Learning Under Concept Drift for Regression-A Systematic Literature Review
IEEE ACCESS(2022)
摘要
Context: The amount and diversity of data have increased drastically in recent years. However, in certain situations, the data to which a trained Machine Learning model is significantly different from testing data, a problem known as Concept Drift (CD). Because CD can be a serious issue, there has been a wealth of research on how to detect and work around it. However, most of the literature focuses on classification tasks. Objective: Making a Systematic Literature Review (SLR) for CD in the context of regression. Research questions: How to detect CD and how to build CD techniques for regression problems using machine learning? Method: We ran an automatic search process on reference databases, selecting papers from 2010 to August 2020, following the methodological process proposed by (Kitchenhame and Charters) (2007). Results:We selected 41 papers. Drift Detection Methods based on ensembles and neural networks with highlight OS-ELM were the most frequent in the selected papers with superior performance. However, only two papers confirm such superiority statistically. Furthermore, identify CD problems as the batch size, drift points, and where drift happens. Conclusions: SLR focuses on highlighting the existing literature on CD applied to regression.
更多查看译文
关键词
Machine learning, Detectors, Data models, Adaptation models, Task analysis, Classification algorithms, Training, Concept drift, data stream, ensemble, regression, non-stationary environments, systematic literature review
AI 理解论文
溯源树
样例
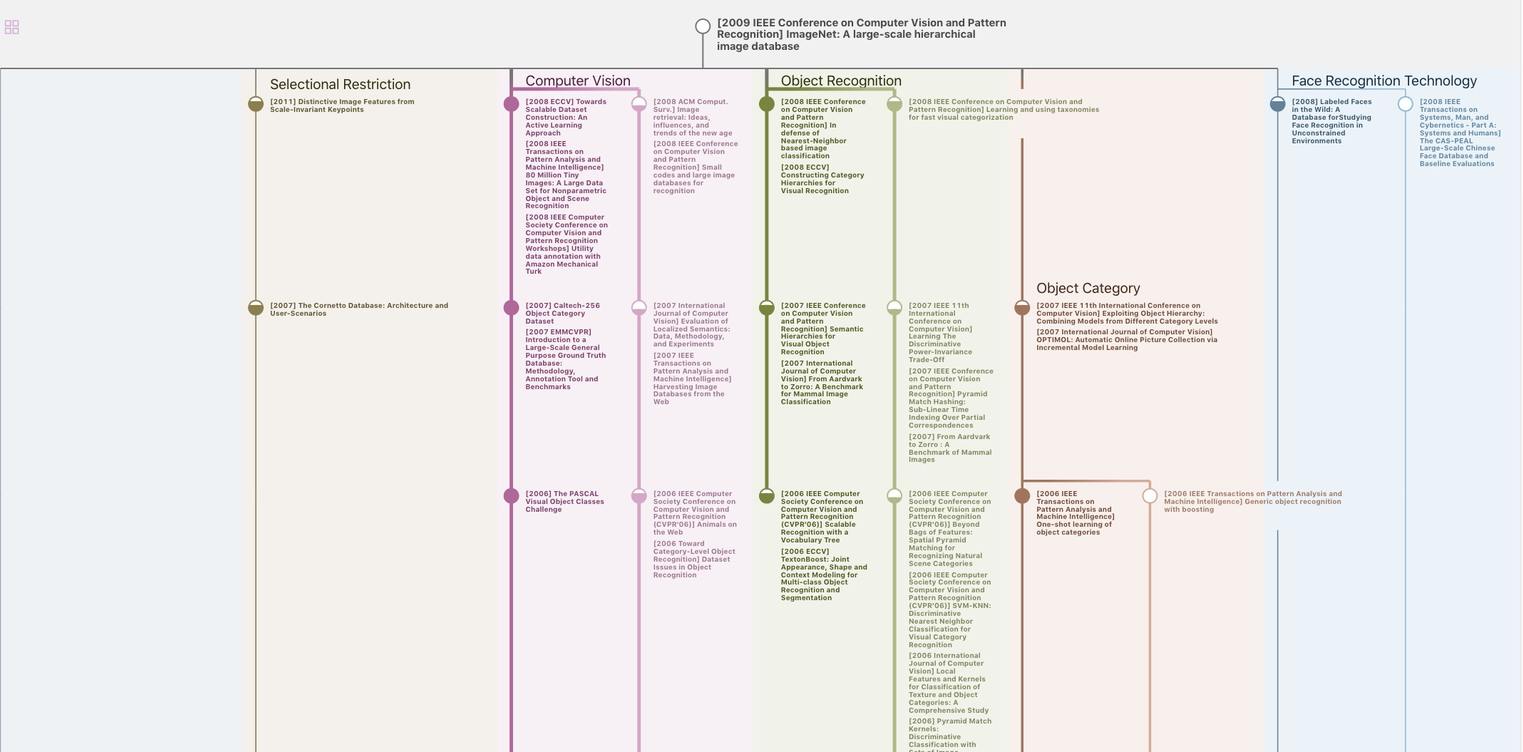
生成溯源树,研究论文发展脉络
Chat Paper
正在生成论文摘要