An Efficient Intrusion Prevention System for CAN: Hindering Cyber-Attacks With a Low-cost Platform
IEEE ACCESS(2021)
摘要
The controller area network (CAN), which is still today the most used in-vehicle network, does not provide any security or authentication mechanism by design. Since current vehicles, which have numerous connectivity technologies, such as Bluetooth, Wi-Fi, and cellular radio, can be easily accessed from the exterior world, they can be easy targets of cyber-attacks. It is therefore urgently necessary to enhance vehicle security by detecting and stopping cyber-attacks. In this paper, we propose a novel unsupervised intrusion prevention system (IPS) for automotive CANs that detects and hinders attacks without modifying the architecture of the electronic control units (ECUs) or requiring information that is restricted to car manufacturers. We compare two machine learning algorithms' ability to detect fuzzing and spoofing attacks, and evaluate which of them is most accurate with the fewest number of data bytes. The fewer data bytes required, the sooner detection can start and the sooner attacking frames can be detected. Experiment results show that our proposed detection mechanism achieves accuracy higher than 99%, F1-scores higher than 97%, and detection times shorter than 80 mu s for the types of attacks considered. Moreover, when compared to four state-of-the-art intrusion detection systems, it is the only solution that is capable of discarding attacking frames before damage occurs while being deployed on inexpensive Raspberry Pi. Such an inexpensive deployment is particularly desirable, as cost is one of the automotive industry's primary concerns.
更多查看译文
关键词
Intrusion detection system (IDS), intrusion prevention system (IPS), machine learning, controller area network (CAN)
AI 理解论文
溯源树
样例
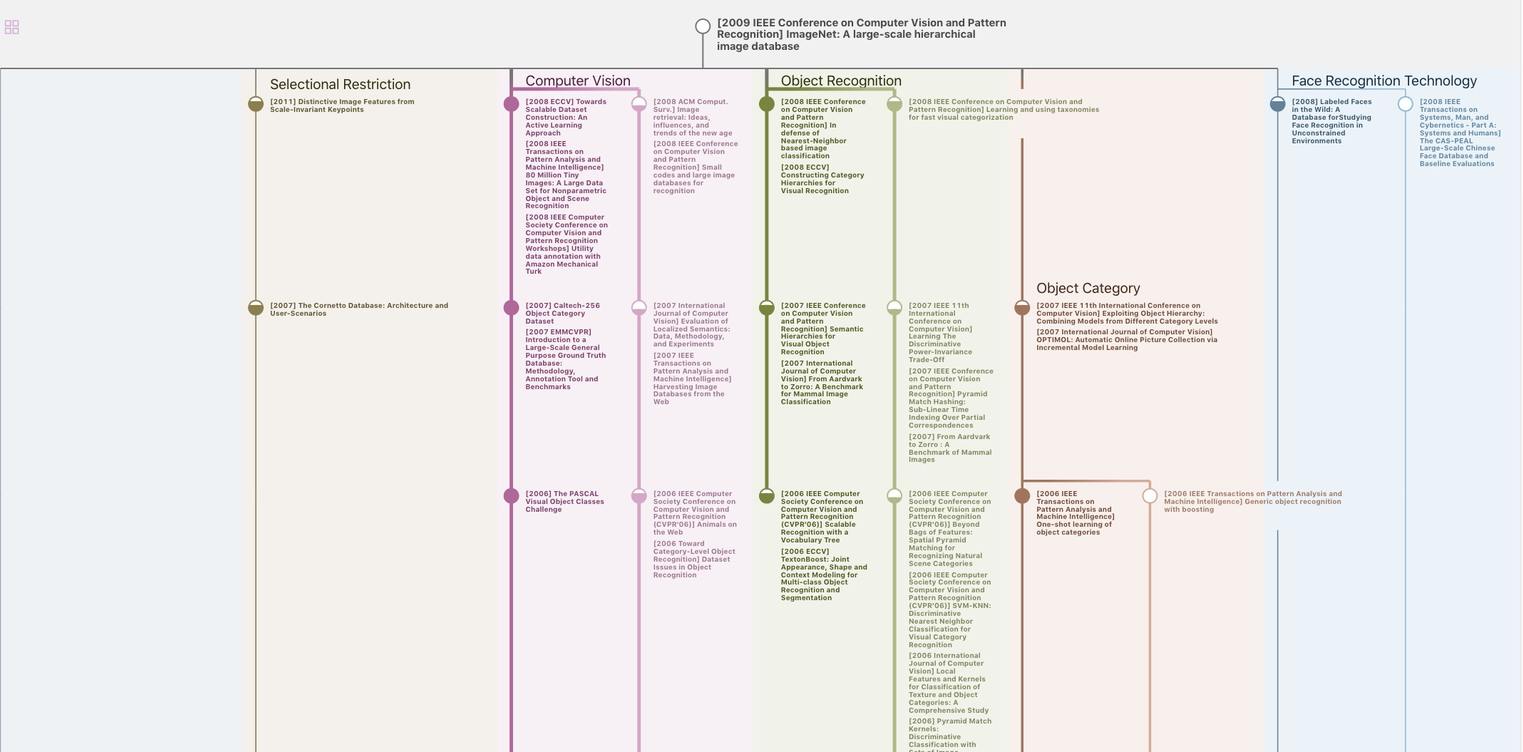
生成溯源树,研究论文发展脉络
Chat Paper
正在生成论文摘要