Action control, forward models and expected rewards: representations in reinforcement learning
SYNTHESE(2021)
摘要
The fundamental cognitive problem for active organisms is to decide what to do next in a changing environment. In this article, we analyze motor and action control in computational models that utilize reinforcement learning (RL) algorithms. In reinforcement learning, action control is governed by an action selection policy that maximizes the expected future reward in light of a predictive world model. In this paper we argue that RL provides a way to explicate the so-called action-oriented views of cognitive systems in representational terms.
更多查看译文
关键词
Representation, Reinforcement learning, Action control, Radical enactivism, Cognitive science
AI 理解论文
溯源树
样例
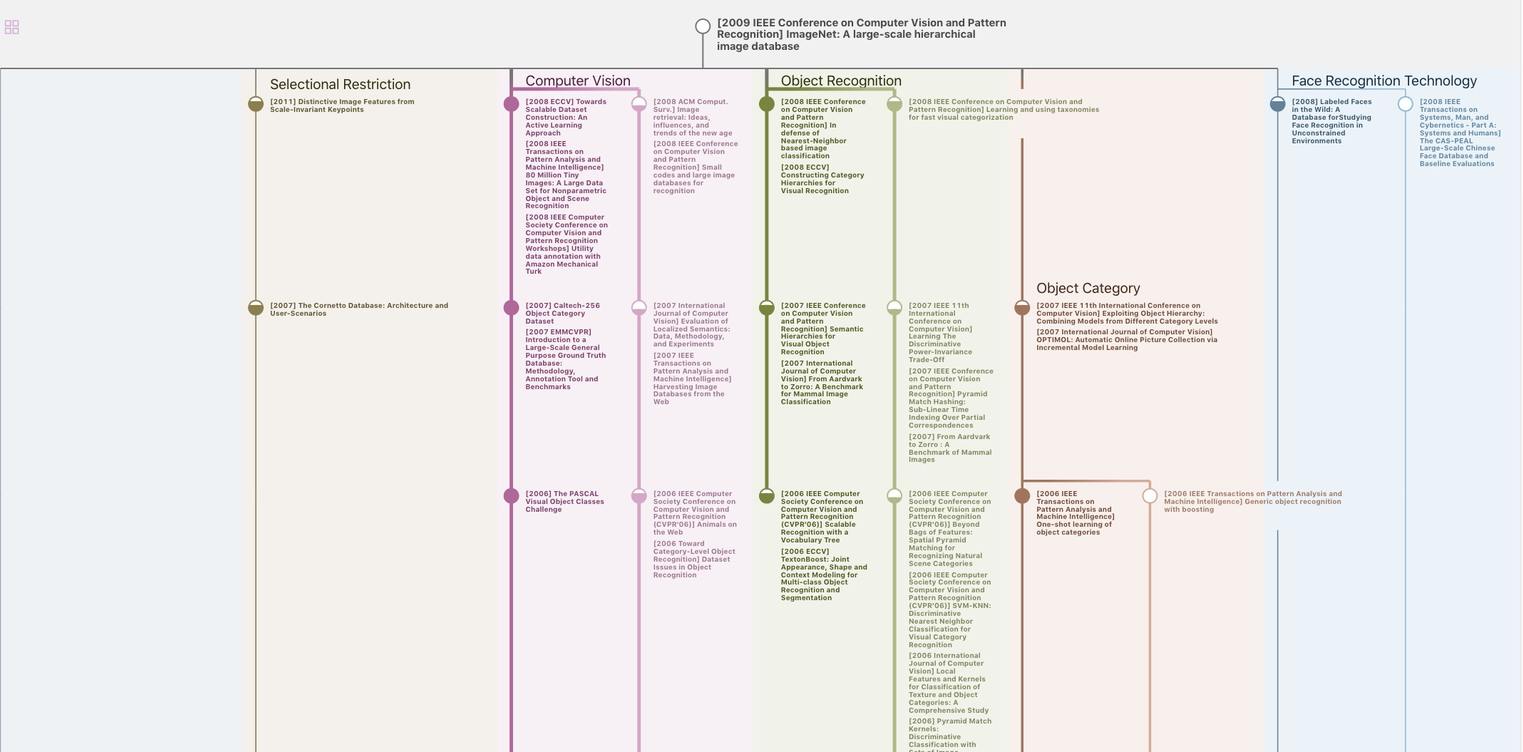
生成溯源树,研究论文发展脉络
Chat Paper
正在生成论文摘要