Machine learning approach to improve vapor recovery: Prediction and frequency converter with a new vapor recovery system
PROCEEDINGS OF THE INSTITUTION OF MECHANICAL ENGINEERS PART C-JOURNAL OF MECHANICAL ENGINEERING SCIENCE(2022)
摘要
In practice, the volatile organic compounds (VOCs) pollution can exist when refueling due to the properties of the gasoline, low viscosity and high saturated-vapor pressure. A new gasoline vapor recovery system involving frequency conversion technology and machine learning is developed to cope with this problem. In the proposed system, firstly, the pumping capacity of the vacuum pump is evaluated, and test shows an almost linear relationship between suction volume and frequency. Then, the Multi-Layer Perception (MLP) neural network and the support vector regression (SVR) are employed to predict the gas-liquid ratio, and the numerical examples are presented to prove the high prediction accuracy of the MLP and SVR, respectively, where the MLP neural network has better generalization ability. Finally, compared with the two gasoline vapor recovery systems based on the 1: 1 fixed control model and the PID control model, respectively, the gasoline vapor recovery efficiency is improved significantly by the new gasoline vapor recovery system.
更多查看译文
关键词
Volatile organic compounds, gasoline vapor recovery, frequency conversion technology, gas-liquid ratio, machine learning
AI 理解论文
溯源树
样例
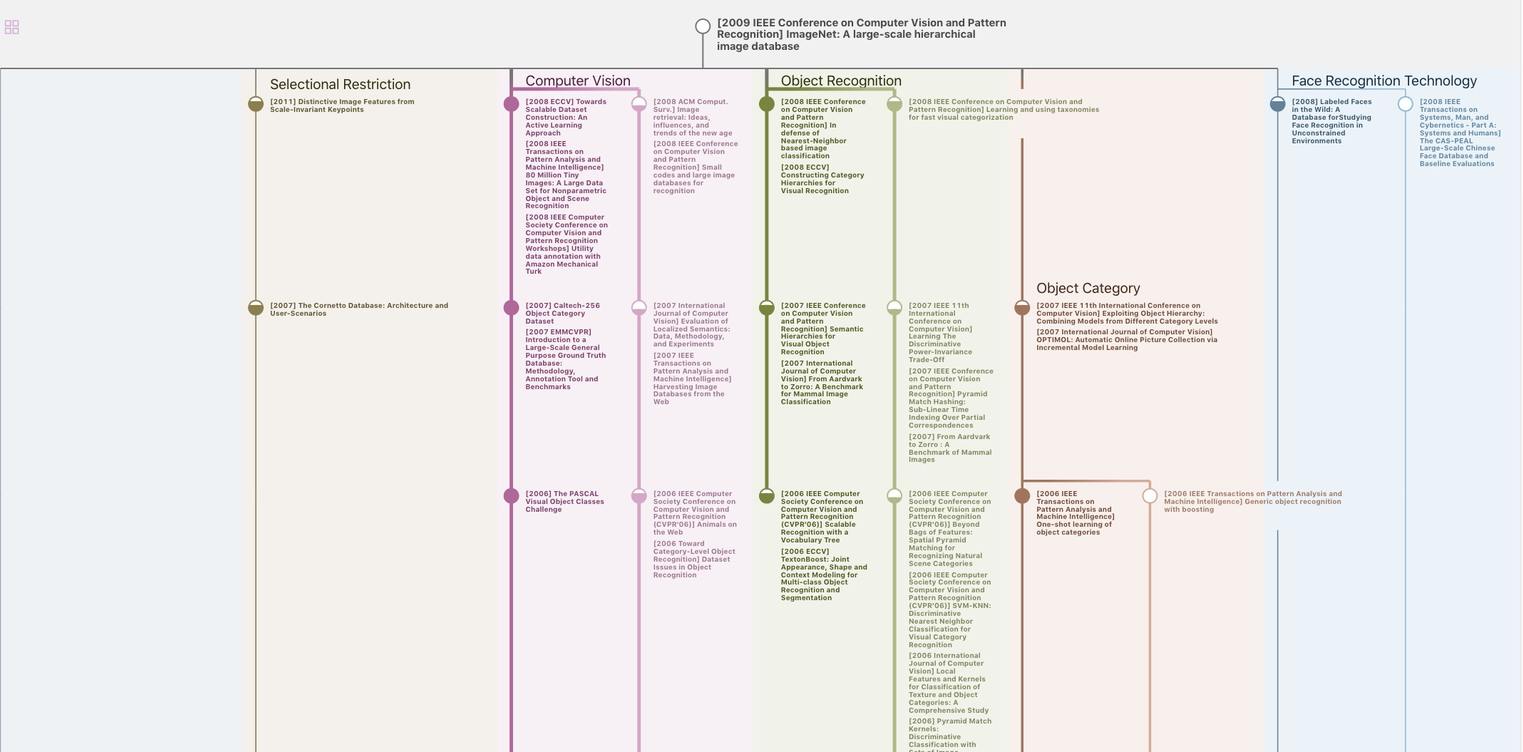
生成溯源树,研究论文发展脉络
Chat Paper
正在生成论文摘要