DRDDN: dense residual and dilated dehazing network
VISUAL COMPUTER(2022)
摘要
Recently, deep convolutional neural networks (CNNs) have made great achievements in image restoration. However, there exists a large space to improve the performance of CNN-based dehazing model. In this paper, we design a fully end-to-end dehazing network, which can be called as dense residual and dilated dehazing network (DRDDN), for single image dehazing. In detail, a dilated densely connected block is designed to fully exploit multi-scale features through an adaptive learning process. The receptive field of the network is enlarged by dilation convolution without losing spatial information. Furthermore, we use deep residual to propagate the low-level features to high-level layers. Therefore, our model can fully exploit both low-level and high-level features for dehazing. Experiments on benchmark datasets and real-world hazy images show that the proposed DRDDN achieves favorable performance against the state-of-the-art methods.
更多查看译文
关键词
Dense residual learning, Dehazing, Dilated densely connected block
AI 理解论文
溯源树
样例
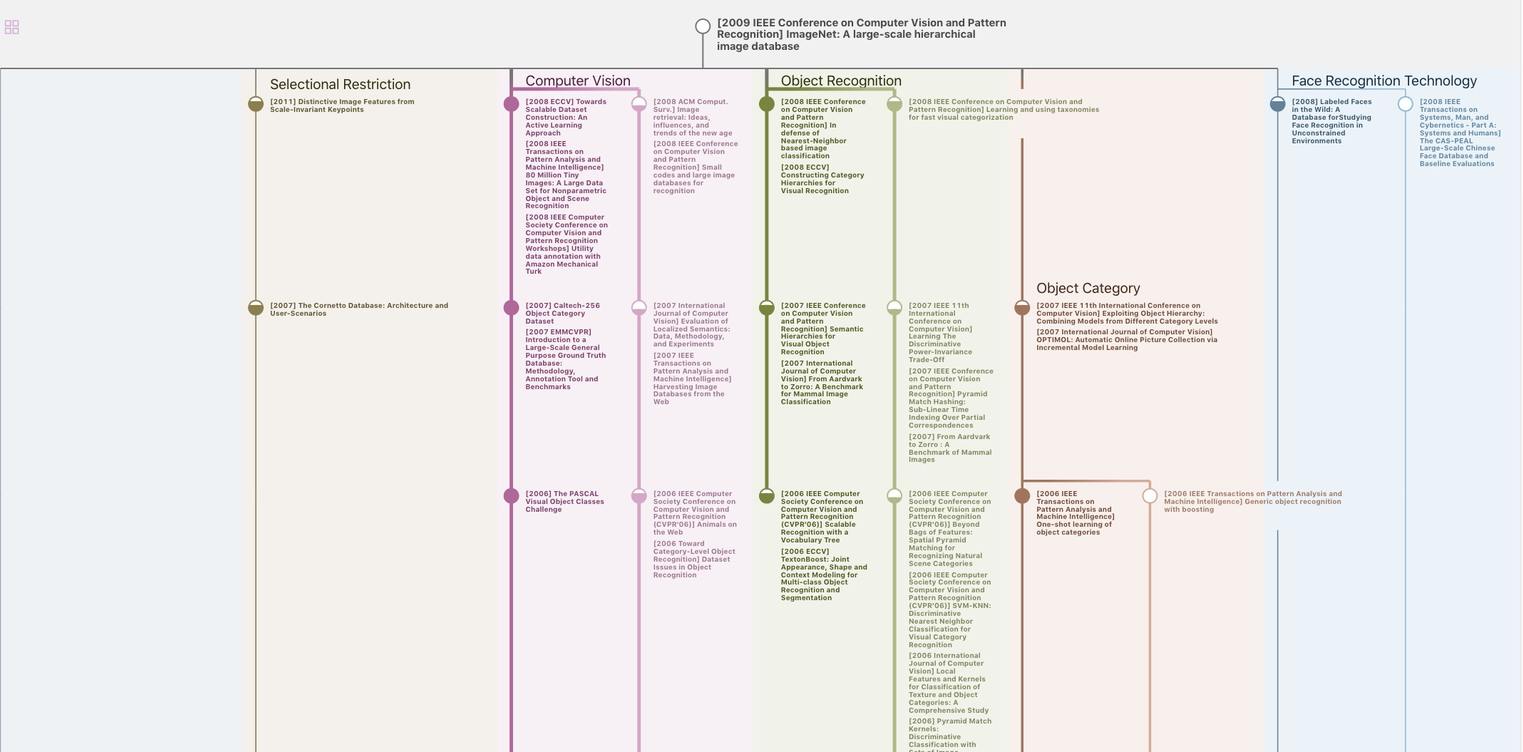
生成溯源树,研究论文发展脉络
Chat Paper
正在生成论文摘要