In-depth research on the interpretable disruption predictor in HL-2A
NUCLEAR FUSION(2021)
摘要
In-depth research is implemented on the disruption predictor in HL-2A to improve the accuracy and interpretability of the model. For higher accuracy, four adjustments are tried to solve four corresponding problems in a baseline model. Reductive comparison experiments are designed to evaluate their contribution to performance. The result shows that these adjustments together can improve the AUC (area under receiver operating characteristic curve) of the baseline model by 0.039. For interpretability of model, an interpretation method is proposed to evaluate the real-time importance of each input signal. The result of single shot interpretation shows good coherence with the causes of disruptions. To further validate the reasonability of this interpretation method, disruption causes of shot nos. 20000-36000 are analysed to make a disruption cause dataset. Statistical analysis of the output of the interpretation algorithm on this dataset also shows a good coherence with the disruption causes. Then a Bayes classifier is developed to recognize the cause of disruption based on the interpretation algorithm's output. This classifier has an accuracy of 71.2% on the labelled dataset, which contains 605 disruptive shots categorized into five disruption causes.
更多查看译文
关键词
disruption prediction, deep learning, interpretability, disruption causes, HL-2A
AI 理解论文
溯源树
样例
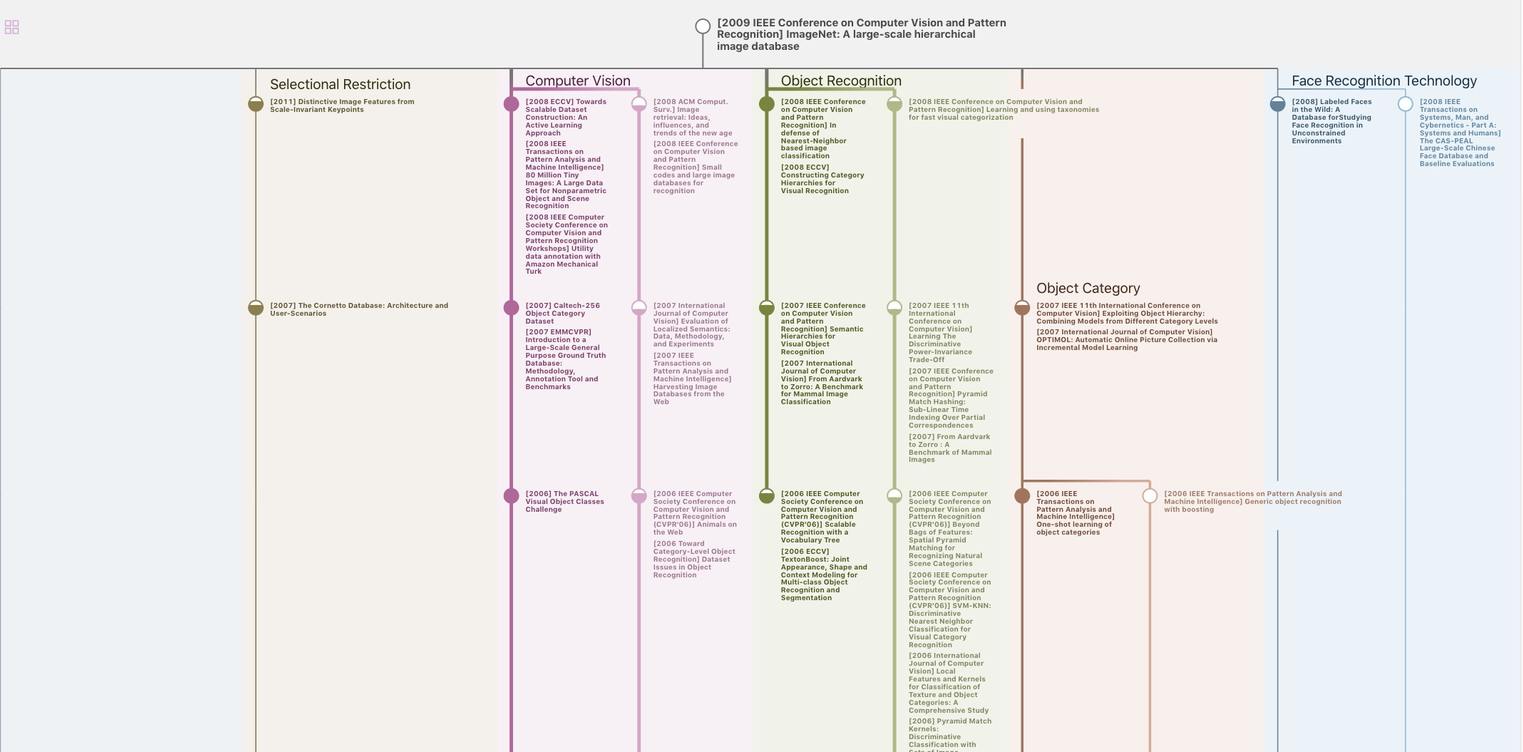
生成溯源树,研究论文发展脉络
Chat Paper
正在生成论文摘要