DDMF: A Method for Mining Relatively Important Nodes Based on Distance Distribution and Multi-Index Fusion
APPLIED SCIENCES-BASEL(2022)
摘要
In research on complex networks, mining relatively important nodes is a challenging and practical work. However, little research has been done on mining relatively important nodes in complex networks, and the existing relatively important node mining algorithms cannot take into account the indicators of both precision and applicability. Aiming at the scarcity of relatively important node mining algorithms and the limitations of existing algorithms, this paper proposes a relatively important node mining method based on distance distribution and multi-index fusion (DDMF). First, the distance distribution of each node is generated according to the shortest path between nodes in the network; then, the cosine similarity, Euclidean distance and relative entropy are fused, and the entropy weight method is used to calculate the weights of different indexes; Finally, by calculating the relative importance score of nodes in the network, the relatively important nodes are mined. Through verification and analysis on real network datasets in different fields, the results show that the DDMF method outperforms other relatively important node mining algorithms in precision, recall, and AUC value.
更多查看译文
关键词
complex network, distance distribution, multi-index fusion, relatively important node
AI 理解论文
溯源树
样例
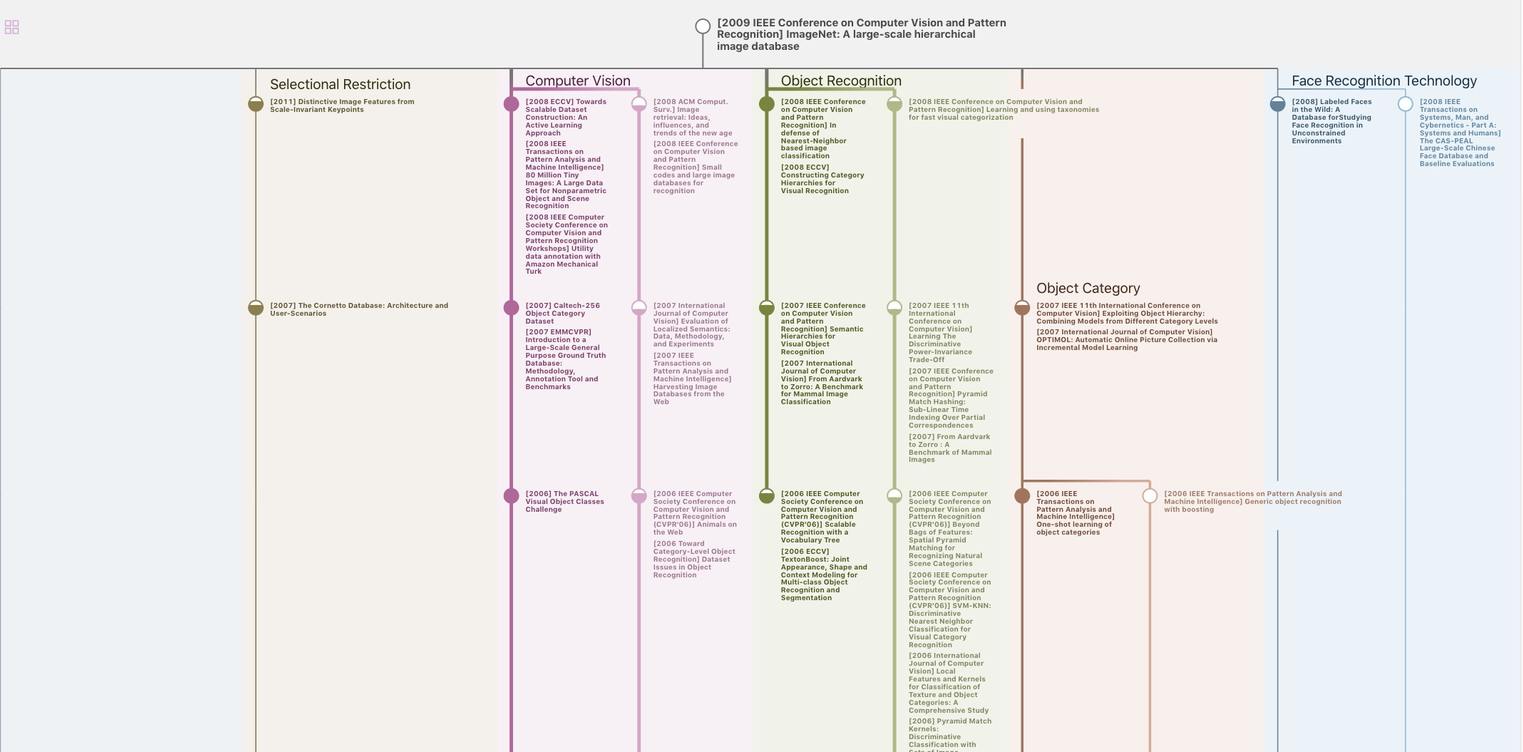
生成溯源树,研究论文发展脉络
Chat Paper
正在生成论文摘要