Feature engineering for machine learning enabled early prediction of battery lifetime
Journal of Power Sources(2022)
摘要
Accurate battery lifetime estimates enable accelerated design of novel battery materials and determination of optimal use protocols for longevity in deployments. Unfortunately, traditional battery testing may take years to reach thousands of cycles. Recent studies have shown that machine learning (ML) tools can predict lithium-ion battery lifetimes from 100 or fewer preliminary cycles, representing only a few weeks of cycling. Until now, conclusions about the efficacy and broad applicability of these predictions across a variety of cathode chemistries have been limited by available experimental information. In this work, we leverage a battery cycling dataset representing six cathode chemistries (NMC111, NMC532, NMC622, NMC811, HE5050, and 5Vspinel), multiple electrolyte/anode compositions, and 300 total carefully prepared pouch batteries to explore feature selection and battery chemistry's role in ML battery lifetime predictions. A mean absolute error (MAE) of 78 cycles in prediction was seen for a chemistry-spanning test set from 100 preliminary cycles. Furthermore, an MAE of 103 cycles was seen when using only the first cycle. This study represents an in-depth investigation of strategies for feature selection for battery lifetime prediction, ML models' generalization across multiple battery chemistries, and predictions beyond the training set in the chemical space.
更多查看译文
关键词
Lithium-ion,Battery,Life prediction,State of health,Machine learning,Feature engineering
AI 理解论文
溯源树
样例
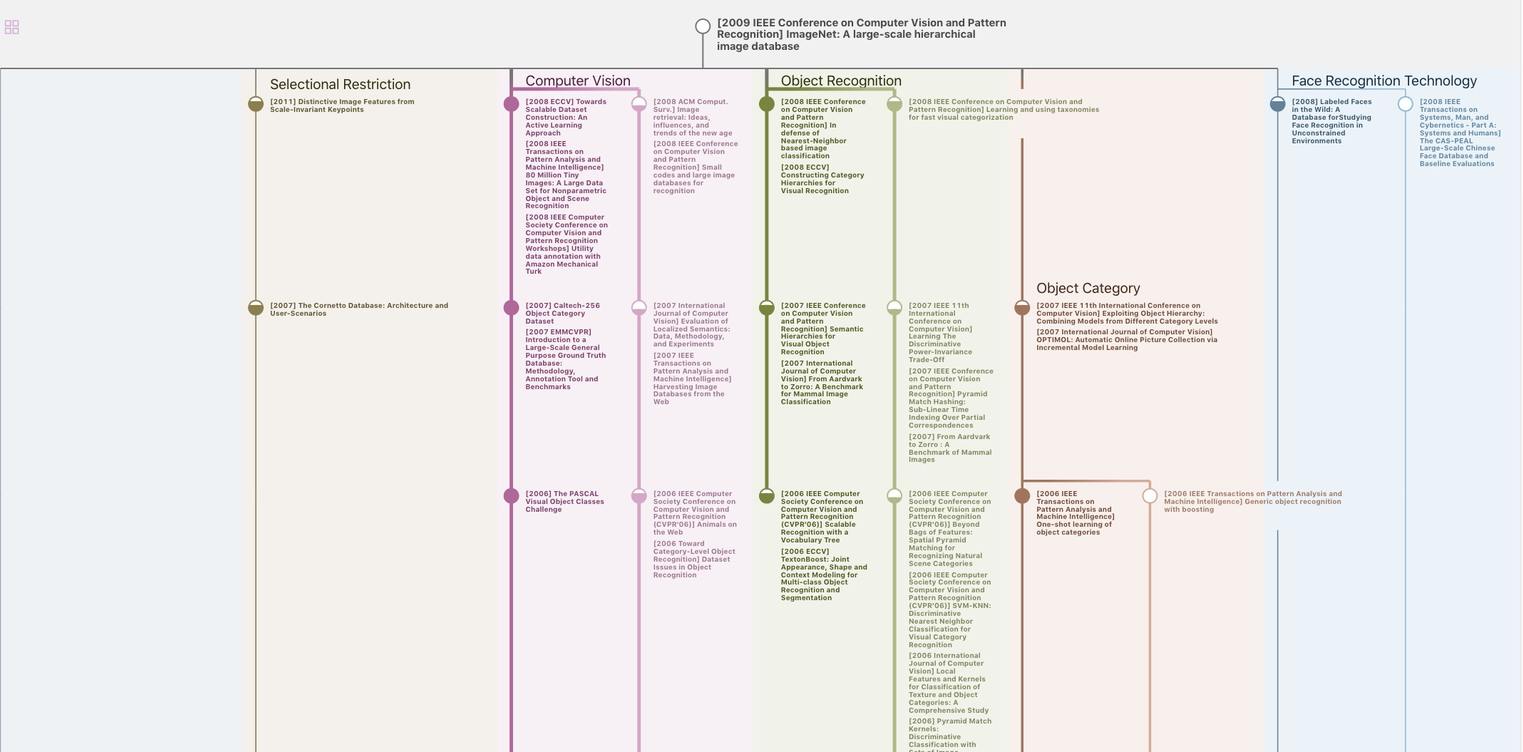
生成溯源树,研究论文发展脉络
Chat Paper
正在生成论文摘要