Rainfall observation using surveillance audio
Applied Acoustics(2022)
摘要
Environmental audio recordings are a rich and underexploited source of rainfall events. Widespread surveillance cameras continuously record rainfall information, which provides a basis for the possibility of rainfall monitoring. In this study, using surveillance audio as input, an automatic rainfall level classification system was built. Through rainfall level definitions, the rainfall observation task can be transformed into an audio classification task. Three 2-D baseline convolutional neural networks (CNNs) were proposed as the classifiers. In view of the classifier training and testing, a new dataset named Rainfall Audio_XZ (RA_XZ) was generated based on the surveillance audio data. Because of the significant impact of the input features on the classification performance, 16 kinds of feature aggregation schemes were constructed and investigated to find the best representation of rainfall. The experimental results demonstrate that the proposed CNN (7-stack CNN) achieves 81.67% accuracy in rainfall level classification in the RA_XZ dataset and 93.38% accuracy in environmental sound classification in the UrbanSound8k dataset, which is optimal performance compared to that of some existing relevant algorithms. Furthermore, audio aggregation strategies that facilitate the representation and classification of rainfall events are investigated, which has important implications for audio and speech classification systems. In summary, our study provides an effective supplement to traditional rainfall monitoring technologies.
更多查看译文
关键词
Rainfall observation,Surveillance audio,CNN,Audio classification
AI 理解论文
溯源树
样例
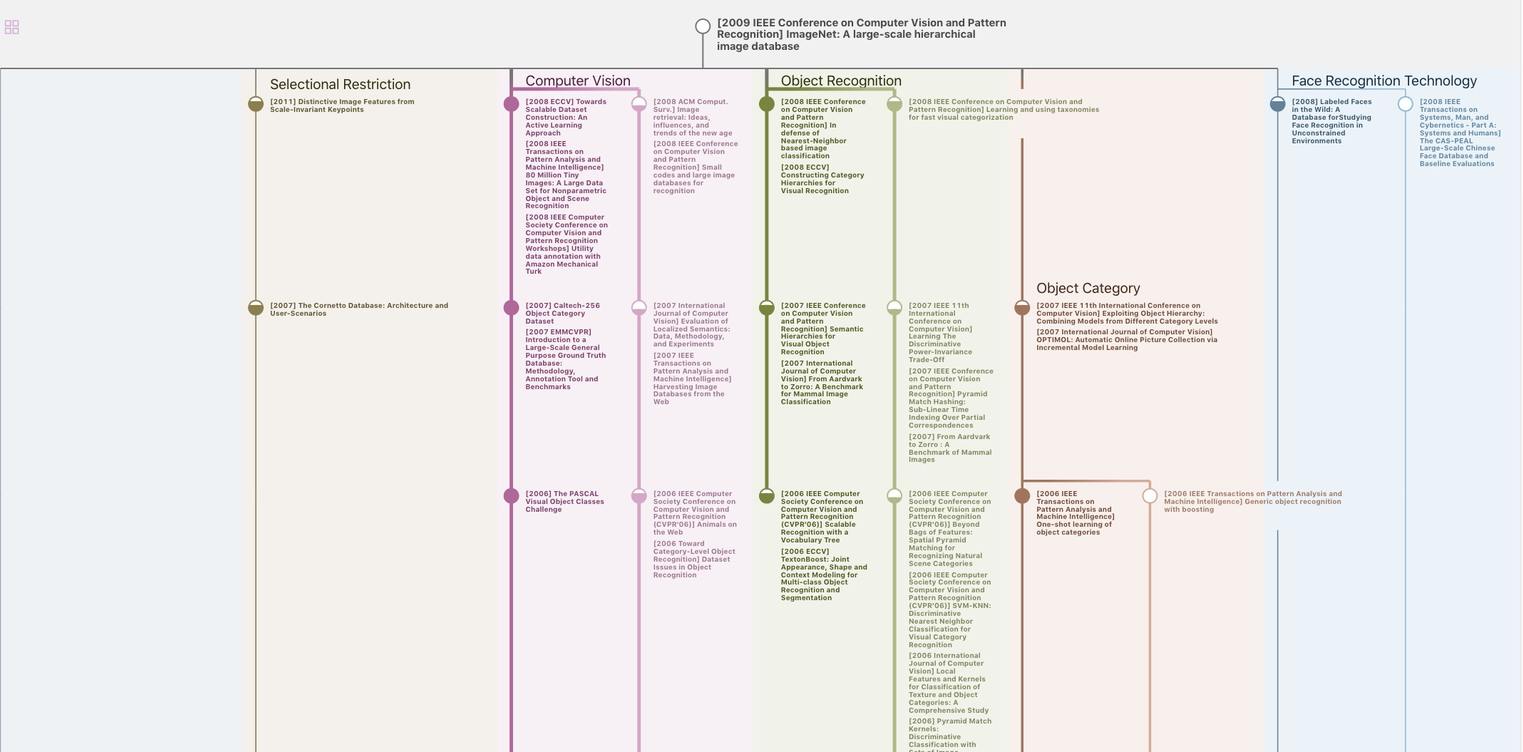
生成溯源树,研究论文发展脉络
Chat Paper
正在生成论文摘要