A Deep Reinforcement Learning Approach with Visual Semantic Navigation with Memory for Mobile Robots in Indoor Home Context
JOURNAL OF INTELLIGENT & ROBOTIC SYSTEMS(2022)
摘要
Navigate is a crucial task to be performed by social robot agents in complex and uncertain environments like homes and offices. Search for specific target objects is usually a required activity. This work aimed to propose a visual semantic navigation with memory architecture model. Based on recent advances in convolutional neural networks and graph neural networks, a visual semantic navigation architecture model (GCN-MLP) was extended with recurrent neural networks for memory mechanisms (GCN-GRU and GCN-LSTM) while exposing a robot agent in navigation experiences to learn navigation policies. The models were evaluated quantitatively and qualitatively, where memory enhanced models demonstrated early convergence, better performance in evaluation metrics, increased successfully terminated episodes, more efficient path trajectories, and lower decrease in performance when exposed to challenger test scheme, presenting a more exploratory behavior. Finally, were analyzed differences between GRU and LSTM, where GRU performed similarly to LSTM in some cases, being a viable option.
更多查看译文
关键词
Deep reinforcement learning,Recurrent neural networks,Graph neural networks,Mobile robots,Learning-based navigation
AI 理解论文
溯源树
样例
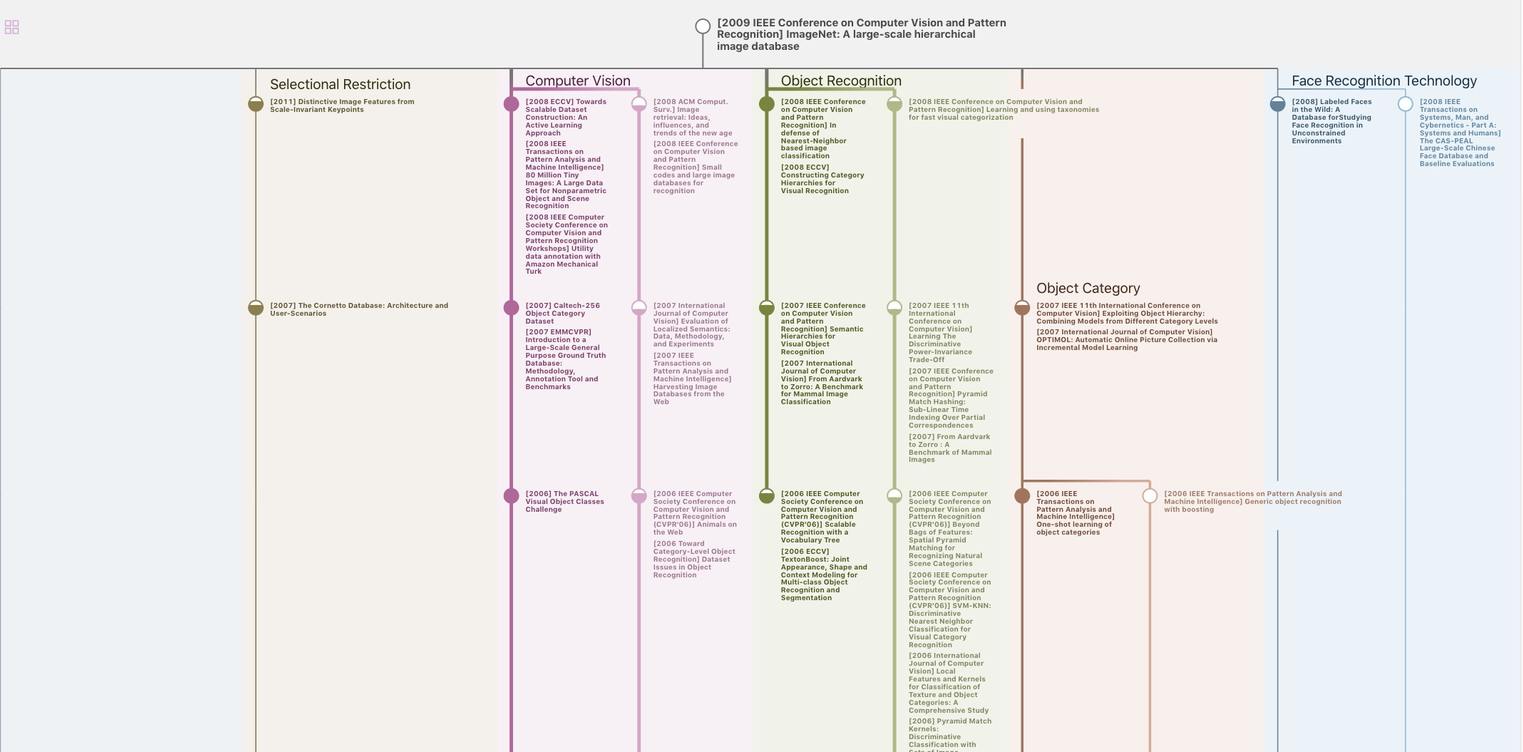
生成溯源树,研究论文发展脉络
Chat Paper
正在生成论文摘要