From Scene Flow to Visual Odometry Through Local and Global Regularisation in Markov Random Fields
IEEE ROBOTICS AND AUTOMATION LETTERS(2022)
摘要
We revisit pairwise Markov Random Field (MRF) formulations for RGB-D scene flow and leverage novel advances in processor design for real-time implementations. We consider scene flow approaches which consist of data terms enforcing intensity consistency between consecutive images, together with regularisation terms which impose smoothness over the flow field. To achieve real-time operation, previous systems leveraged GPUs and implemented regularisation only between variables corresponding to neighbouring pixels. Such systems could estimate continuously deforming flow fields but the lack of global regularisation over the whole field made them ineffective for visual odometry. We leverage the GraphCore Intelligence Processing Unit (IPU) graph processor chip, which consists of 1216 independent cores called tiles, each with 256 kB local memory. The tiles are connected to an ultrafast all-to-all communication fabric which enables efficient data transmission between the tiles in an arbitrary communication pattern. We propose a distributed formulation for dense RGB-D scene flow based on Gaussian Belief Propagation which leverages the architecture of this processor to implement both local and global regularisation. Local regularisation is enforced for pairs of flow estimates whose corresponding pixels are neighbours, while global regularisation is defined for flow estimate pairs whose corresponding pixels are far from each other on the image plane. Using both types of regularisation allows our algorithm to handle a variety of in-scene motion and makes it suitable for estimating deforming scene flow, piece-wise rigid scene flow and visual odometry within the same system.
更多查看译文
关键词
Visual odometry, Smoothing methods, Three-dimensional displays, Real-time systems, Rigidity, Image motion analysis, Belief propagation, Hardware-software integration in robotics, RGB-D perception, visual tracking
AI 理解论文
溯源树
样例
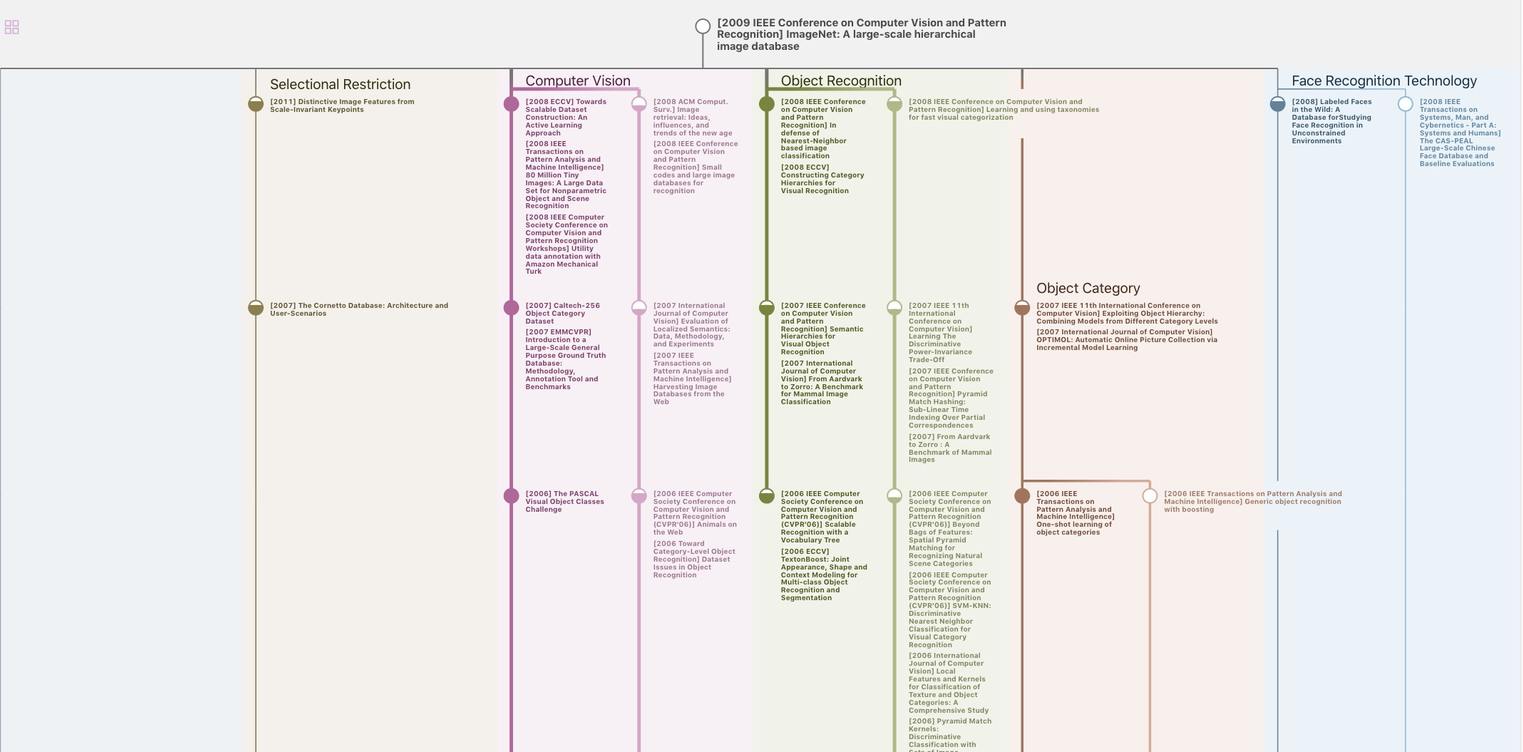
生成溯源树,研究论文发展脉络
Chat Paper
正在生成论文摘要