Evaluation and Prediction of Column Aerosol by Using the Time Series Machine Learning Technique
JOURNAL OF KOREAN SOCIETY FOR ATMOSPHERIC ENVIRONMENT(2022)
摘要
Column aerosol observation has the advantage of obtaining microphysicai information of aerosols present in the vertical atmospheric column at a given point. In this study, in order to predict column aerosol loads in the local atmosphere, time series machine learning technique was applied by using Aerosol Optical Depth (AOD) and Angstrom Exponent (AE) data acquired from the selected ground-based Sun-sky radiometer observation network. For the determination of the best time series machine learning model, the independent optional properties including three regression models (glmnet, Im, and spark), four training period (1-4 years), and five regularization parameters (0-0.08) were tested in time series modelling. The results showed that spark model with the 1 year training period and regularization constant of 0.08 has the highest accuracy (RMSE=0.302, bias=0.213) for the AOD prediction. In the case of AE prediction, the highest accuracy (RMSE=0.356, bias=0.238) was obtained by the glmnet model with 1 year training period and the regularization constant of 0.08. In addition, machine learning clustering results shows that urban/industrial aerosol types occurred at a rate of 63.7% in Korea. The methodology and results of this study can be used for short-term aerosol prediction models and remote sensing data.
更多查看译文
关键词
Aerosol, Machine learning, Aerosol optical depth, Classification, Air quality
AI 理解论文
溯源树
样例
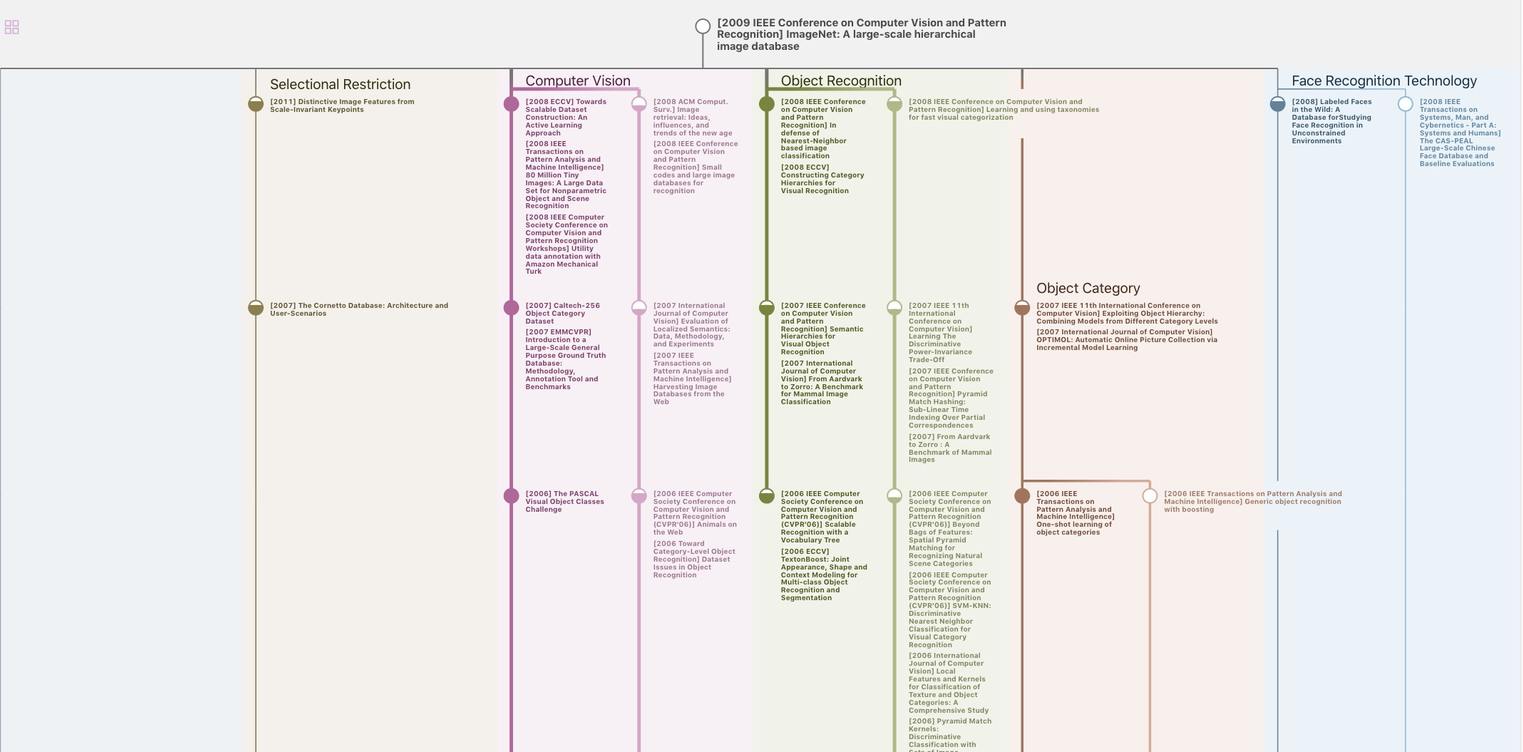
生成溯源树,研究论文发展脉络
Chat Paper
正在生成论文摘要