A novel constrained non-negative matrix factorization method based on users and items pairwise relationship for recommender systems
EXPERT SYSTEMS WITH APPLICATIONS(2022)
摘要
Non-negative matrix factorization (NMF) is a famous method to learn parts-based representations of non-negative data. It has been used successfully in various applications such as information retrieval and recommender systems. Most of the current NMF methods only focus on how each decomposed matrices vector should be modeled and disregard the relation among pairwise feature vectors of users and items. In recommender systems, the relationship between users’ factors or items’ features provides better factorization. This paper proposes a constrained non-negative matrix factorization for recommender systems (CNMF-RS), which puts constraints on pairwise vectors of users and items by applying penalties using similarity. Two cost functions are proposed to generate the corresponding updating rules for finding unknown ratings. The computational complexity analysis confirmed that CNMF-RS does not raise time complexity using constraints compared with the basic NMF. Experiments on benchmark datasets indicate that the suggested update rules converge quickly and obtain outstanding results compared to other methods.
更多查看译文
关键词
Recommender systems,Non-negative matrix factorization,Multiplicative updating rules,Latent factors
AI 理解论文
溯源树
样例
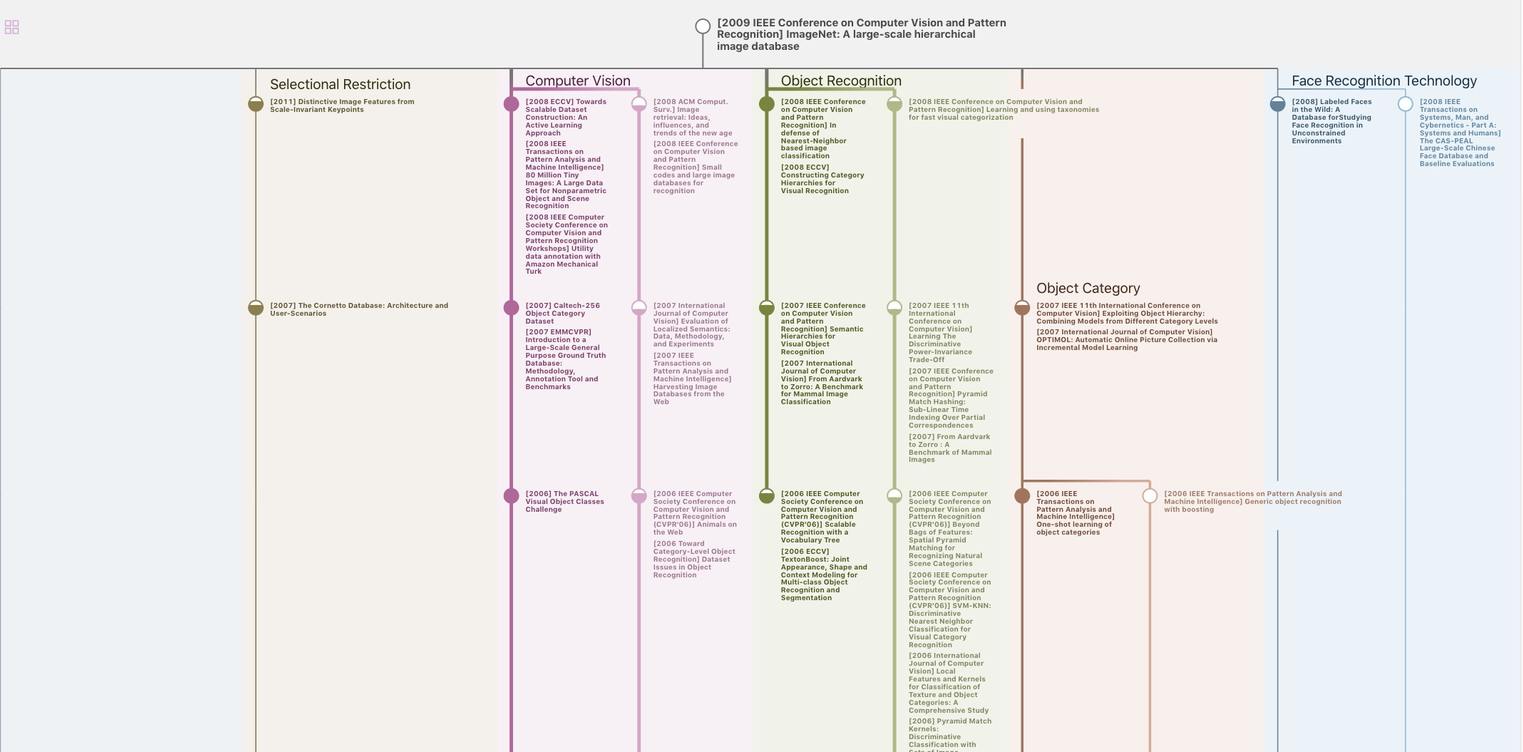
生成溯源树,研究论文发展脉络
Chat Paper
正在生成论文摘要