Stock market movement prediction using evolving spiking neural networks
HANDBOOK OF BIG DATA ANALYTICS, VOL. 2: Applications in ICT, Security and Business Analytics(2021)
摘要
Stock price movement direction prediction is regarded as one of the most difficult and challenging tasks in the real world. An accurate prediction can yield profit to the investors and protect them from financial risk. In this study, we propose three variants of the evolving spiking neural networks (eSNNs) for the stock trend prediction: eSNN model using technical stock indicators (SIs) as input variables - SI-eSNN; a parallel implementation of the SI-eSNN model on a GPU machine - Compute Unified Device Architecture (CUDA)-SI-eSNN; and finally, a model for incremental learning using a sliding window (SW) of data - SW-eSNN. We also propose logistic distribution in place of Gaussian distribution in characterizing the receptive fields. The models are applied on nine large-scale benchmark stock indices of different countries. We considered classification accuracy and area under ROC curve (AUC) to measure the performance of the models in terms of predicting UP or DOWN movements on their daily prices. Our experimental results show that the eSNN model and its parallel implementation achieve a high accuracy of 80%-90% of predicting stock movements from 1 day to 1 month ahead forecasts, which is a significant improvement when compared with the use of traditional AI methods. Also, the performance of eSNN model with logistic receptive fields is compared with that of a deep learning architecture: long short-term memory (LSTM) network. The comparative analysis shows that the proposed model outperformed LSTM in the case of four stock indices out of nine in terms of accuracy. The CUDA-SI-eSNN model performs three to five times faster than the sequential eSNN model. The window-based eSNN model, SW-eSNN, achieved around 75% accuracy on average across all indexes. As eSNN can be considered as one specific implementation of a comprehensive SNN architecture called NeuCube, future development of improved models is also discussed. The results are promising for a future development of automated trading systems.
更多查看译文
关键词
stock,prediction,neural networks,movement,market
AI 理解论文
溯源树
样例
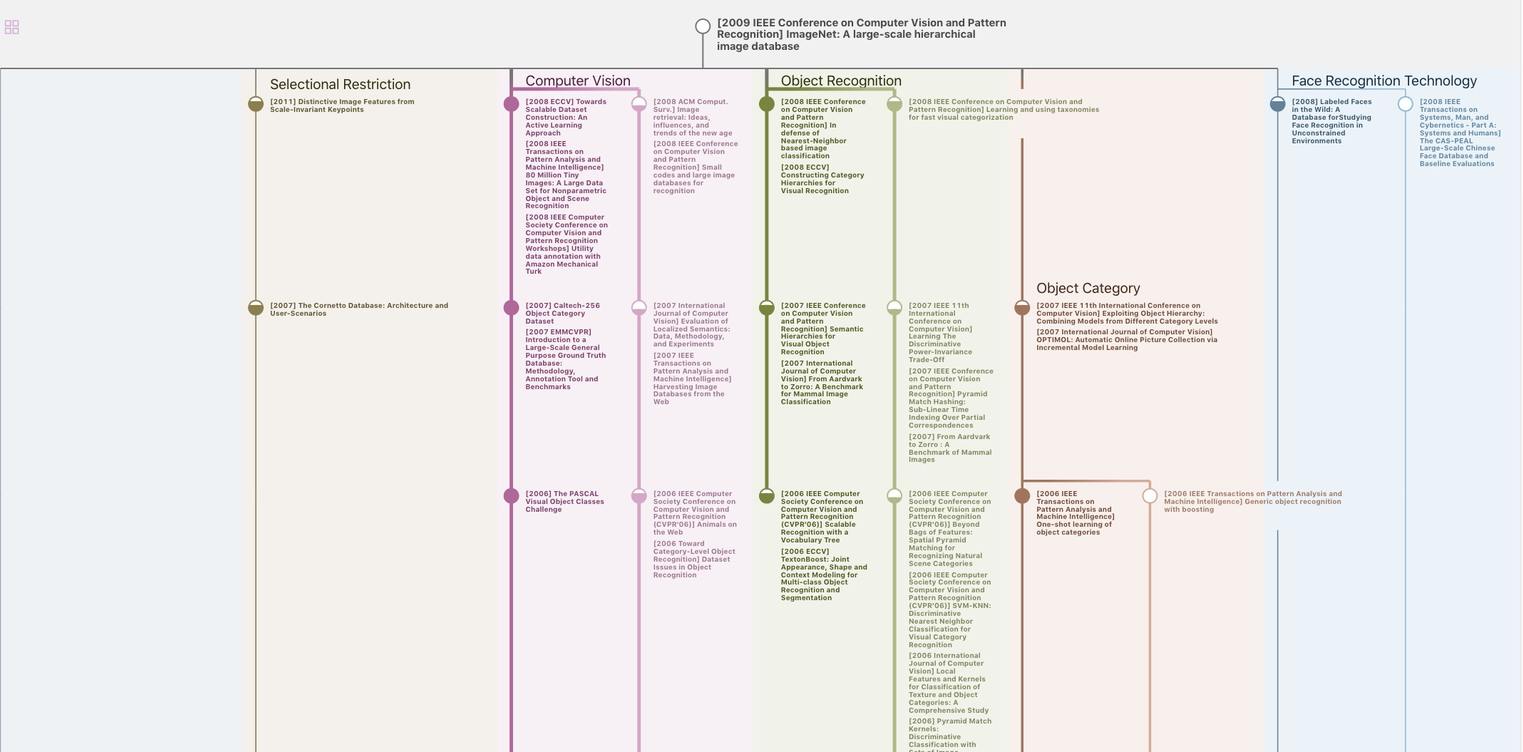
生成溯源树,研究论文发展脉络
Chat Paper
正在生成论文摘要