SimpleMeshNet: end to end recovery of 3d body mesh with one fully connected layer
Journal of Real-Time Image Processing(2022)
摘要
According to recent research, reconstructing high-precision 3D human body shape and pose using neural networks necessitates not just large datasets with ground-truth 3D annotations, but also depends significantly on sophisticated network structures to utilize spatial and temporal information. Employing these strategies will also make training more difficult and time-consuming. We proposed SimpleMeshNet, the simplest frame-based model to present, to estimate 3D human body mesh for in-the-wild images. On the one hand, the SimpleMeshNet contains just one fully connected layer after extracting the features and utilizing a pre-trained ResNet as a regressor to output the SMPL model parameters; on the other hand, it performed well and runs fairly fast. To minimize overfitting concerns when the ground-truth SMPL annotations are missing, SimpleMeshNet employs two different training strategies when training the network with or without ground-truth SMPL parameter annotations. Without bells and whistles, the network is quite easy to train and the results are highly convincing. In comparison to other methods, SimpleMeshNet's performance is measured using a video with five persons and an RTX3090 GPU. SimpleMeshNet alone can achieve 107 frames per second, whereas the whole system can get 45 frames per second while using YOLOv3-416 as a tracker. Compared with the leading algorithms, the performance of SimpleMeshNet can rival them, sometimes even better. What’s more, SimpleMeshNet can be used to process different in-the-wild images captured by a variety of devices: cell phones, monitors, cameras, and more.
更多查看译文
关键词
3D human pose and shape estimation, In-the-wild images, Fully connected layer
AI 理解论文
溯源树
样例
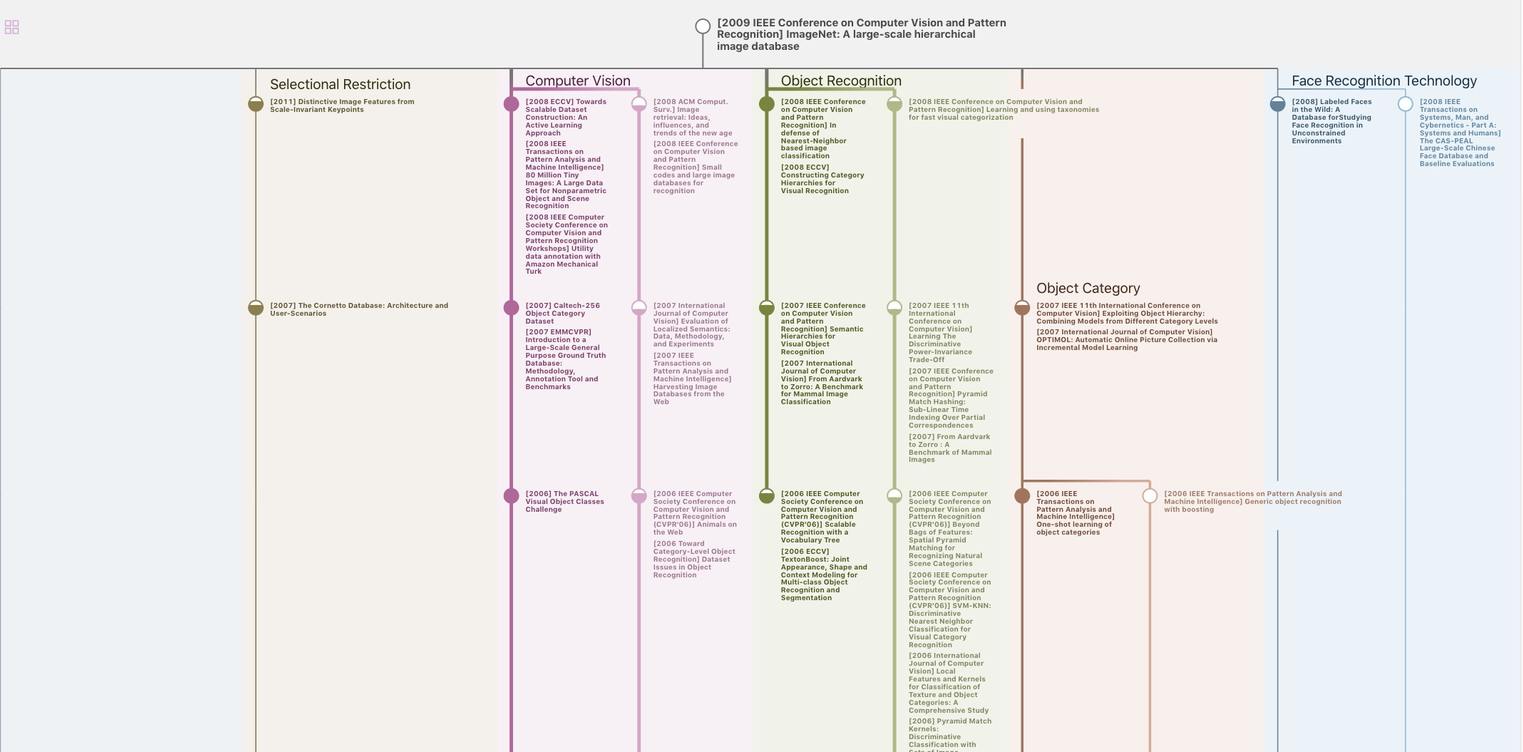
生成溯源树,研究论文发展脉络
Chat Paper
正在生成论文摘要