Online Binary Incomplete Multi-view Clustering
MACHINE LEARNING AND KNOWLEDGE DISCOVERY IN DATABASES, ECML PKDD 2020, PT I(2021)
摘要
Multi-view clustering has attracted considerable attention in the past decades, due to its good performance on the data with multiple modalities or from diverse sources. In real-world applications, multi-view data often suffer from incompleteness of instances. Clustering on such multi-view data is called incomplete multi-view clustering (IMC). Most of the existing IMC solutions are offline and have high computational and memory costs especially for large-scale datasets. To tackle these challenges, in this paper, we propose a Online Binary Incomplete Multiview Clustering (OBIMC) framework. OBIMC robustly learns the common compact binary codes for incomplete multi-view features. Moreover, the cluster structures are optimized with the binary codes in an online fashion. Further, we develop an iterative algorithm to solve the resultant optimization problem with linear computational complexity and theoretically prove its convergence. Experiments on four real datasets demonstrate the efficiency and effectiveness of the proposed OBIMC method. As indicated, our algorithm significantly and consistently outperforms some state-of-the-art algorithms with much less running time.
更多查看译文
关键词
clustering,online,multi-view
AI 理解论文
溯源树
样例
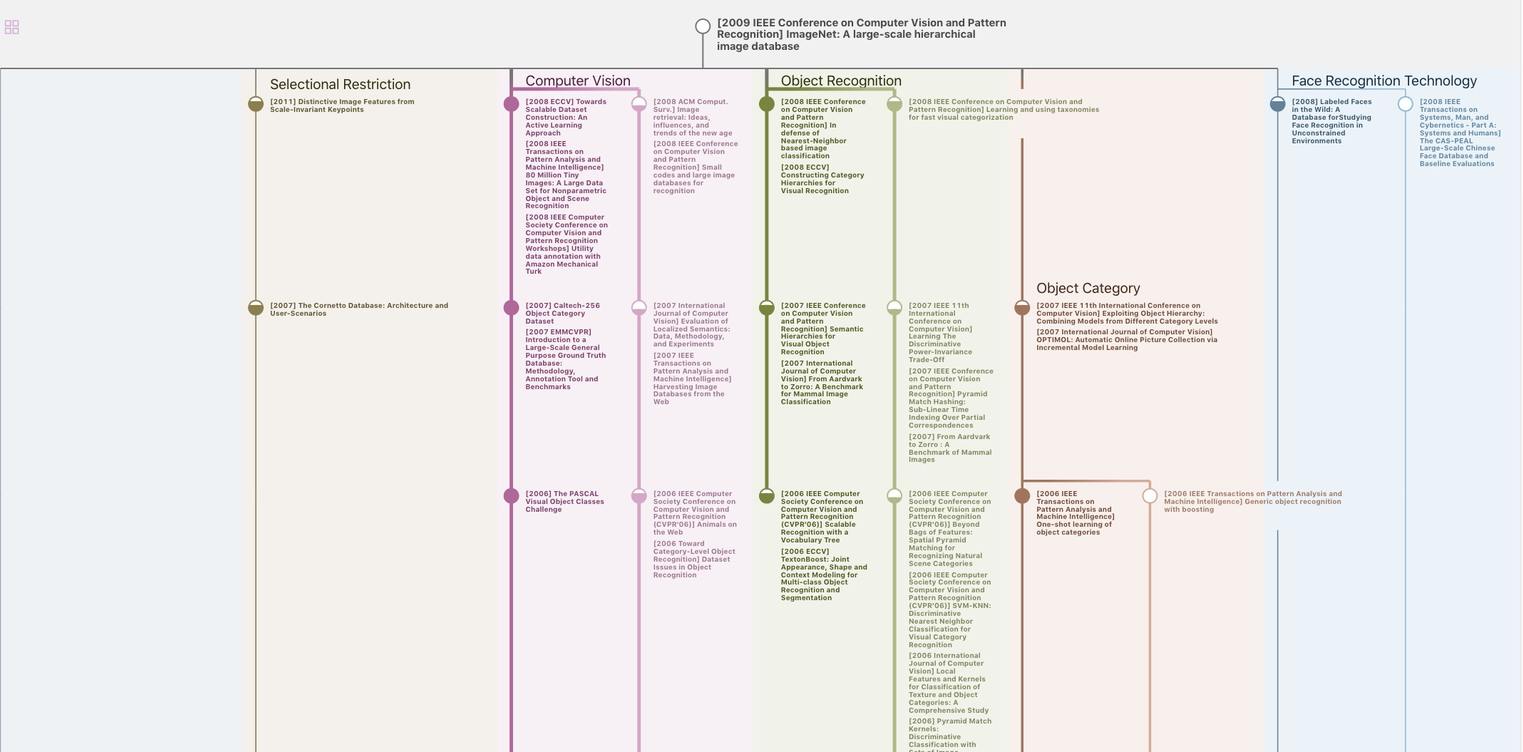
生成溯源树,研究论文发展脉络
Chat Paper
正在生成论文摘要