Trace-to-Trace Translation for SCA
SMART CARD RESEARCH AND ADVANCED APPLICATIONS (CARDIS 2021)(2022)
摘要
Neural Networks (NN) have been built to solve universal function approximation problems. Some architectures as Convolutional Neural Networks (CNN) are dedicated to classification in the context of image distortion. They have naturally been considered in the community to perform side-channel attacks showing good results on traces exposing time misalignment. However, even where these timing distortions are not present, NN have produced better results than legacy attacks. Recently in TCHES 2020, auto-encoders have been used as preprocessing for noise reduction. The main idea is to train an auto-encoder using as inputs noisy traces and less noisy traces so that the auto-encoder is able to remove part of the noise in the attack dataset. We propose to extend this idea of using NN for pre-processing by not only considering the noise-reduction but to translate data between two side-channel domains. In a nutshell, clean (or less noisy) traces may not be available to an attacker, but similar traces that are easier to attack may be obtainable. Availability of such traces can be leveraged to learn how to translate difficult traces to easy ones to increase attackability.
更多查看译文
关键词
Side-channel analysis, Generative Adversarial Network, Profiled attacks, Neural networks, Electromagnetic emanations, Power consumption
AI 理解论文
溯源树
样例
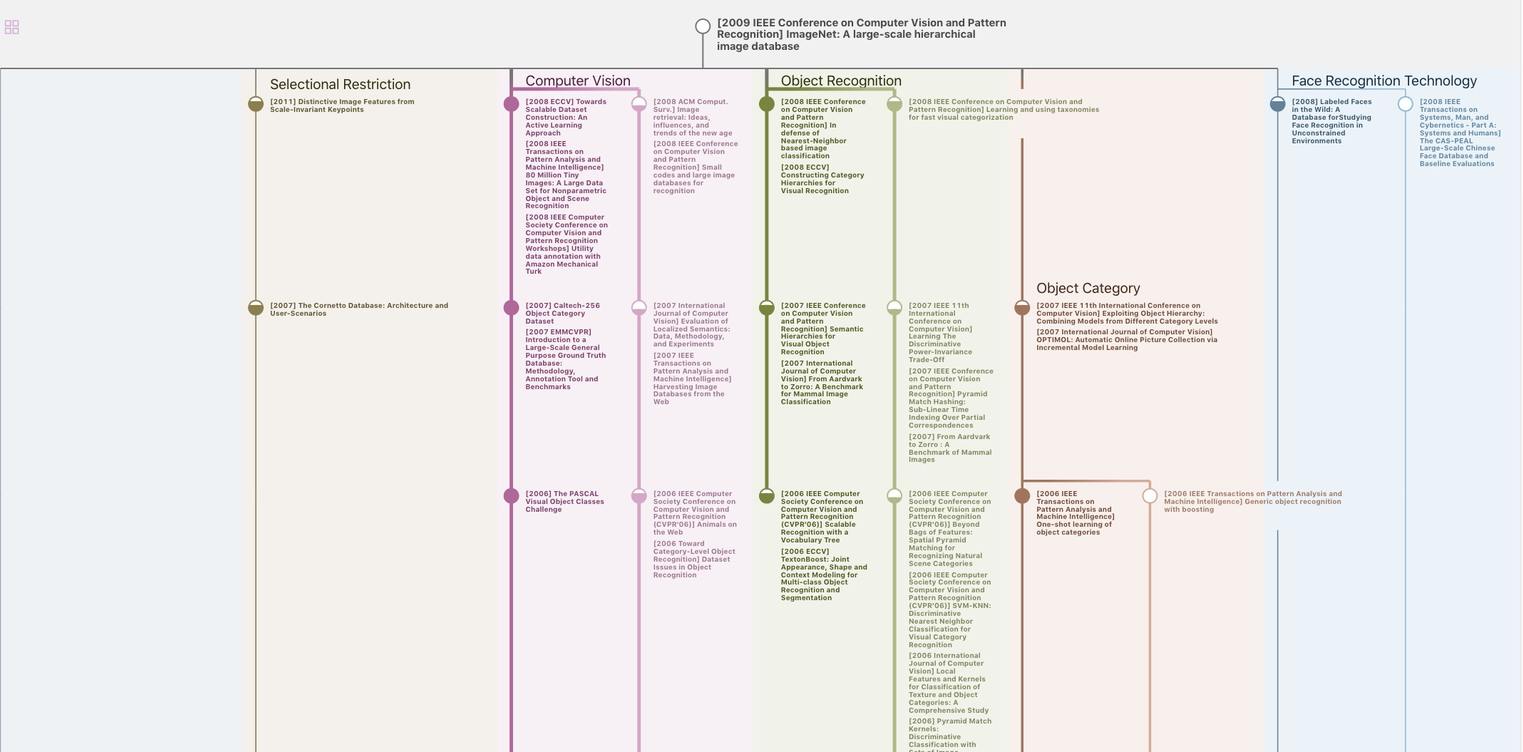
生成溯源树,研究论文发展脉络
Chat Paper
正在生成论文摘要