Polarity-based graph neural network for sign prediction in signed bipartite graphs
WORLD WIDE WEB-INTERNET AND WEB INFORMATION SYSTEMS(2022)
摘要
As a fundamental data structure, graphs are ubiquitous in various applications. Among all types of graphs, signed bipartite graphs contain complex structures with positive and negative links as well as bipartite settings, on which conventional graph analysis algorithms are no longer applicable. Previous works mainly focus on unipartite signed graphs or unsigned bipartite graphs separately. Several models are proposed for applications on the signed bipartite graphs by utilizing the heuristic structural information. However, these methods have limited capability to fully capture the information hidden in such graphs. In this paper, we propose the first graph neural network on signed bipartite graphs, namely Polarity-based Graph Convolutional Network (PbGCN), for sign prediction task with the help of balance theory. We introduce the novel polarity attribute to signed bipartite graphs, based on which we construct one-mode projection graphs to allow the GNNs to aggregate information between the same type nodes. Extensive experiments on five datasets demonstrate the effectiveness of our proposed techniques.
更多查看译文
关键词
Graph neural network,Signed bipartite graph,Sign prediction
AI 理解论文
溯源树
样例
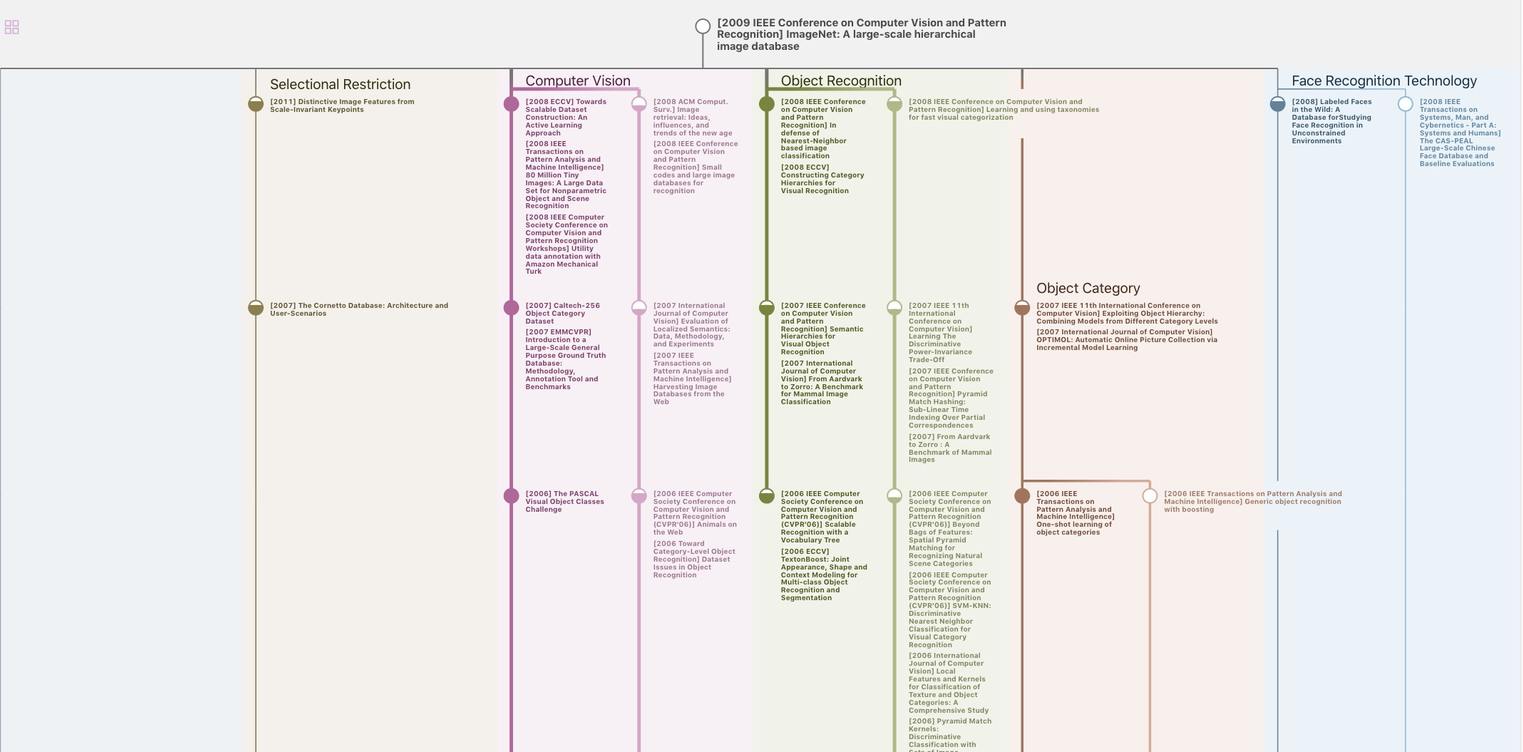
生成溯源树,研究论文发展脉络
Chat Paper
正在生成论文摘要