Fault Diagnosis and Reconstruction of Wind Turbine Anemometer Based on RWSSA-AANN
ENERGIES(2021)
摘要
When the state of the wind turbine sensors, especially the anemometer, appears abnormal it will cause unnecessary wind loss and affect the correctness of other parameters of the whole system. It is very important to build a simple and accurate fault diagnosis model. In this paper, the model has been established based on the Random Walk Improved Sparrow Search Algorithm to optimize auto-associative neural network (RWSSA-AANN), and is used for fault diagnosis of wind turbine group anemometers. Using the cluster analysis, six wind turbines are determined to be used as a wind turbine group. The 20,000 sets of normal historical data have been used for training and simulating of the model, and the single and multiple fault states of the anemometer are simulated. Using this model to analyze the wind speed supervisory control and data acquisition system (SCADA) data of six wind turbines in a wind farm from 2013 to 2017, can effectively diagnose the fault state and reconstruct the fault data. A comparison of the results obtained using the model developed in this work has also been made with the corresponding results generated using AANN without optimization and AANN optimized by genetic algorithm. The comparison results indicate that the model has a higher accuracy and detection rate than AANN, genetic algorithm auto-associative neural network (GA-AANN), and principal component analysis (PCA).
更多查看译文
关键词
auto-associative neural network, sparrow search algorithm, anemometer, fault diagnosis, cluster analysis
AI 理解论文
溯源树
样例
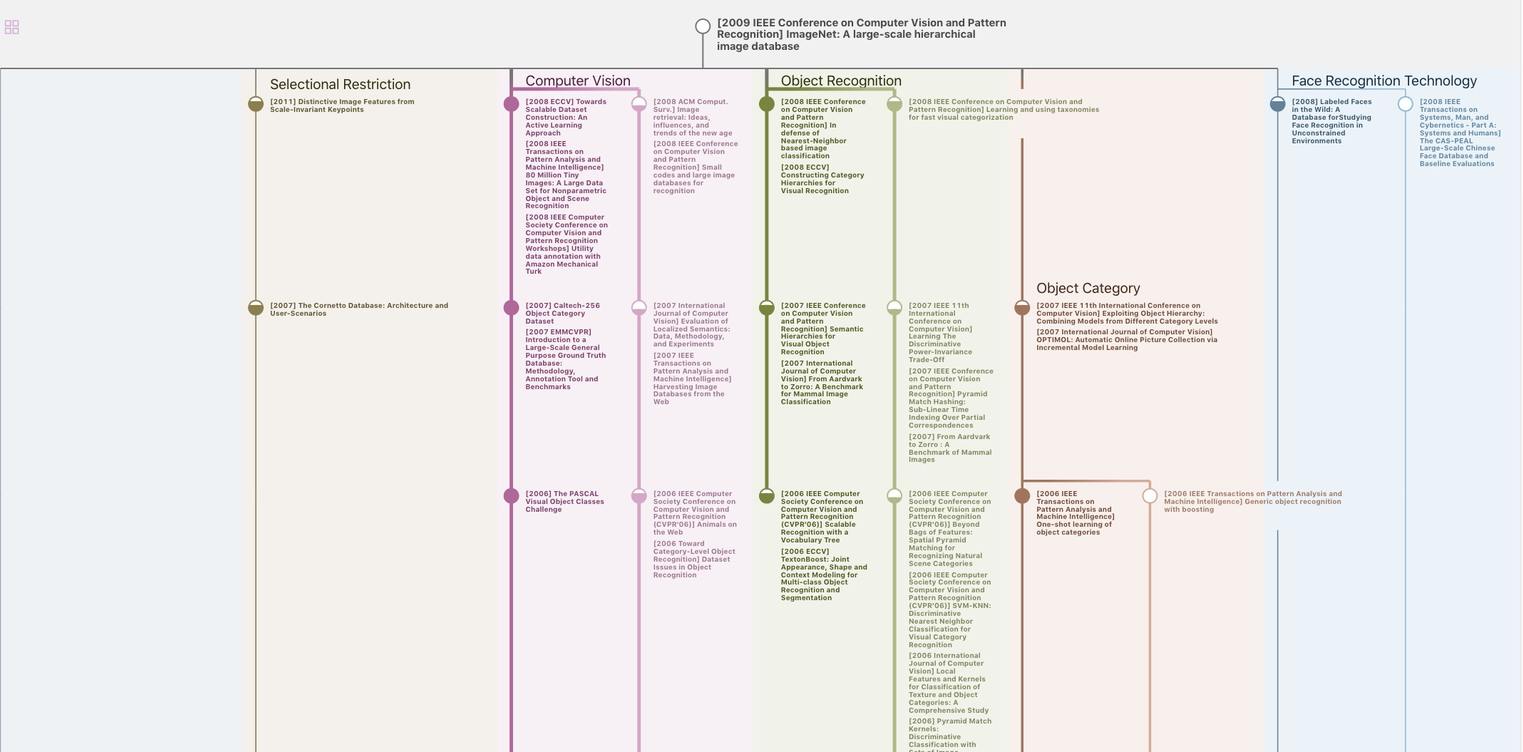
生成溯源树,研究论文发展脉络
Chat Paper
正在生成论文摘要