On the Estimation of the SHARP Parameter MEANALP from AIA Images Using Deep Neural Networks
SOLAR PHYSICS(2021)
摘要
Space-weather HMI Active Region Patches (SHARPs) data from the Helioseismic and Magnetic Imager (HMI) on board the Solar Dynamics Observatory (SDO) provides high cadence data from the full-disk photospheric magnetic field. The SHARP’s MEANALP ( α _m ) parameter, which characterizes the twist, can provide a measure of nonpotentiality of an active region, which can be a condition for the occurrence of solar flares. The SDO/Atmospheric Imaging Assembly (AIA) captures images at a higher cadence (12 or 24 seconds) than the SDO/HMI. Hence, if the α _m can be inferred from the AIA data, we can estimate the magnetic field evolution of an active region at a higher temporal cadence. Shortly before a flare occurs, we observed a change in the α _m in some active regions that produced stronger (M- or X-class) flares. Therefore, we study the ability of neural networks to estimate the α _m parameter from SDO/AIA images. We propose a classification and regression scheme to train deep neural networks using AIA filtergrams of active regions with the objective to estimate the α _m of active regions outside our training set. Our results show a classification accuracy greater than 85
更多查看译文
关键词
Deep neural networks,Active regions,SHARP data,SHARP MEANALP
AI 理解论文
溯源树
样例
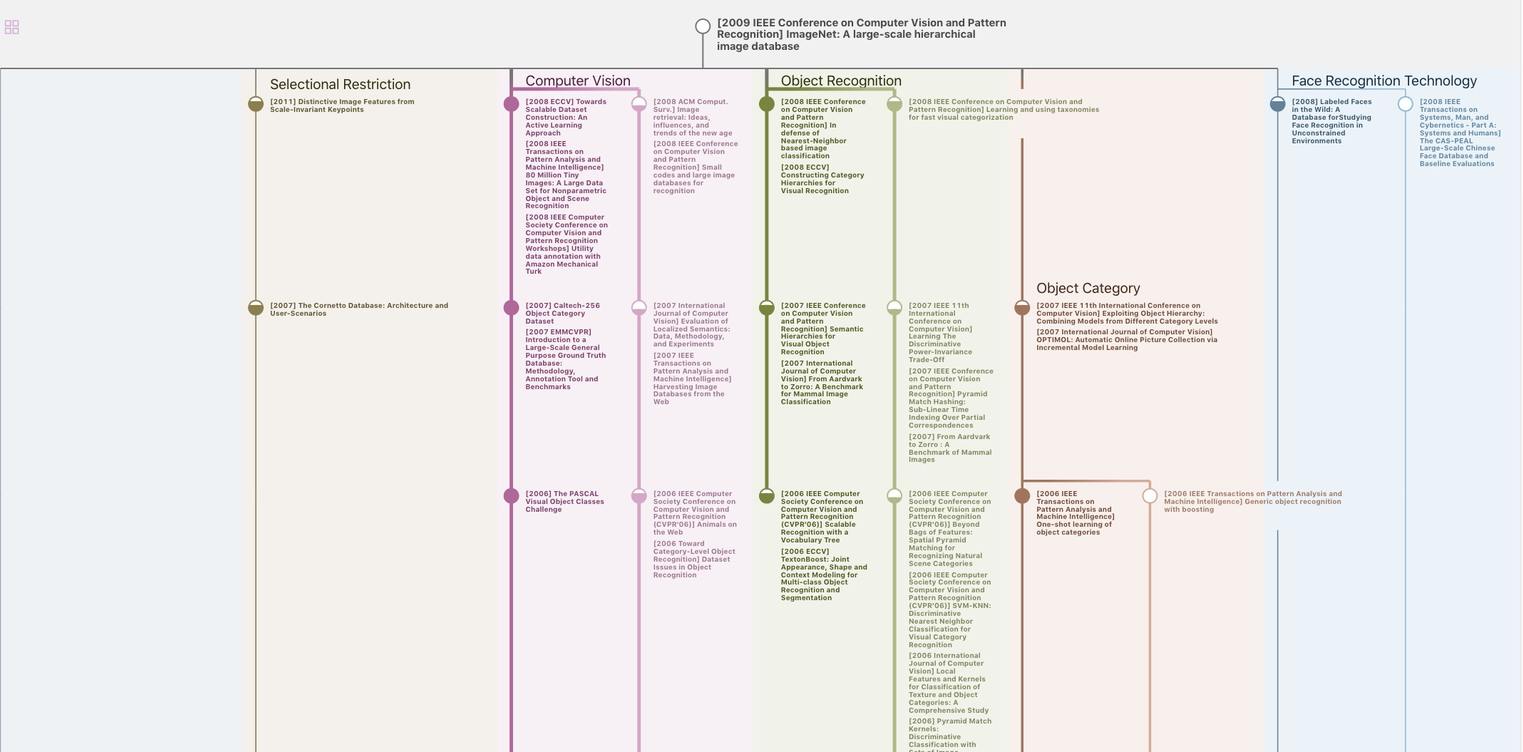
生成溯源树,研究论文发展脉络
Chat Paper
正在生成论文摘要