Robust Pareto solutions for convex quadratic multiobjective optimization problems under data uncertainty
ANNALS OF OPERATIONS RESEARCH(2022)
摘要
In this paper, we consider a convex quadratic multiobjective optimization problem, where both the objective and constraint functions involve data uncertainty. We employ a deterministic approach to examine robust optimality conditions and find robust (weak) Pareto solutions of the underlying uncertain multiobjective problem. We first present new necessary and sufficient conditions in terms of linear matrix inequalities for robust (weak) Pareto optimality of the multiobjective optimization problem. We then show that the obtained optimality conditions can be alternatively checked via other verifiable criteria including a robust Karush–Kuhn–Tucker condition. Moreover, we establish that a (scalar) relaxation problem of a robust weighted-sum optimization program of the multiobjective problem can be solved by using a semidefinite programming (SDP) problem. This provides us with a way to numerically calculate a robust (weak) Pareto solution of the uncertain multiobjective problem as an SDP problem that can be implemented using, e.g., MATLAB.
更多查看译文
关键词
Multiobjective program, Robust optimization, Optimality condition, Semidefinite programming, Relaxation
AI 理解论文
溯源树
样例
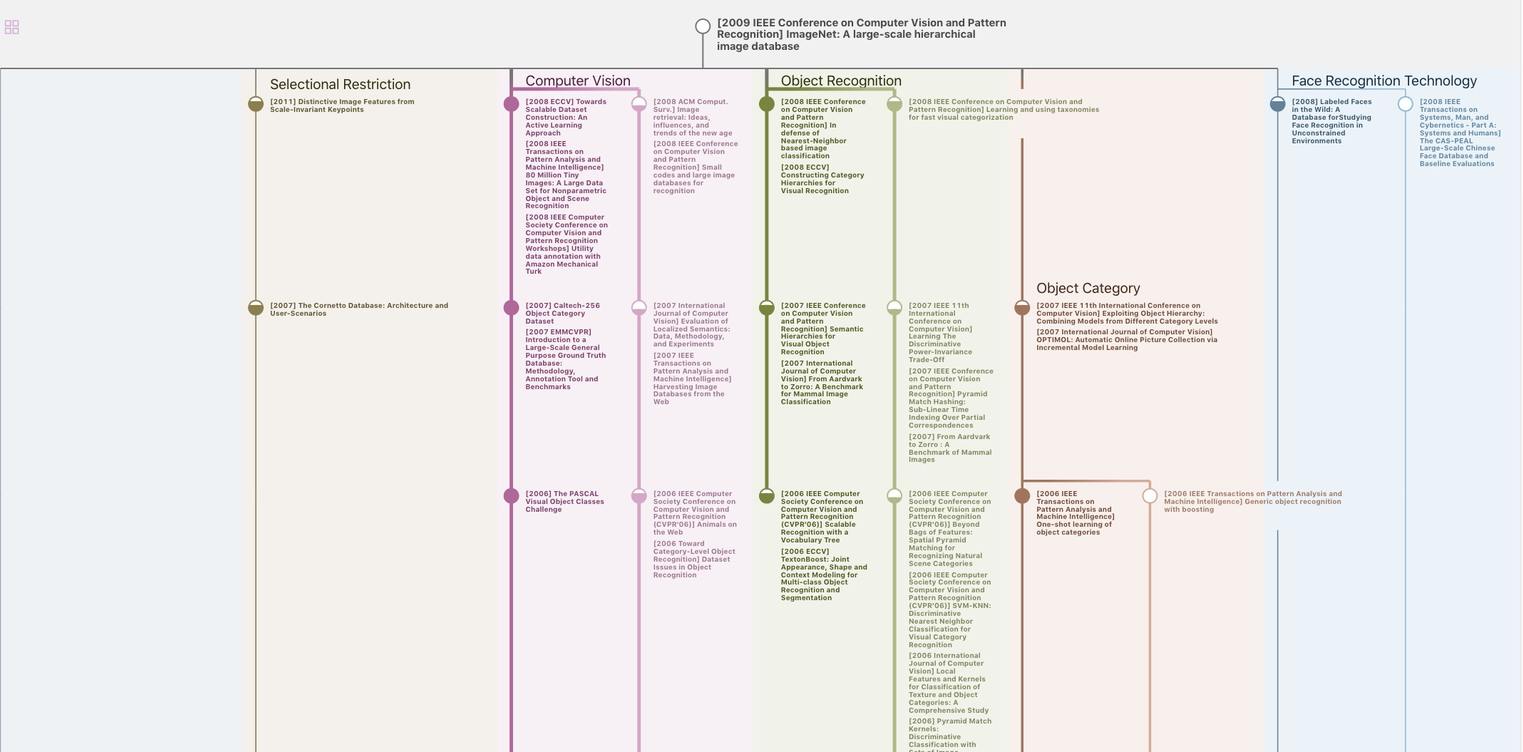
生成溯源树,研究论文发展脉络
Chat Paper
正在生成论文摘要