Forecasting natural gas price trends using random forest and support vector machine classifiers
JOURNAL OF ENERGY MARKETS(2021)
摘要
Price forecasting using statistical modeling methods and data mining has been a topic of great interest among data scientists around the world. In this paper, different machine learning approaches are applied to forecasting future yearly price trends in the natural gas Title Transfer Facility market in the Netherlands. The study compares two models: random forest and support vector classifiers. The identification of potential natural gas price drivers that improve the model's classification is crucial. The forecast horizon was set in a range from 10 to 60 trading days, considering that shorter time horizons have greater importance for trading. The results reflect values up to 85% of the area-under-the-curve score as a reaction of the models to the four different feature combinations used. This invites continued research on the multiple opportunities that these new technologies could create.
更多查看译文
关键词
natural gas market, Title Transfer Facility (TTF), random forest (RF), support vector machine (SVM), energy economics, machine learning
AI 理解论文
溯源树
样例
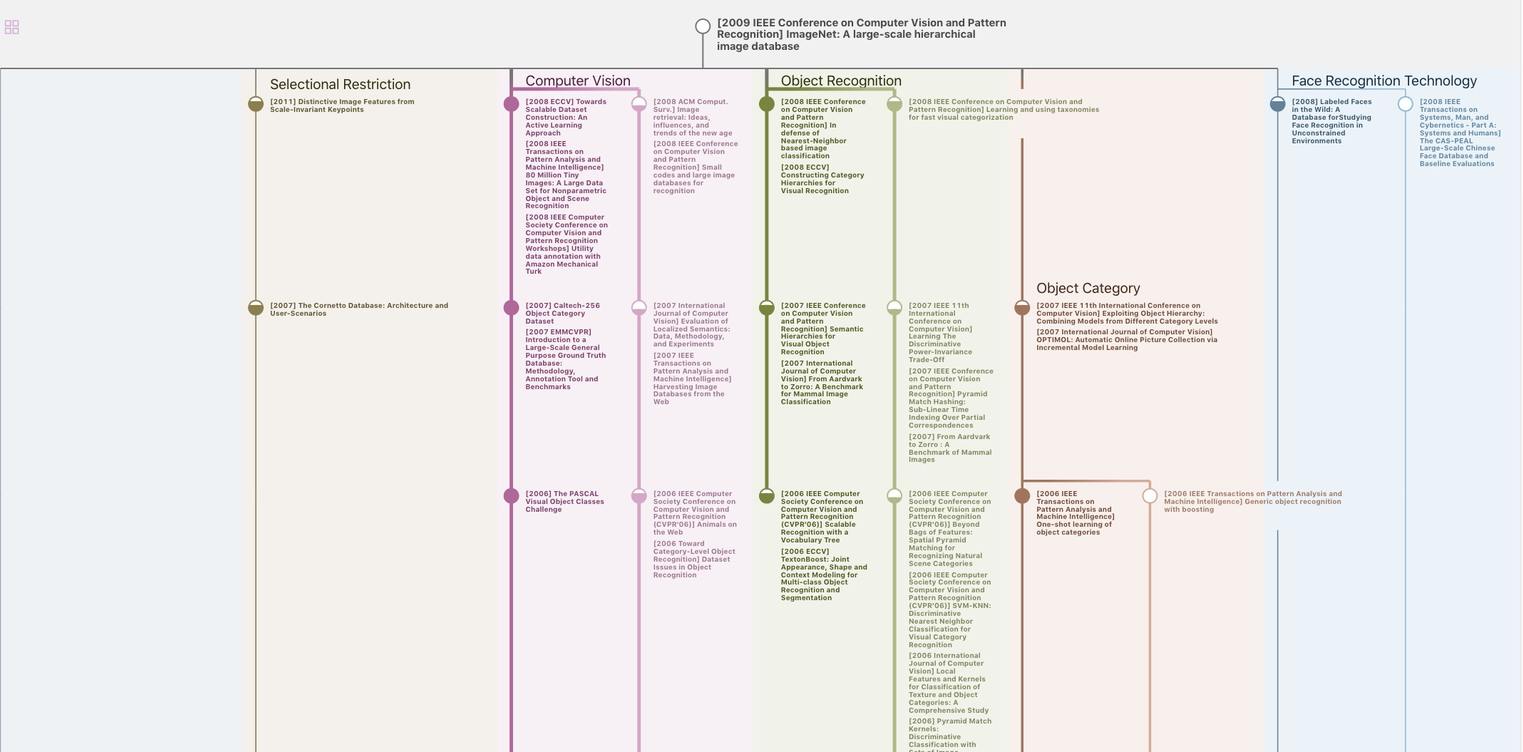
生成溯源树,研究论文发展脉络
Chat Paper
正在生成论文摘要