Robust Supervised and Semisupervised Least Squares Regression Using ℓ $$ _ {2, p} $-Norm Minimization
IEEE TRANSACTIONS ON NEURAL NETWORKS AND LEARNING SYSTEMS(2022)
摘要
Least squares regression (LSR) is widely applied in statistics theory due to its theoretical solution, which can be used in supervised, semisupervised, and multiclass learning. However, LSR begins to fail and its discriminative ability cannot be guaranteed when the original data have been corrupted and noised. In reality, the noises are unavoidable and could greatly affect the error construction in LSR. To cope with this problem, a robust supervised LSR (RSLSR) is proposed to eliminate the effect of noises and outliers. The loss function adopts
$\ell _{2,p}$
-norm (
$0< p\leq 2$
) instead of square loss. In addition, the probability weight is added to each sample to determine whether the sample is a normal point or not. Its physical meaning is very clear, in which if the point is normal, the probability value is 1; otherwise, the weight is 0. To effectively solve the concave problem, an iterative algorithm is introduced, in which additional weights are added to penalize normal samples with large errors. We also extend RSLSR to robust semisupervised LSR (RSSLSR) to fully utilize the limited labeled samples. A large number of classification performances on corrupted data illustrate the robustness of the proposed methods.
更多查看译文
关键词
l(2,p)-norm, least squares regression (LSR), robust, supervised and semisupervised classification
AI 理解论文
溯源树
样例
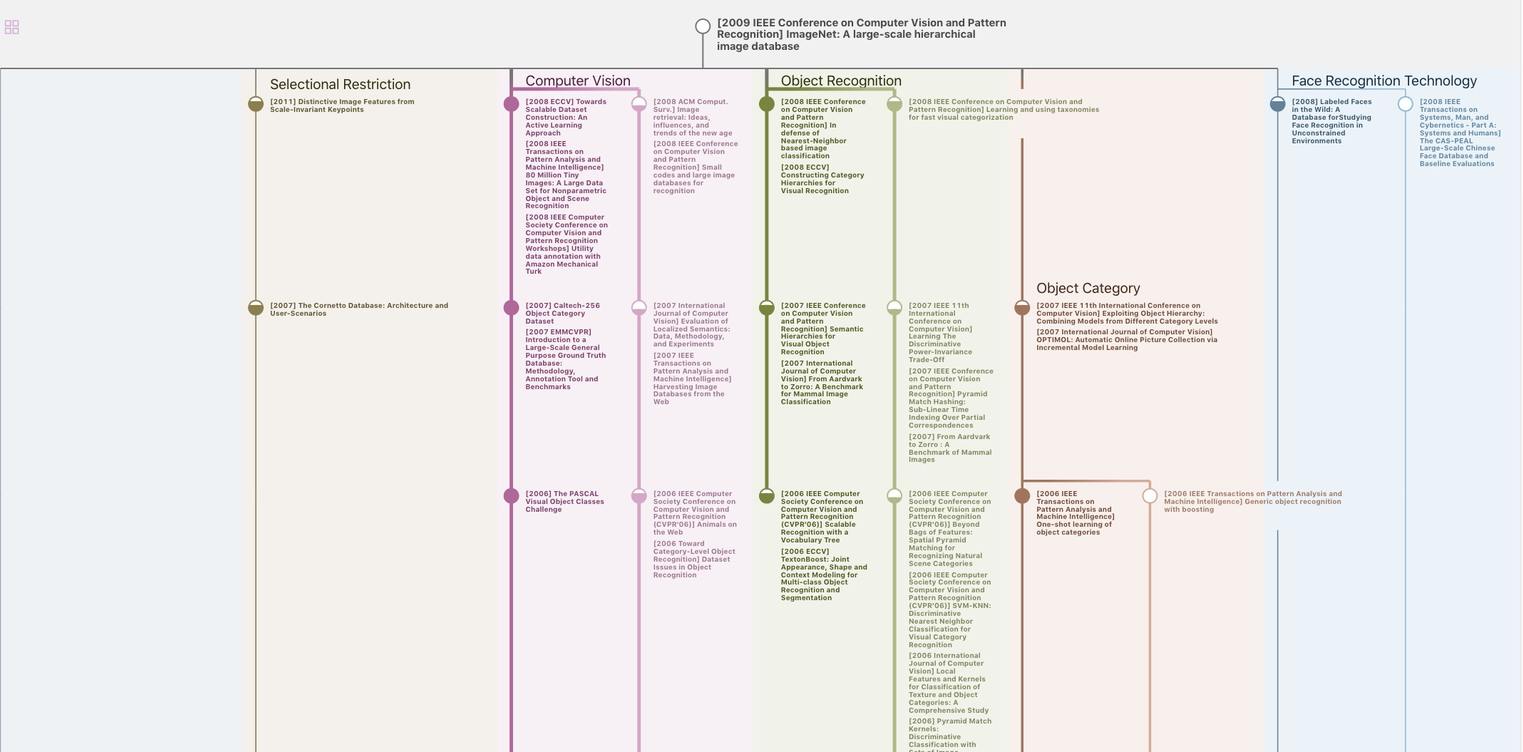
生成溯源树,研究论文发展脉络
Chat Paper
正在生成论文摘要