Rational design of high power density "Blue Energy Harvester" pressure retarded osmosis (PRO) membranes using artificial intelligence-based modeling and optimization
ENERGY CONVERSION AND MANAGEMENT(2022)
摘要
The challenge in harvesting Salinity Gradient Power (SGP) through pressure retarded osmosis (PRO) requires design of high power density (PD) membranes and optimized process for operation. Recent studies show that for a feasible PRO operation the minimum net PD should be around 50 W/m2. In this study, a data-driven approach has been adopted for designing optimum membranes as well as operating conditions. 200 papers, from last decade, were extensively reviewed and 34 experimental research articles were shortlisted for possible data mining, to predict water flux (WF) and PD. Comprehensive screened/pre-processed data related to both membrane and process (16 inputs) was obtained from 18 articles amounting to 339 data points. Two artificial neural network (ANN) models were explored (i) Levenberg-Marquardt (ANN-LM), and (ii) Bayesian Regularization (ANN-BR) along with a combination of three different activation functions i.e., hyperbolic tangent sigmoid transfer function (Tan-Sigmoid), logarithmic sigmoid transfer function (Log-Sigmoid) in the input and output layers. Out of the six resulting combinations, the best performing combination was found to be Tan-Sigmoid activation function in both layers with ANN-BR model having an R2 value of 0.97 for WF and 0.98 for PD. Membrane properties like the type of membrane, thickness, and water permeability coefficient were found to be the major contributing factors for the prediction of WF while for PD, operating conditions such as applied pressure were found to play the major contributing factor (10-16 %). Optimization results yield a maximum WF of 147 LMH and PD of 87 W/m2. These results were compared with the solution diffusion (S-D) model.
更多查看译文
关键词
Pressure retarded osmosis, Salinity gradient power, High power density membrane, Artificial intelligence, Machine learning, Inverse design
AI 理解论文
溯源树
样例
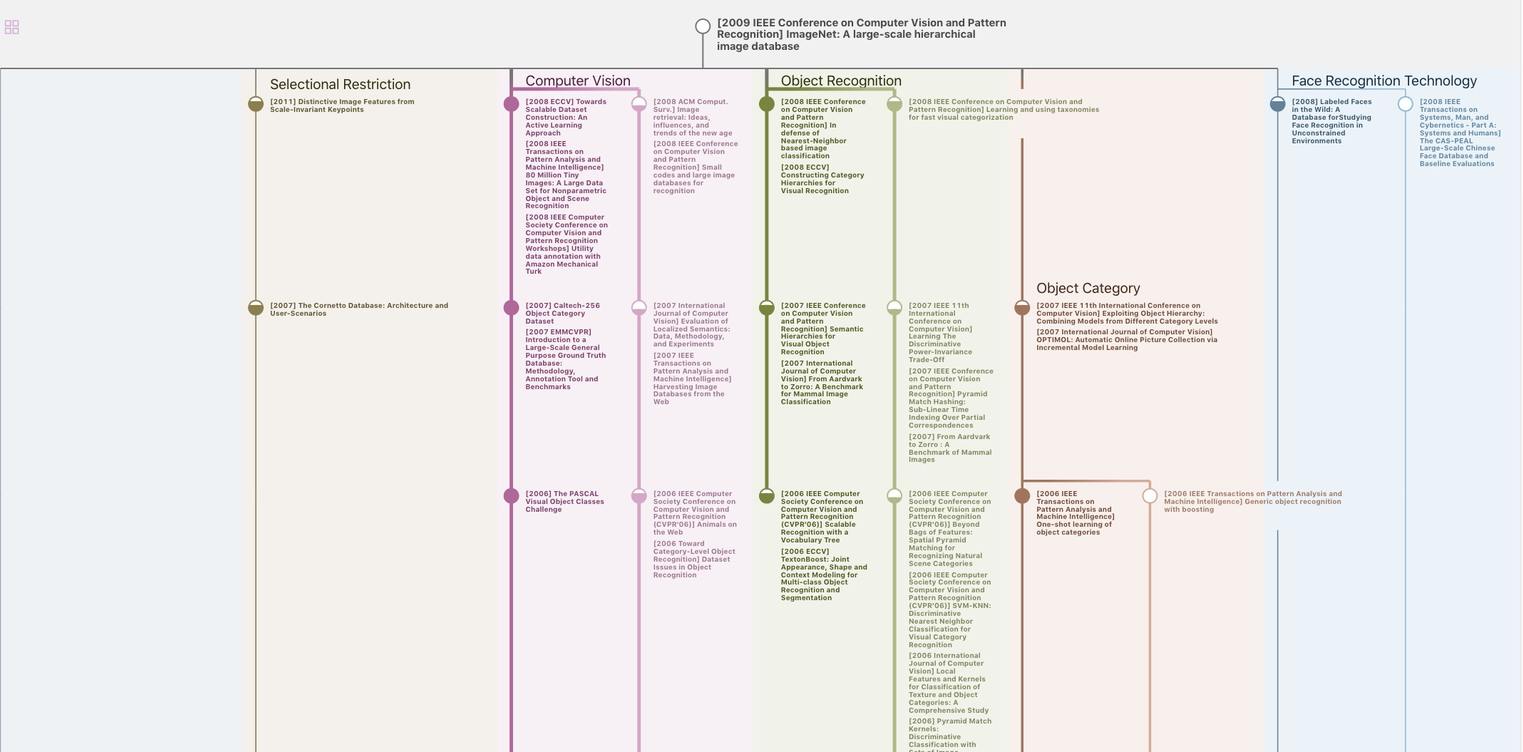
生成溯源树,研究论文发展脉络
Chat Paper
正在生成论文摘要