A robust clustering algorithm based on the identification of core points and KNN kernel density estimation
Expert Systems with Applications(2022)
摘要
Density peaks clustering (DPC) has been proved to be an effective clustering method and applied to many scientific fields. It can detect the density peak within each cluster and assign the rest of the points in a single step. However, the original method suffers from several drawbacks. First of all, the authors provided two density metrics for the density estimation of large and small datasets. However, the arbitrary choice of the density metric will influence the effectiveness of DPC. Besides, the identification of cluster centers requires assigning thresholds manually for two extra quantities, which lowers the robustness of DPC. Moreover, the stepwise procedure of DPC in assigning the remaining points after cluster centers have been found may cause the propagation of errors. To eliminate these drawbacks, we proposed a new KNN based clustering method named ICKDC. The new framework aims at representing a cluster by a set of core points instead of a single object for the center and classifying the rest of the objects effectively based on the distribution information. Generally, the process of KNN based local density estimation, core points identification and integration, and the classification of the non-core objects allow ICKDC to reveal the structure of clusters. The experiments on various synthetic and real-world datasets demonstrate the superiority of ICKDC over several widely used clustering algorithms and state-of-the-art DPC-variants.
更多查看译文
关键词
Clustering,Density peaks,Core points,Density estimation,KNN kernel
AI 理解论文
溯源树
样例
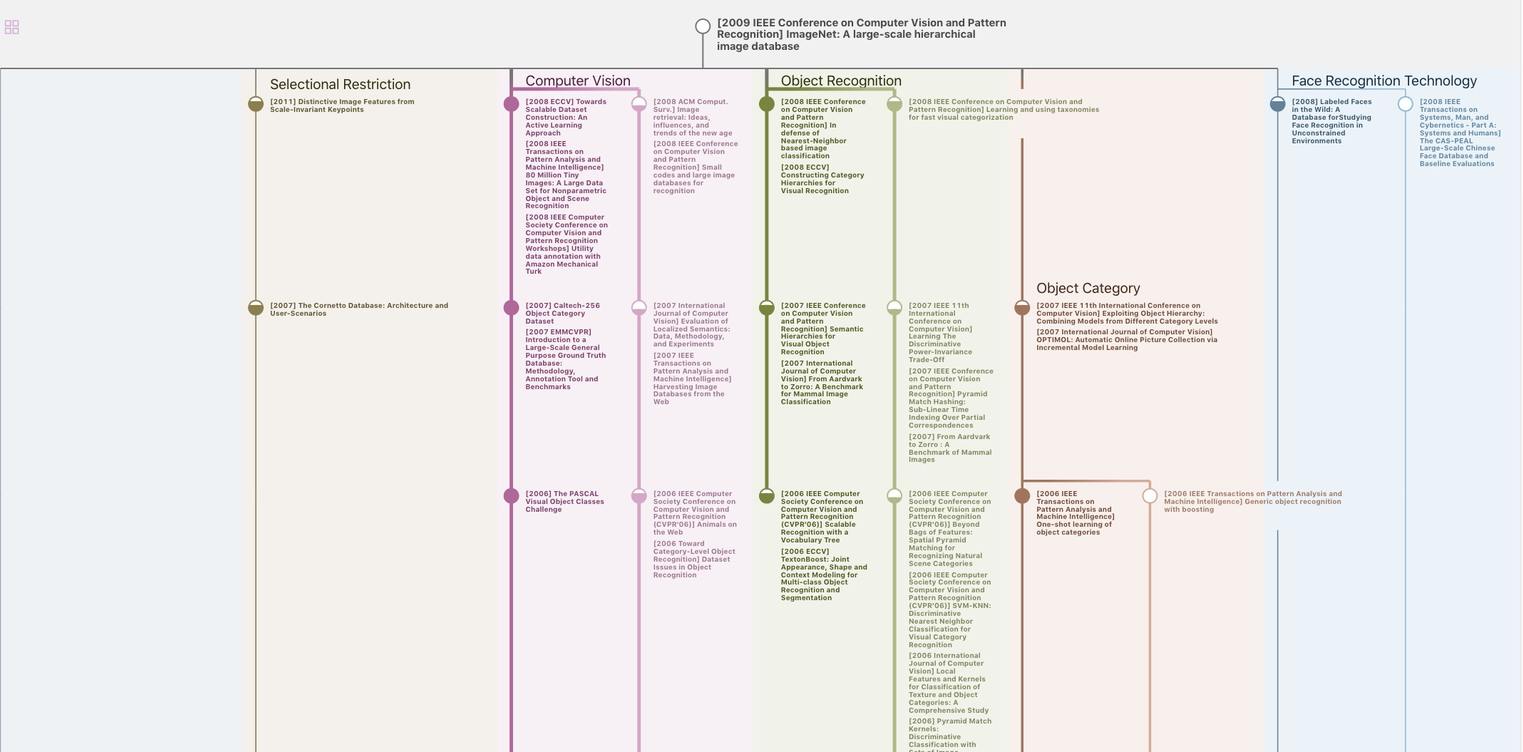
生成溯源树,研究论文发展脉络
Chat Paper
正在生成论文摘要