Prediction of human cytochrome P450 inhibition using bio-selectivity induced deep neural network
BULLETIN OF THE KOREAN CHEMICAL SOCIETY(2022)
摘要
Since the successful debut of AlphaGo, deep learning (DL) techniques have been applied to almost all areas of data sciences and are achieving remarkable milestones. For example, in predicting cytochrome P450 (CYP450) inhibition, DL and other machine learning techniques applied were proven significantly useful. However, currently, most models are focused on how much they can improve compared to previously published methods by using different methodologies and larger data sets without considering bio-selectivity. This study provides a multitask classification model for five important isoforms of CYP450. Our model uses the most extensive data set of over 150 000 molecules ever used. To consider the stereoisomerism of bio-selectivity, new descriptors have been developed. We illustrated the discriminating power of the new descriptors with some examples that demonstrated the improvement in the model's performance using three-dimensional descriptors, which account for the stereoselectivity of drug-protein/enzymes that two-dimensional models cannot achieve.
更多查看译文
关键词
3D descriptors, bio-selectivity, convolution neural networks, CYP450 inhibition, multitask classification model
AI 理解论文
溯源树
样例
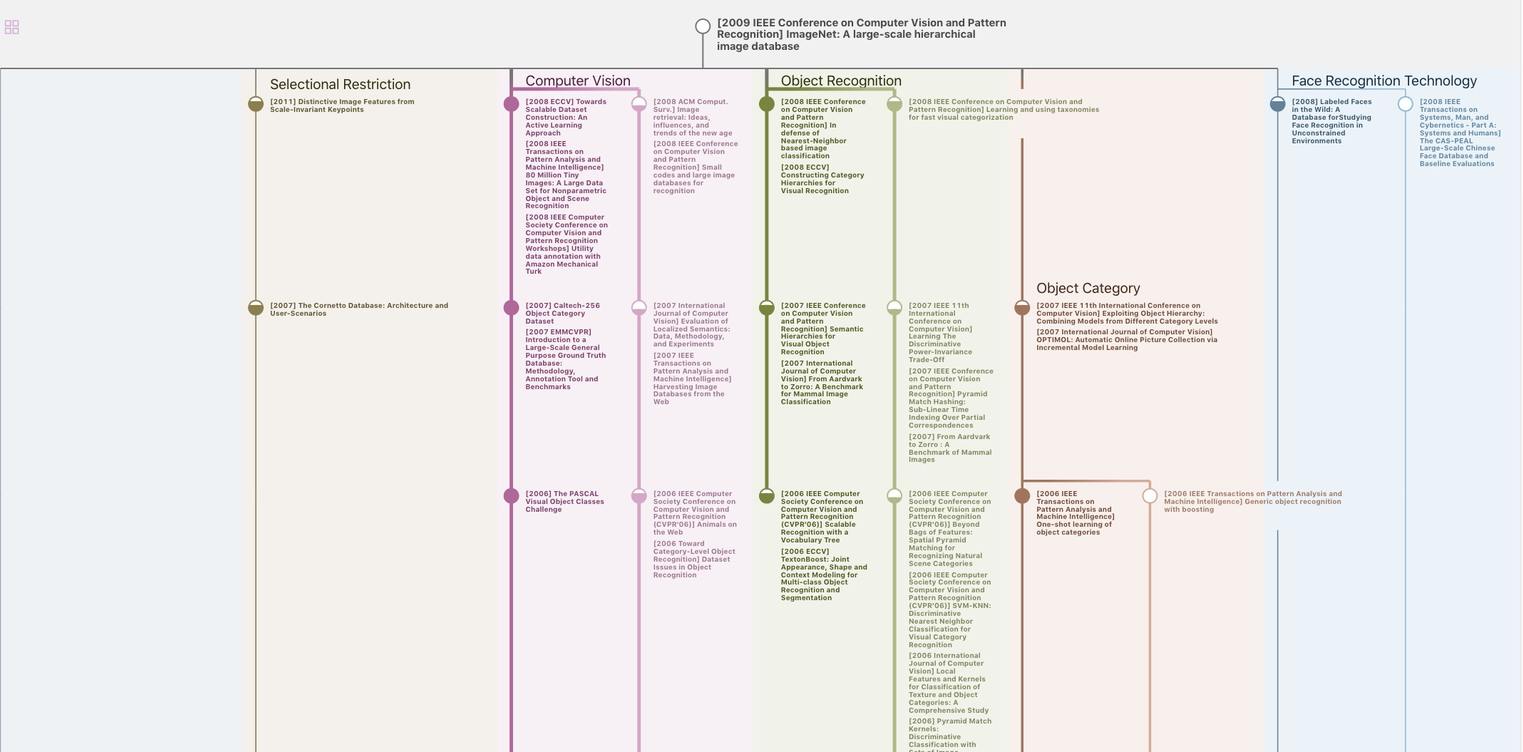
生成溯源树,研究论文发展脉络
Chat Paper
正在生成论文摘要