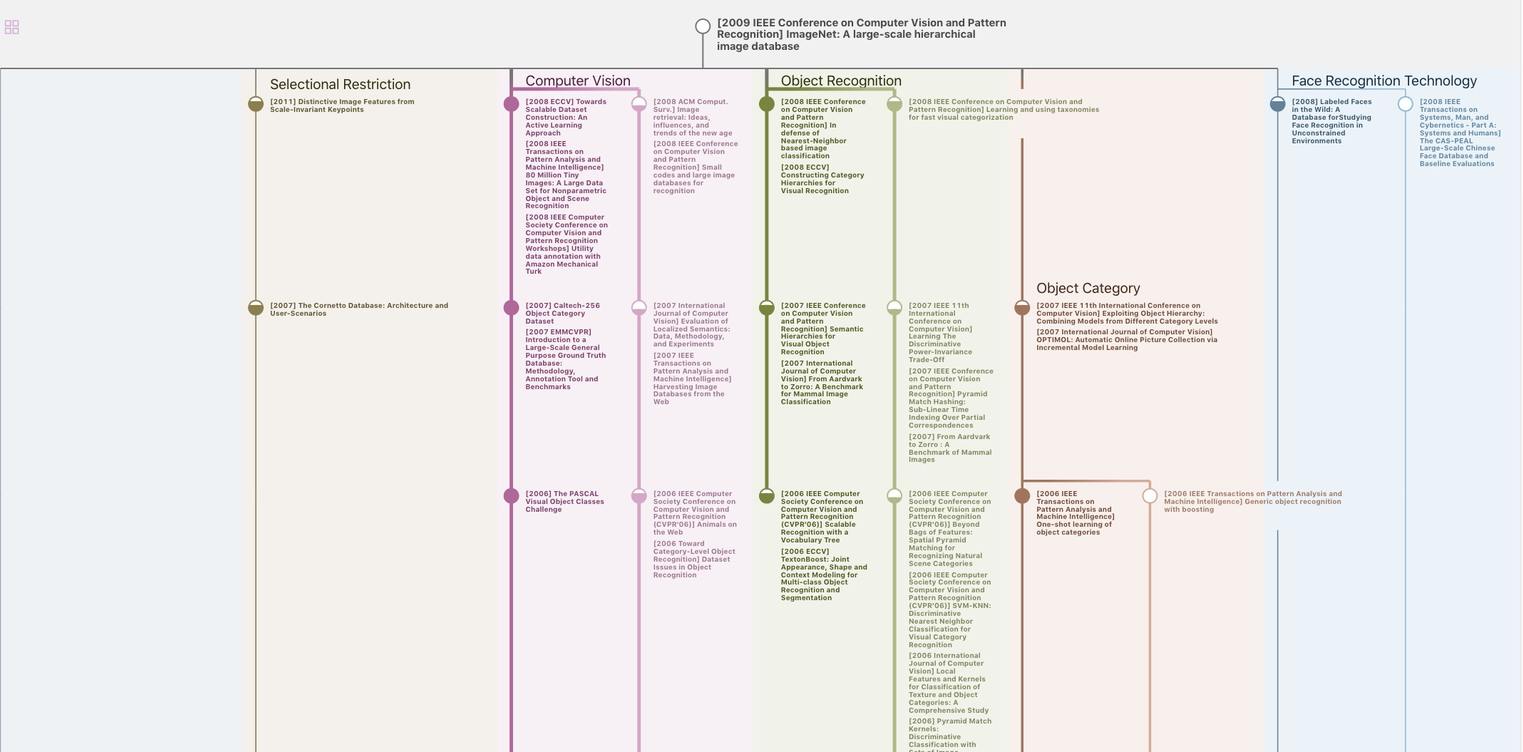
生成溯源树,研究论文发展脉络
Chat Paper
正在生成论文摘要