Image matching based on a structured deep coupled metric learning framework
Signal, Image and Video Processing(2022)
摘要
The general similarity metric method is difficult to calculate the similarity of images under different resolutions, occlusion, and deformation. Therefore, this paper proposes a structured deep coupled metric learning framework (SDCML), which provides a solution for image matching under the above special interference. For the input image pairs, the structured region segmentation is performed to derive global and local sub-region images. After that, a stacked sparse auto-encoder network is used to implement deep feature extraction on these derived images. Then, the features with different dimensions of derived image pairs are transformed to coupled space through multi-supervised coupled mapping, and the similarity metric of feature pairs is completed in this space. Finally, all calculated similarities of derived image pairs are fused to obtain the final matching result. The effectiveness of the proposed method is verified by image matching experiments under non-ideal conditions; the optimal recognition rate was 91.98% on Yale-B dataset and 72.80% on Market1501 dataset, respectively.
更多查看译文
关键词
Structured region segmentation,Deep sparse auto-encoder network,Multi-supervised coupled mapping,Non-ideal image matching
AI 理解论文
溯源树
样例
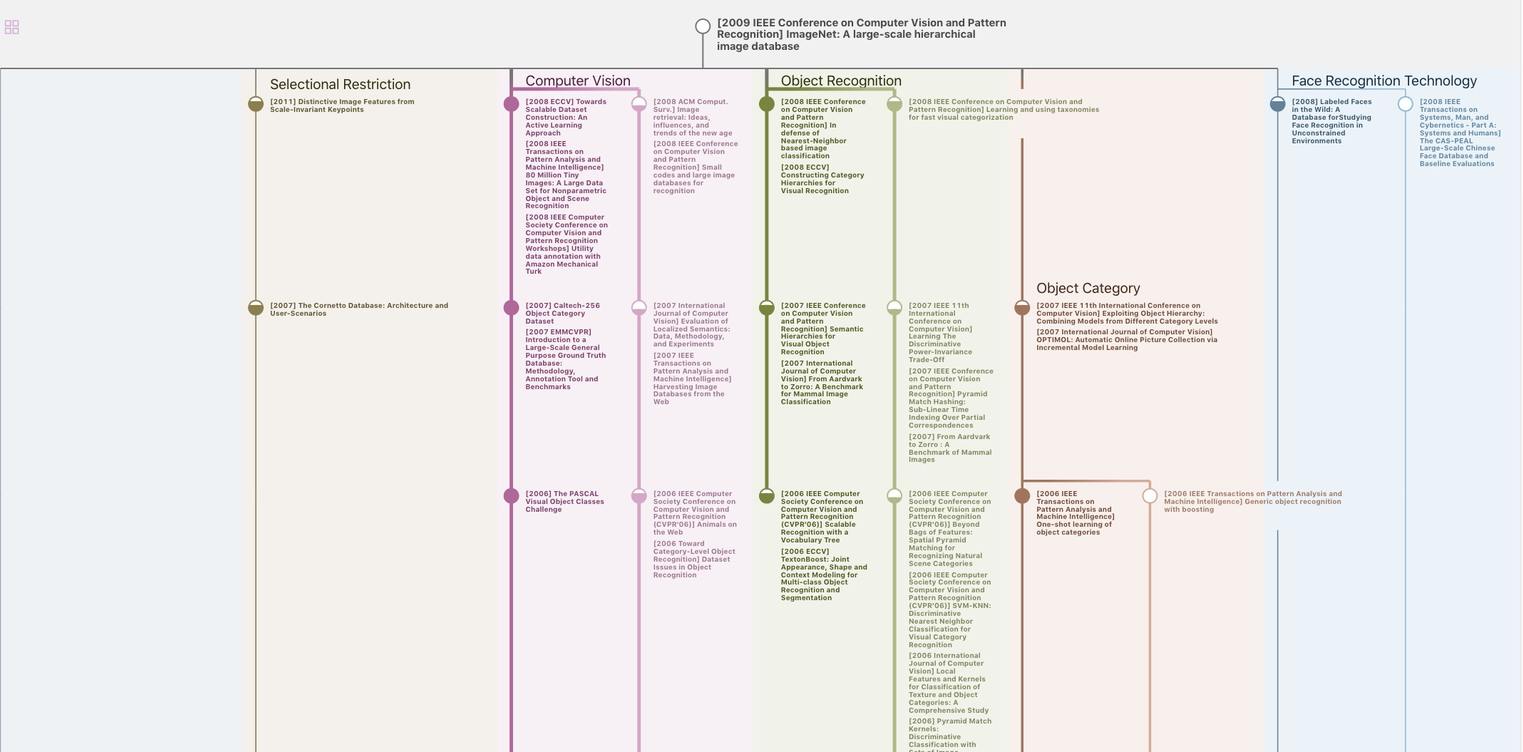
生成溯源树,研究论文发展脉络
Chat Paper
正在生成论文摘要