Automatic Itinerary Planning Using Triple-Agent Deep Reinforcement Learning
IEEE TRANSACTIONS ON INTELLIGENT TRANSPORTATION SYSTEMS(2022)
摘要
Automatic itinerary planning that provides an epic journey for each traveler is a fundamental yet inefficient task. Most existing planning methods apply heuristic guidelines for certain objective, and thereby favor popular preferred point of interests (POIs) with high probability, which ignore the intrinsic correlation between the POIs exploration, traveler's preferences, and distinctive attractions. To tackle the itinerary planning problem, this paper explores the connections of these three objectives in probabilistic manner based on a Bayesian model and proposes a triple-agent deep reinforcement learning approach, which generates 4-way direction, 4-way distance, and 3-way selection strategy for iteratively determining next POI to visit in the itinerary. Experiments on five real-world cities demonstrate that our triple-agent deep reinforcement learning approach can provide better planning results in comparison with state-of-the-art multiobjective optimization methods.
更多查看译文
关键词
Planning,Search problems,Reinforcement learning,Urban areas,Space exploration,Probabilistic logic,Computer science,Automatic itinerary planning,deep reinforcement learning,multiobjective optimization
AI 理解论文
溯源树
样例
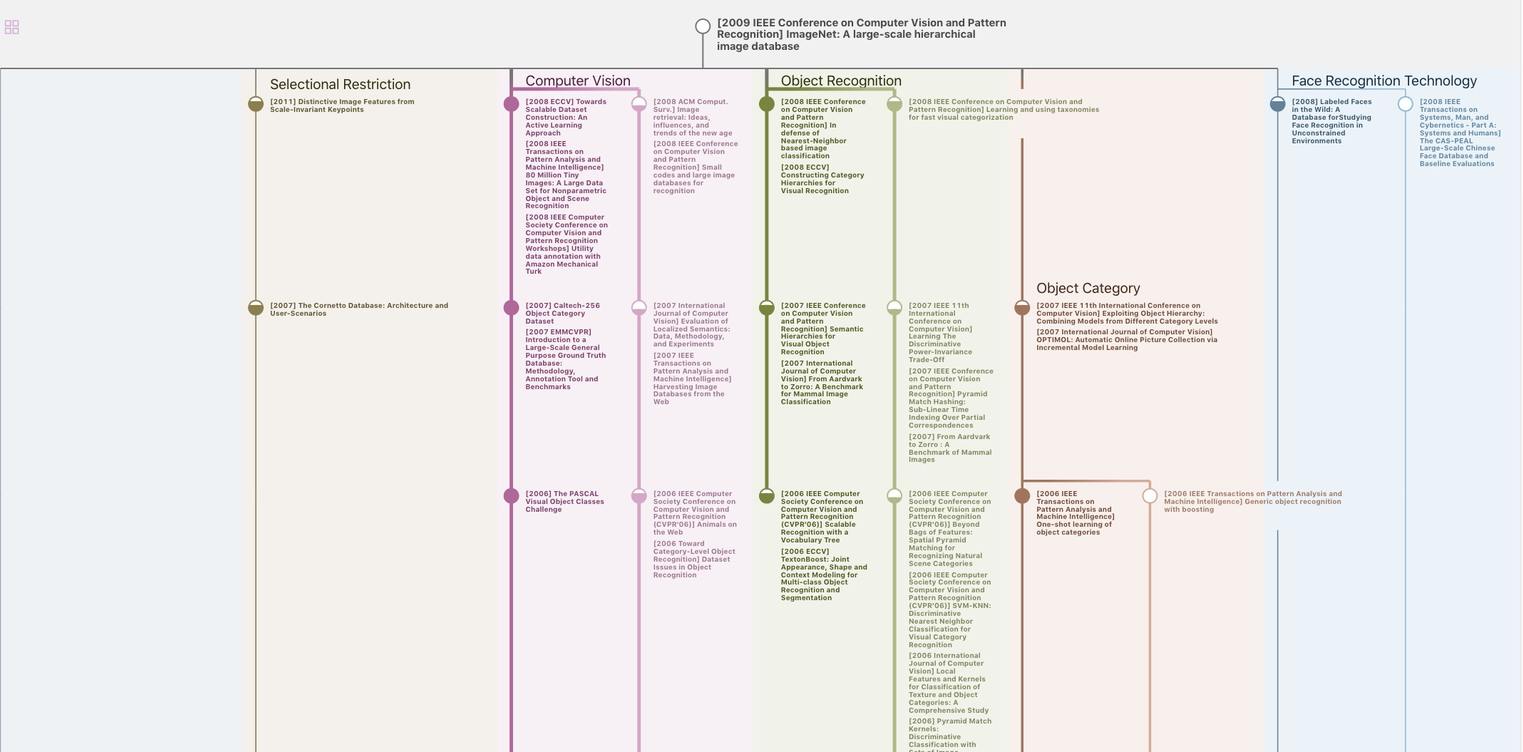
生成溯源树,研究论文发展脉络
Chat Paper
正在生成论文摘要