Reliable metrics-based linear regression model for multilevel privacy measurement of face instances
IET IMAGE PROCESSING(2022)
摘要
Social networking sites have made photo sharing convenient and, consequently, users of those sites frequently share photos. The proliferation of these social images throughout the Internet has inadvertently exposed an increasing number of personally identifiable information, particularly from the visual information of the faces in the images. Most existing methods to de-identify faces results in an excessive loss of visual information. To solve this problem, this paper proposes a reliable metrics-based linear regression model for multilevel privacy measurement of face instances using the size information of face instances. The proposed privacy measurement model provides a novel instance-level-based solution to measure privacy levels. The paper also establishes a scientific relationship between the size information of face instances and their privacy levels, quantifying the degree to which face instances need to be de-identified. Finally, the paper proposes a novel k-Same-DT de-identification method to provide reliable metrics for a linear regression model. It is a real-time k-same-related de-identification algorithm that combines the PCA dimensionality reduction strategy and the Delaunay triangle-based face alignment algorithm.The proposed k-Same-DT method creates a de-identified face with a lower identifiable rate and higher structural similarity, and it can provide reliable de-identification metrics for privacy measurement. Experiments using the classical face dataset demonstrates the effectiveness of the proposed de-identification method. Extensive experiments and surveys on real-world social images were also conducted to verify the proposed measurement model.
更多查看译文
AI 理解论文
溯源树
样例
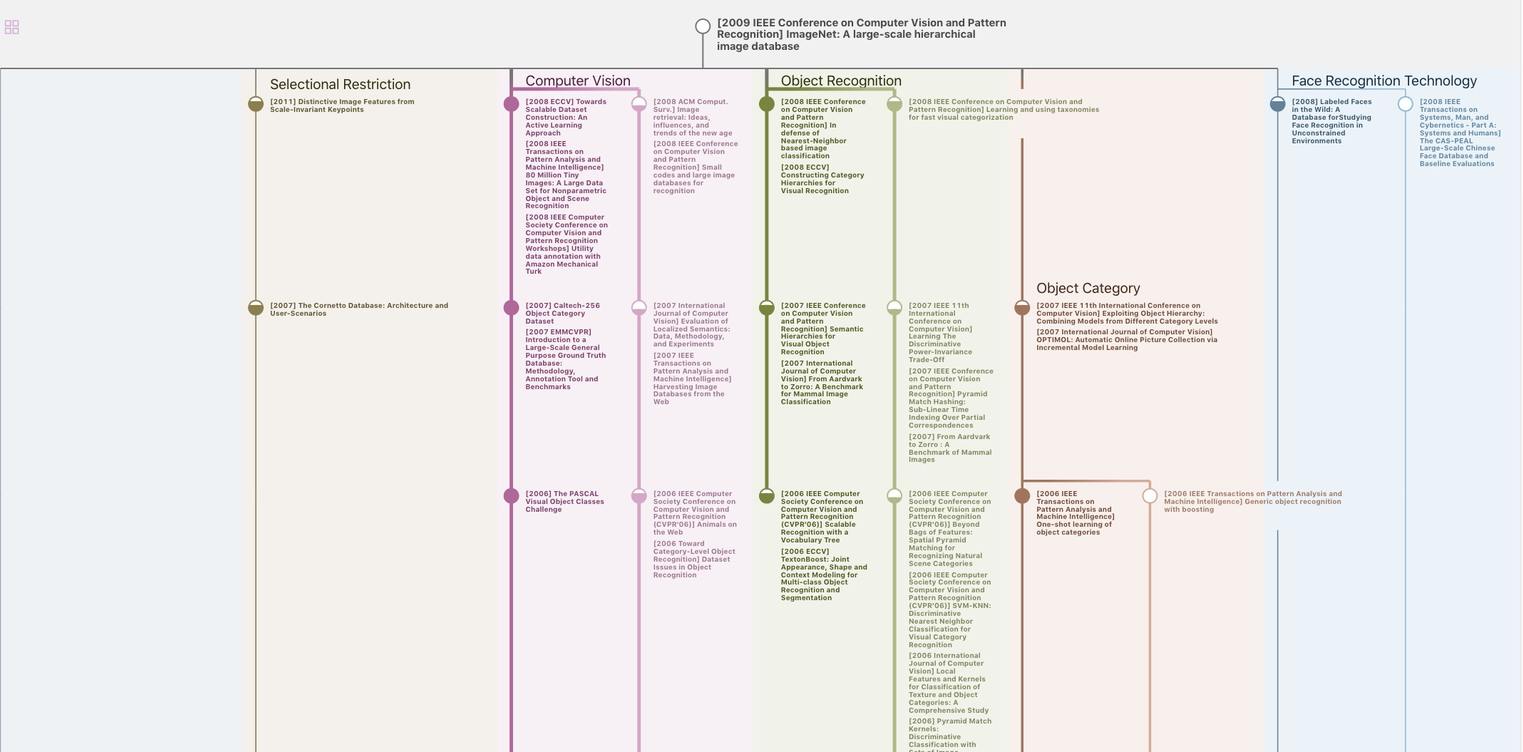
生成溯源树,研究论文发展脉络
Chat Paper
正在生成论文摘要