Artificial Neural Network Potential for Encapsulated Platinum Clusters in MOF-808
JOURNAL OF PHYSICAL CHEMISTRY C(2022)
摘要
Metal-organic frameworks (MOFs) have been recognized as one of the ideal supporting sintering-resistant catalyst materials because of their high specific surface area and unique nano-porous structure capable of manipulating the sizes and shapes of encapsulated metal clusters. To explore the binding sites, the stability, and migration mechanisms of encapsulated metal clusters in MOF materials, a robust potential model that accurately describes the interaction between metal clusters and MOF materials is highly desired for large-scale atomic simulations. Herein, as a demonstration case, an artificial neural network potential for encapsulated platinum (Pt) clusters in MOF-808 was developed using the machine learning-based global neural network (G-NN) technique. The artificial G-NN potential was tested and validated against a series of density functional theory calculation data, including structure optimization, adsorption energies, and the migration energy barrier of Pt-n (n = 1-13) clusters in MOF-808. The newly developed Pt-MOF G-NN potential was further used to predict the adsorption and migration behaviors of Pt-n clusters in MOF-808. It is found that the most stable adsorption site varies with the Pt-n cluster size. The migration possibility of the Pt-n cluster is strongly correlated with the adsorption energies of the Pt-n clusters. Finally, the CO adsorption on the single Pt atom would effectively promote the aggregation of Pt-n clusters via the Ostwald ripening mechanism.
更多查看译文
AI 理解论文
溯源树
样例
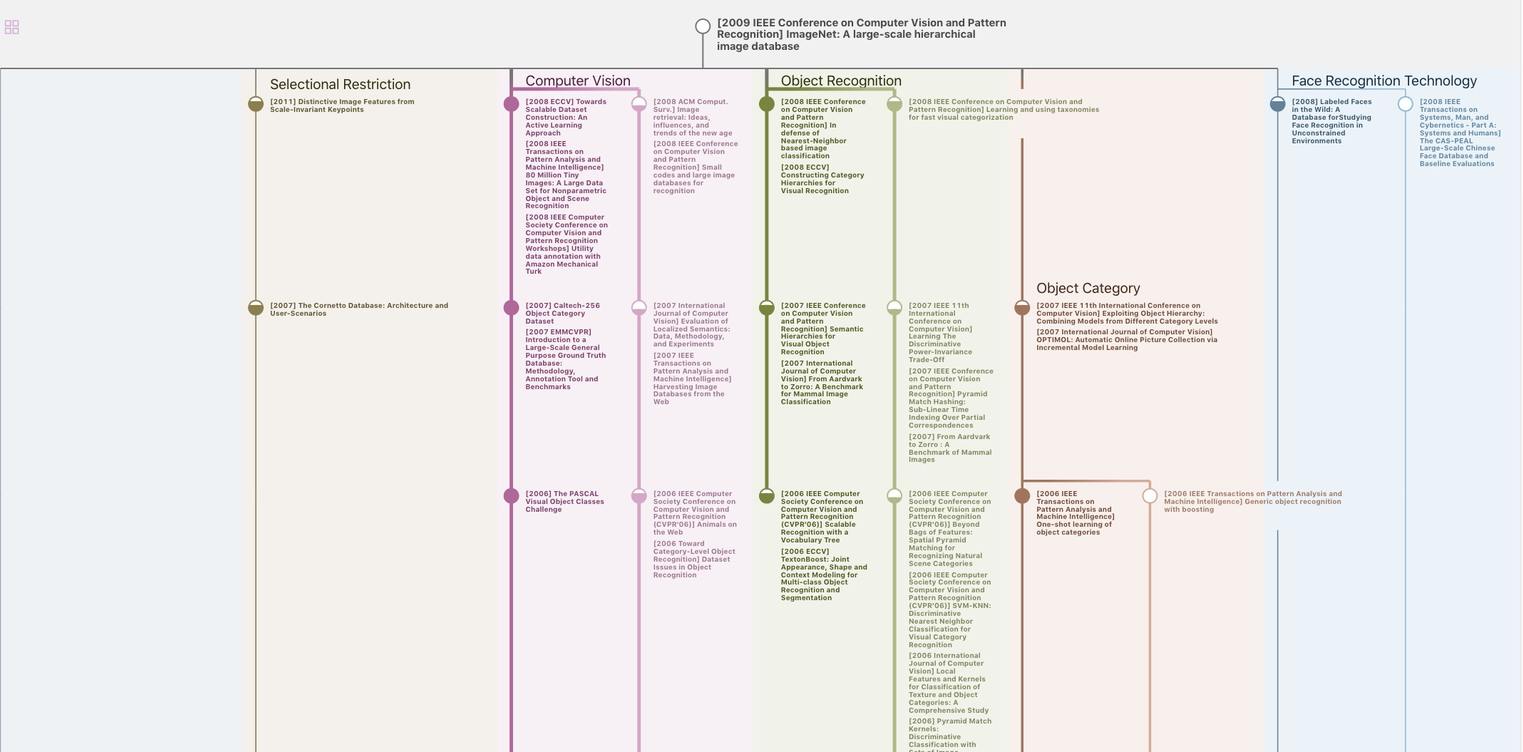
生成溯源树,研究论文发展脉络
Chat Paper
正在生成论文摘要