Intelligent modeling of nonlinear dynamical systems by machine learning
International Journal of Non-Linear Mechanics(2022)
摘要
An intelligent data-driven method of modeling nonlinear dynamical systems named as LSTM network with output recurrence (OR-LSTM) is proposed, which can learn the inherent characteristics of the dynamical systems from data and predict the states of the systems given external excitation and initial conditions. For the proposed OR-LSTM architecture, the most important key point is output recurrence connections. In order to evaluate it, a teacher-forcing LSTM network without output recurrence connections (TF-LSTM) is designed for comparison. The differences between OR-LSTM and TF-LSTM from the perspective of gradient propagation and parameter updating are analyzed firstly. To verify the capability of the LSTM networks in dynamical systems modeling, the oscillation of a 42-story reinforced concrete frame-core tube building under severe and extreme seismic excitations are modeled. The results show that the OR-LSTM network performs well and maintains the long-term robustness in earthquake responses prediction, even only known displacements of 9 stories. However, the TF-LSTM network fails. In order to evaluate the extensive practicability of the OR-LSTM network, the famous Van der Pol dynamic system and Lorenz dynamic system with strong nonlinearity are also modeled by the proposed method. The results show that OR-LSTM network is competent in modeling most of nonlinear dynamic systems, while has limited in fully chaotic systems, but the predictable length of time sequence and prediction accuracy are improved.
更多查看译文
关键词
Nonlinear dynamic system,Modeling,LSTM,Output recurrence connections,Long-term robustness
AI 理解论文
溯源树
样例
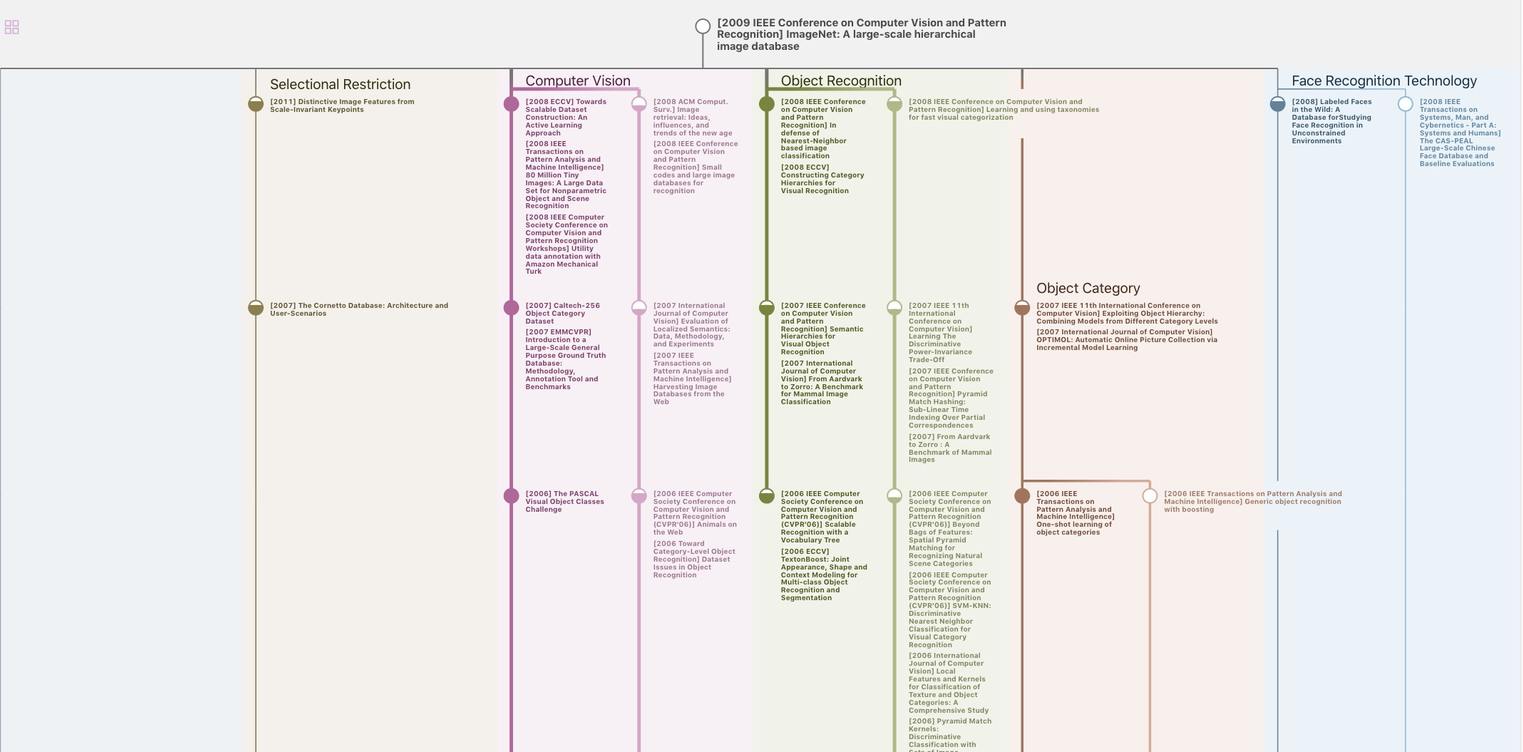
生成溯源树,研究论文发展脉络
Chat Paper
正在生成论文摘要