A 64-channel scheme for autism detection via scaled conjugate gradient-based neural network classification of electroencephalogram ripples' complexity
EXPERT SYSTEMS(2023)
摘要
The description of autism spectrum disorder (ASD) includes typically the alterations in social skills and attitude as well as problems of communication. At present, the most promising approaches suggested for ASD automatic diagnosis are the machine learning (ML)-based procedures that begin by the identification and extraction of the most discriminant features. One of the proposed discriminant features of ASD is electroencephalogram (EEG) entropy that reflects the level of complexity or randomness of brain dynamics. The two main techniques currently used to describe the entropy in ASD are multi-scale entropy (MSE) and spectral entropy (SE). However, MSE is unable to track the exact frequency content on the different time scales that suffer-themselves-from mismatching. In addition, the outcomes of SE approaches in literature are contradictory and confusing about the altered band, direction of change as well as about the affected brain region. The common point in those SE approaches is the examination of one or more of the well-known frequency bands: Delta, Theta, Alpha, Beta and low Gamma. Hence, the range of high Gamma ripples (>80 Hz) is barely examined in ASD literature due to the needed high carefulness in its technical acquisition, detection and processing as well as to the common acceptance of the idea that it is rather related to pathological seizure activity; which is a misconception recently refuted. Further, the study of automatic ASD diagnosis based on ripples is almost inexistent in literature. The present work suggests an accurate automatic technique to classify ASD and neuro-typical EEG entropy based on the whole range of ripples (75-250 Hz): (1) EEG signals are collected (120 ASD and neuro-typical children) by an efficient 64-channel system highly suitable in noisy environment with movement artefacts. (2) All signal disturbances are automatically removed. (3) The power spectra of all EEG channels are computed. (4) Shannon entropy values are calculated for the range of ripples without filtering. (5) Scaled Conjugate Gradient-based Neural Network (NN) classification, k-fold cross validation and T-test are applied to entropy values. (6) Statistical assessment is conducted. The best testing and the overall accuracies were found to be 98.9% and 95.0% respectively.
更多查看译文
关键词
autism, classification, EEG, entropy, ripples, SCG-NN
AI 理解论文
溯源树
样例
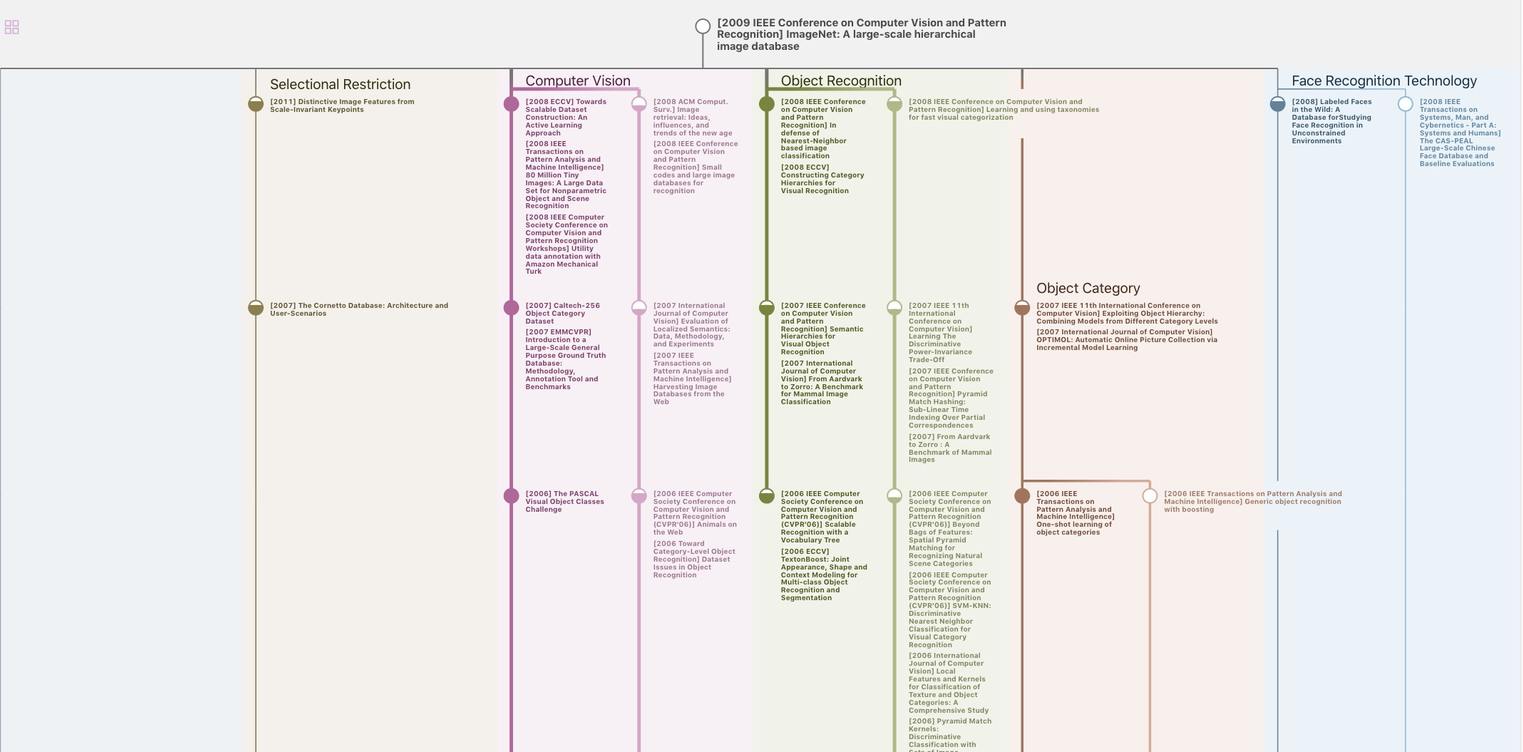
生成溯源树,研究论文发展脉络
Chat Paper
正在生成论文摘要