Optimization-Based Hyperspectral Spatiotemporal Super-Resolution
IEEE ACCESS(2022)
摘要
Due to hardware limitations and financial considerations, it is challenging to acquire fine spatial and temporal resolution (FSFT) images, which leads to the interest in spatiotemporal fusion problems. Instead of directly obtaining costly FSFT images, an alternative is to fuse fine spatial, coarse temporal resolution (FSCT) images with coarse spatial, fine temporal resolution (CSFT) images. Unlike existing spatiotemporal methods which are only designed for multispectral images, this paper first proposes a new fusion framework for hyperspectral spatiotemporal super-resolution, termed HSTSR. In this paper, we first deal with the coarse temporal resolution issue by adopting the fast iterative shrinkage-thresholding algorithm (FISTA) to estimate the missing images at the intermediate time series. Then, we fuse the hyperspectral image and the multispectral image in each time series via coupled nonnegative matrix factorization (CNMF) to get FSFT hyperspectral images. Importantly, we can automatically estimate the associated spatial blurring and spectral downsampling matrices without prior satellite hardware information. Compared with other extended multispectral spatiotemporal methods, our method not only attains satisfying qualities significantly faster, but also requires much less input data.
更多查看译文
关键词
Spatial resolution, Spatiotemporal phenomena, Satellites, Hyperspectral imaging, Superresolution, Sensor fusion, Image sensors, Gradient descent, ADMM, FISTA, convex optimization, hyperspectral, multispectral, spatiotemporal, super-resolution, image fusion
AI 理解论文
溯源树
样例
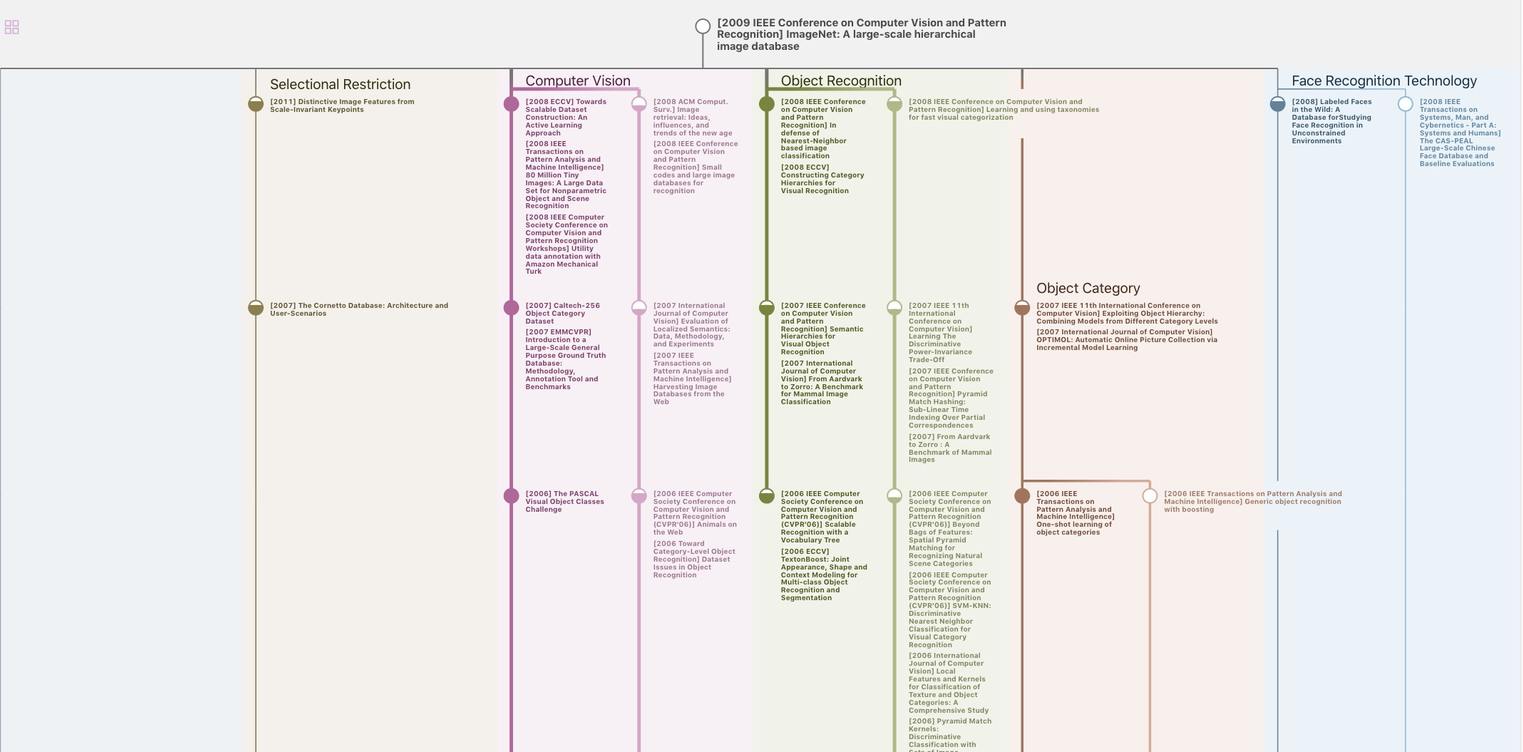
生成溯源树,研究论文发展脉络
Chat Paper
正在生成论文摘要