Avoiding collaborative paradox in multi-agent reinforcement learning
ETRI JOURNAL(2021)
摘要
The collaboration productively interacting between multi-agents has become an emerging issue in real-world applications. In reinforcement learning, multi-agent environments present challenges beyond tractable issues in single-agent settings. This collaborative environment has the following highly complex attributes: sparse rewards for task completion, limited communications between each other, and only partial observations. In particular, adjustments in an agent's action policy result in a nonstationary environment from the other agent's perspective, which causes high variance in the learned policies and prevents the direct use of reinforcement learning approaches. Unexpected social loafing caused by high dispersion makes it difficult for all agents to succeed in collaborative tasks. Therefore, we address a paradox caused by the social loafing to significantly reduce total returns after a certain timestep of multi-agent reinforcement learning. We further demonstrate that the collaborative paradox in multi-agent environments can be avoided by our proposed effective early stop method leveraging a metric for social loafing.
更多查看译文
关键词
lazy agent problem, multi-agent reinforcement learning, multi-agent systems, social dilemmas
AI 理解论文
溯源树
样例
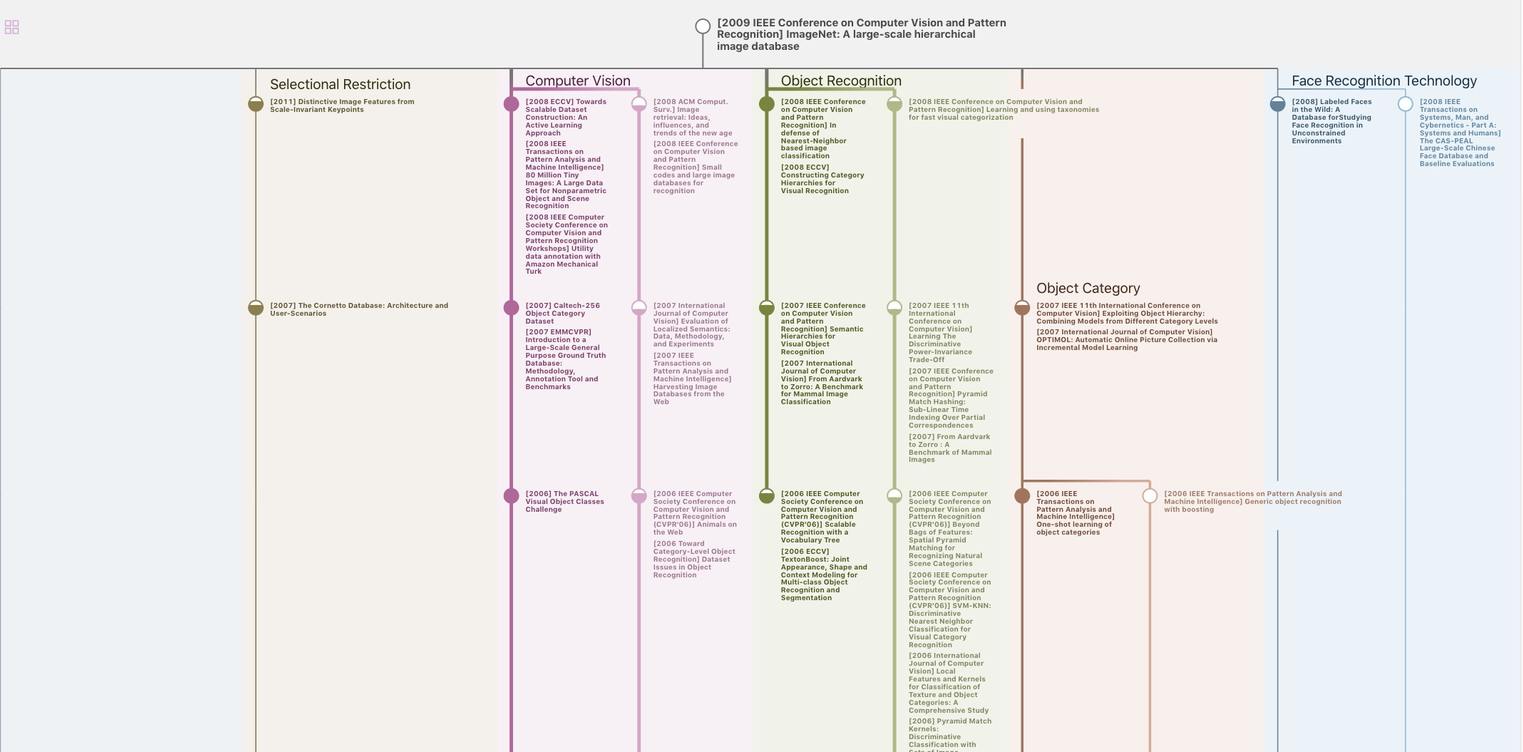
生成溯源树,研究论文发展脉络
Chat Paper
正在生成论文摘要