Planning of Commercial Thinnings Using Machine Learning and Airborne Lidar Data
FORESTS(2022)
摘要
The goal of this study was to predict the need for commercial thinning using airborne lidar data (ALS) with random forest (RF) machine learning algorithm. Two test sites (with areas of 14,750 km(2) and 12,630 km(2)) were used with a total of 1053 forest stands from southwestern Estonia and 951 forest stands from southeastern Estonia. The thinnings were predicted based on the ALS measurements in 2019 and 2017. The two most important ALS metrics for predicting the need for thinning were the 95th height percentile and the canopy cover. The prediction accuracy based on validation stands was 93.5% for southwestern Estonia and 85.7% for southeastern Estonia. For comparison, the general linear model prediction accuracy was less for both test sites-92.1% for southwest and 81.8% for southeast. The selected important predictive ALS metrics differed from those used in the RF algorithm. The cross-validation of the thinning necessity models of southeastern and southwestern Estonia showed a dependence on geographic regions.
更多查看译文
关键词
forest management planning, machine learning, airborne lidar, commercial thinning, sparse lidar point clouds
AI 理解论文
溯源树
样例
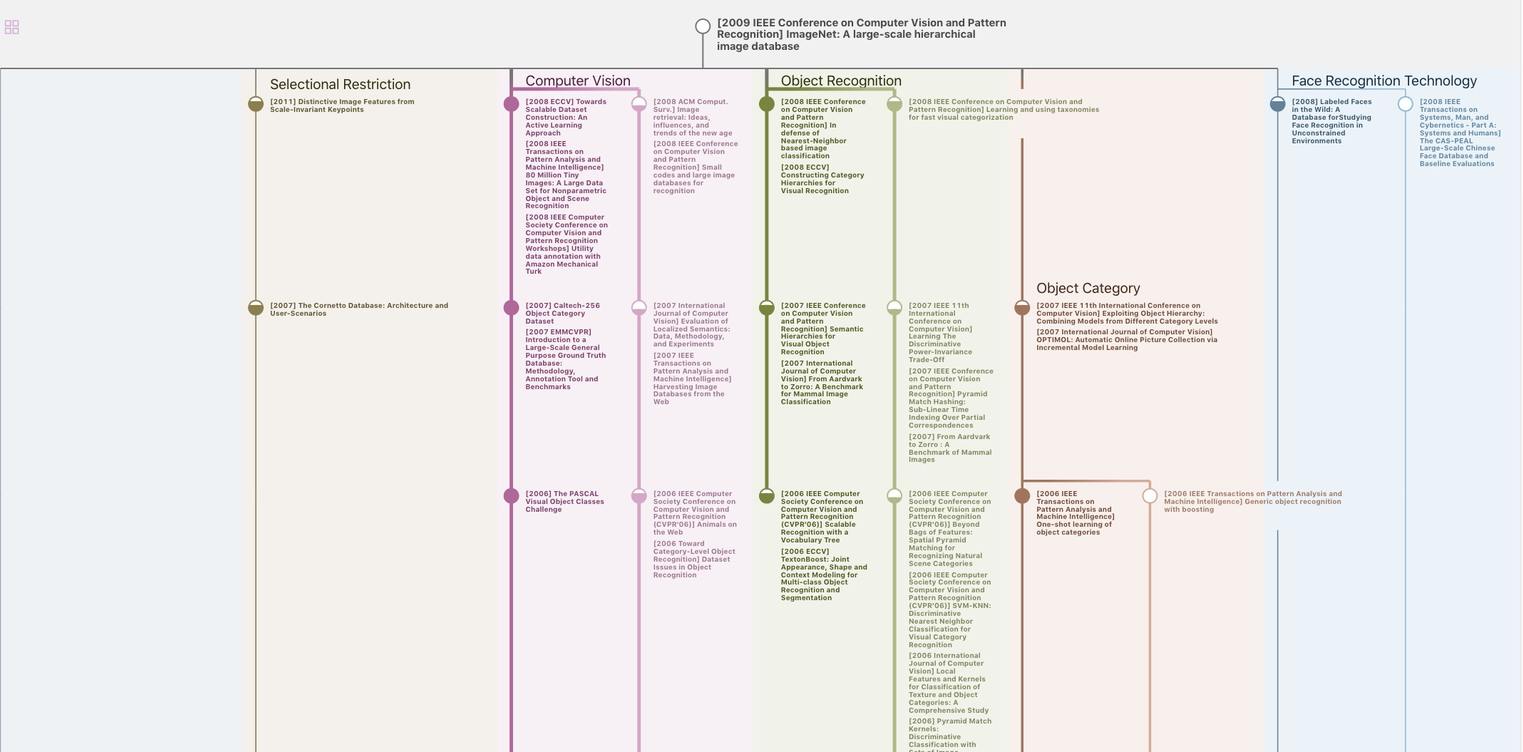
生成溯源树,研究论文发展脉络
Chat Paper
正在生成论文摘要