The data sampling effect on financial distress prediction by single and ensemble learning techniques
COMMUNICATIONS IN STATISTICS-THEORY AND METHODS(2023)
摘要
Financial distress domain problem datasets are usually class imbalanced. In literature, data sampling is one of the widely used solutions to deal with the class imbalance problem. This article focuses on examining the data sampling effect on financial distress prediction models by single and ensemble learning techniques. The experimental datasets are based on three bankruptcy prediction and credit scoring datasets and twelve different single classifiers and classifier ensembles are constructed. We find that although some prediction models trained by the original class imbalanced datasets provide reasonable AUC, their type II errors are very high for the practical usage. However, when data sampling is performed over the datasets, all of the prediction models can slightly increase their AUC and largely reduce their type II errors. More specifically, the decision tree ensembles by bagging and boosting methods are the better choices for financial distress prediction.
更多查看译文
关键词
Data mining, data sampling, financial distress prediction, machine learning, class imbalance
AI 理解论文
溯源树
样例
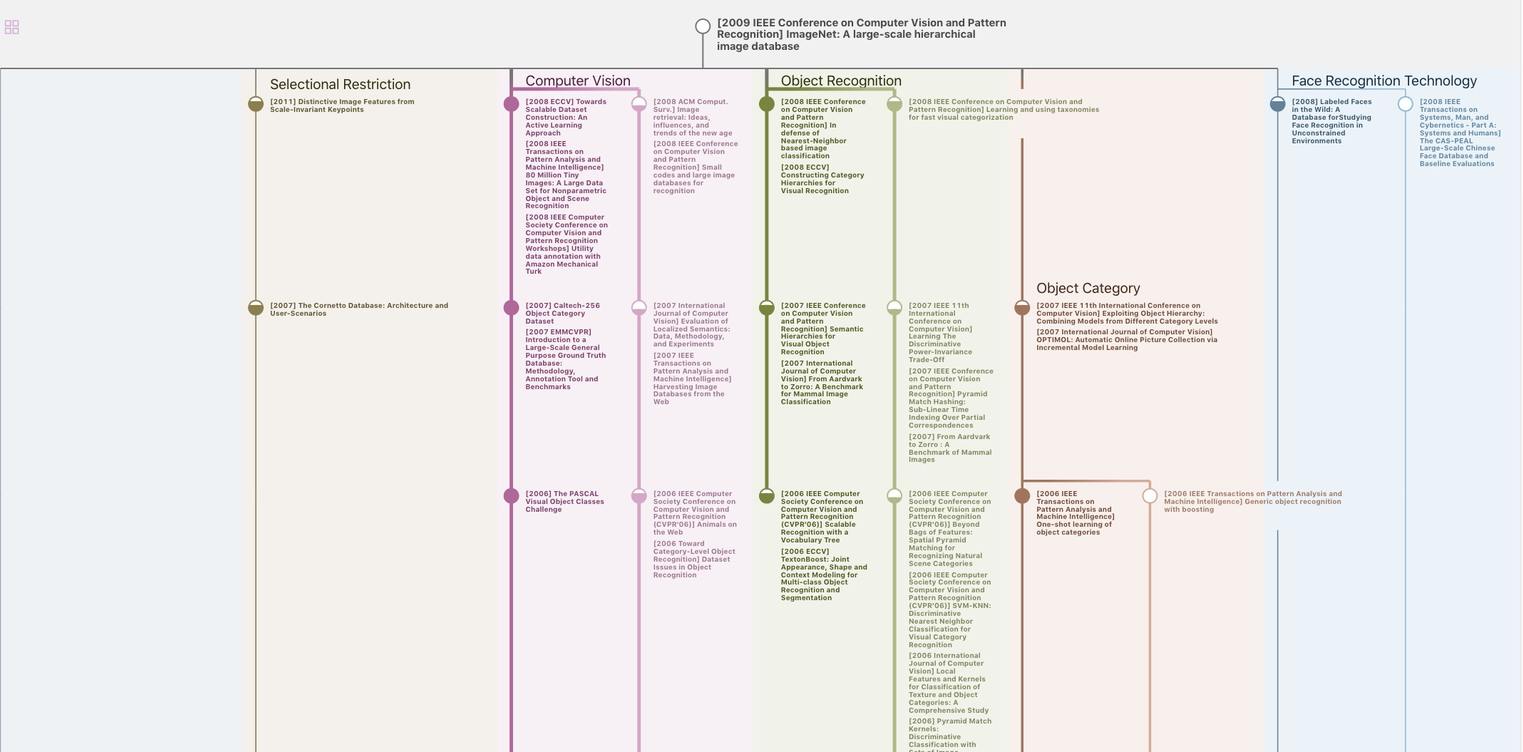
生成溯源树,研究论文发展脉络
Chat Paper
正在生成论文摘要