EDPANs: Enhanced Dual Path Attention Networks for Single Image Super-Resolution
JOURNAL OF CIRCUITS SYSTEMS AND COMPUTERS(2021)
摘要
In this paper, we propose an enhanced dual path attention network (EDPAN) for image superresolution. ResNet is good at implicitly reusing extracted features, DenseNet is good at exploring new features. Dual Path Network (DPN) combines ResNets and DenseNet to create a more accurate architecture than the straightforward one. We experimentally show that the residual network performs best when each block consists of two convolutions, and the dense network performs best when each micro-block consists of one convolution. Following these ideas, our EDPAN exploits the advantages of the residual structure and the dense structure. Besides, to deploy the computations for features more effectively, we introduce the attention mechanism into our EDPAN. Moreover, to relieve the parameters burden, we also utilize recursive learning to propose a lightweight model. In the experiments, we demonstrate the effectiveness and robustness of our proposed EDPAN on different degradation situations. The quantitative results and visualization comparison can sufficiently indicate that our EDPAN achieves favorable performance over the state-of-the-art frameworks.
更多查看译文
关键词
Image super-resolution, deep-learning, self-attention, dual path network
AI 理解论文
溯源树
样例
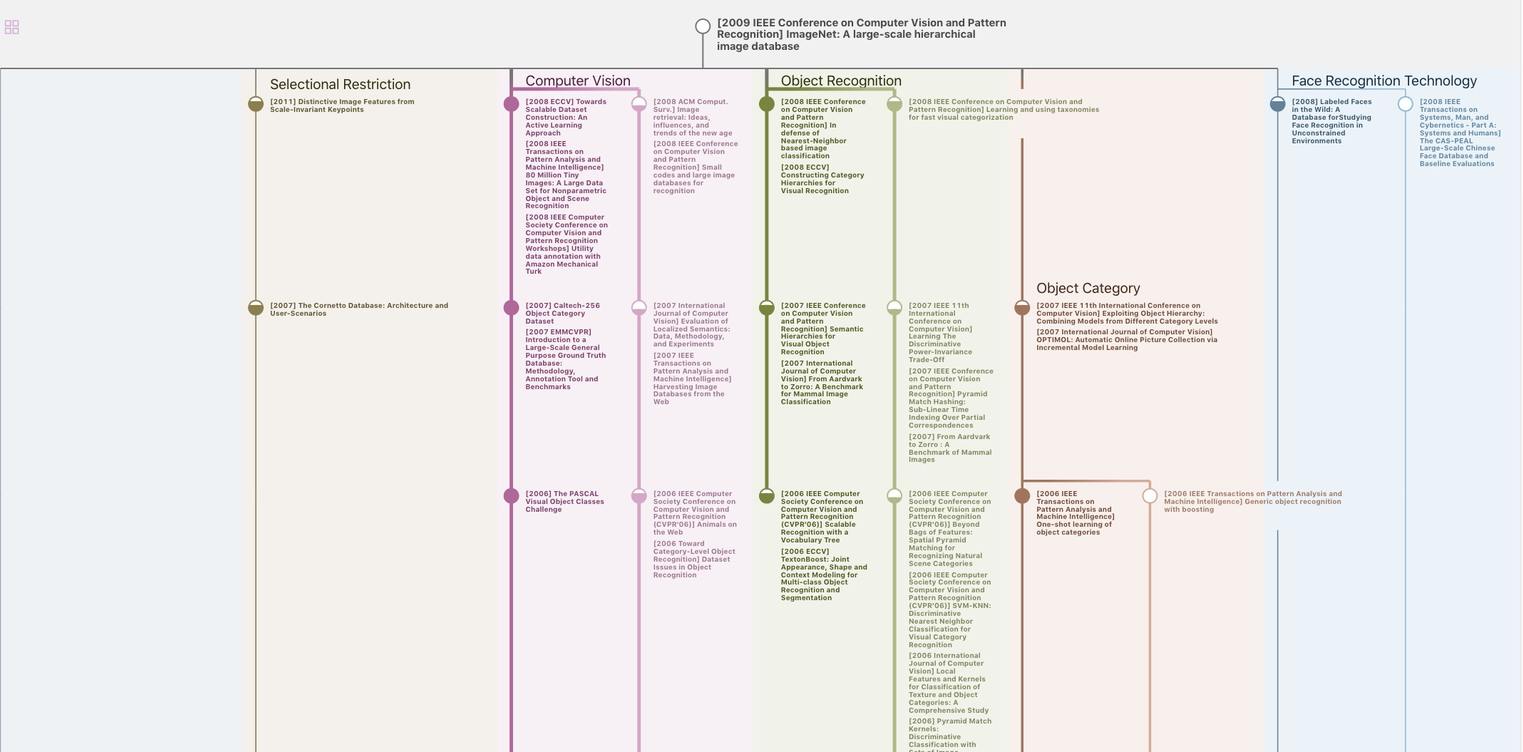
生成溯源树,研究论文发展脉络
Chat Paper
正在生成论文摘要