Application of artificial neural network to estimate the quality of little auks' potential foraging grounds on Spitsbergen
LIMNOLOGY AND OCEANOGRAPHY-METHODS(2022)
摘要
The availability of food for the zooplanktivorous seabirds, such as an endemic High Arctic alcid, the little auk (Alle alle) is essential for its population status and in consequence for fertilizing nutrient-poor Svalbard tundra. Since the zooplankton composition and concentration vary over time and space on foraging grounds, it is challenging to monitor these changes and it could be facilitated by using original machine-learning methods. We propose to use supervised artificial neural network (ANN) with back-propagation algorithm, in which we rank each zooplankton taxonomical category (ZTC) based on its share in biomass of little auks' diet. The highest rank was assigned to the largest and most frequent prey, for example, fifth copepodid stage (CV) of Calanus glacialis (0.75), amphipod Apherusa glacialis (0.17) and females of Calanus hyperboreus (0.12). In order to qualify the potential foraging grounds for little auks, zooplankton samples were collected at sea, in the vicinity of five West Spitsbergen fjords characterized by different oceanographic conditions. Consequently, the southern and middle fjords of Spitsbergen were described as the best foraging grounds, while the lowest quality was designated to the northernmost locations. ANN was validated with independent long-term monitoring dataset from Hornsund and 78% of stations were classified correctly, which indicates that the presented method is reliable for quick estimation of little auks' foraging grounds qualities. This research proposes a new, automated approach for potential foraging grounds classification and delivers an open-access application that allows following and predicting changes in an emblematic Arctic predator-prey relationship (little auk-zooplankton).
更多查看译文
AI 理解论文
溯源树
样例
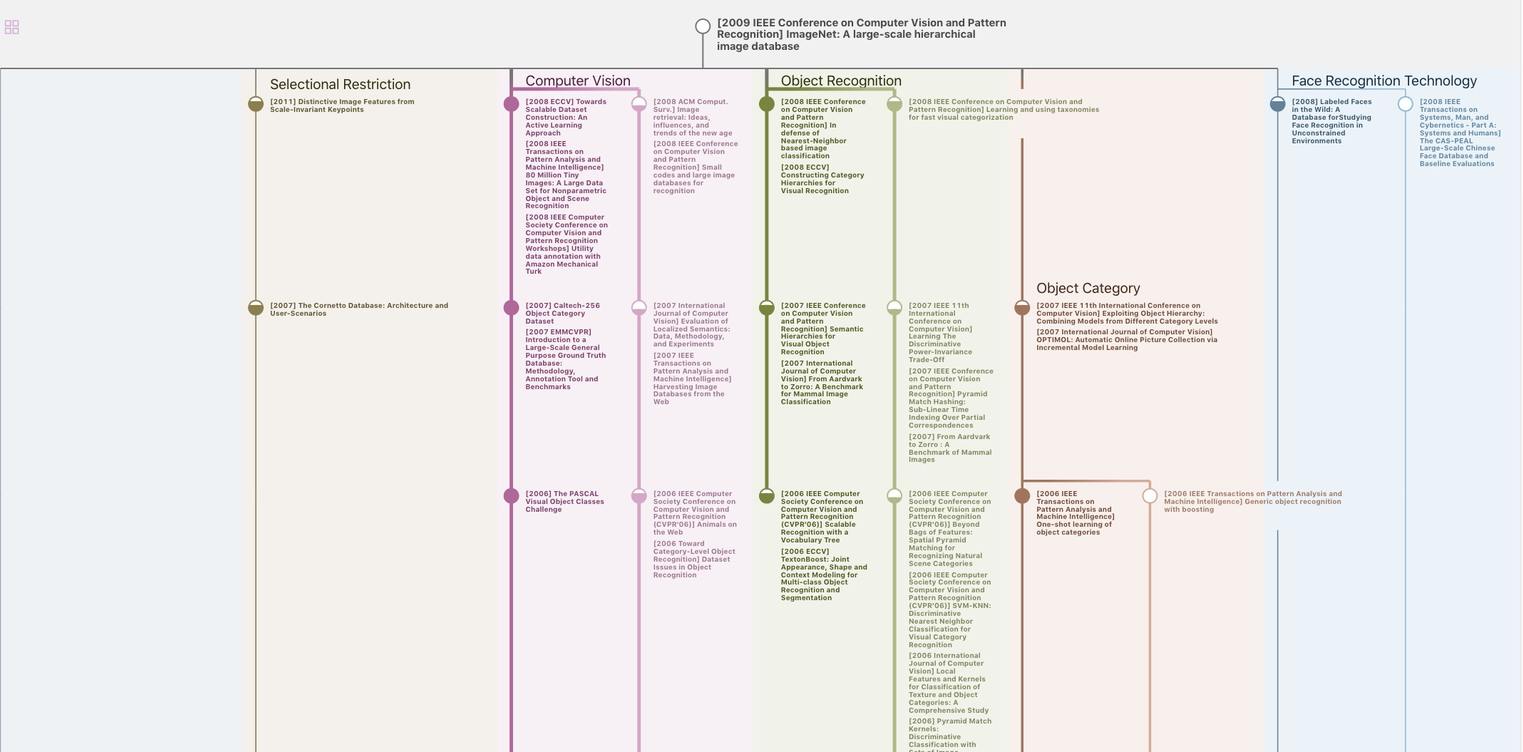
生成溯源树,研究论文发展脉络
Chat Paper
正在生成论文摘要