Language Modeling on Location-Based Social Networks
ISPRS INTERNATIONAL JOURNAL OF GEO-INFORMATION(2022)
摘要
The popularity of mobile devices with GPS capabilities, along with the worldwide adoption of social media, have created a rich source of text data combined with spatio-temporal information. Text data collected from location-based social networks can be used to gain space-time insights into human behavior and provide a view of time and space from the social media lens. From a data modeling perspective, text, time, and space have different scales and representation approaches; hence, it is not trivial to jointly represent them in a unified model. Existing approaches do not capture the sequential structure present in texts or the patterns that drive how text is generated considering the spatio-temporal context at different levels of granularity. In this work, we present a neural language model architecture that allows us to represent time and space as context for text generation at different granularities. We define the task of modeling text, timestamps, and geo-coordinates as a spatio-temporal conditioned language model task. This task definition allows us to employ the same evaluation methodology used in language modeling, which is a traditional natural language processing task that considers the sequential structure of texts. We conduct experiments over two datasets collected from location-based social networks, Twitter and Foursquare. Our experimental results show that each dataset has particular patterns for language generation under spatio-temporal conditions at different granularities. In addition, we present qualitative analyses to show how the proposed model can be used to characterize urban places.
更多查看译文
关键词
spatio-temporal text data, location-based social networks, language models
AI 理解论文
溯源树
样例
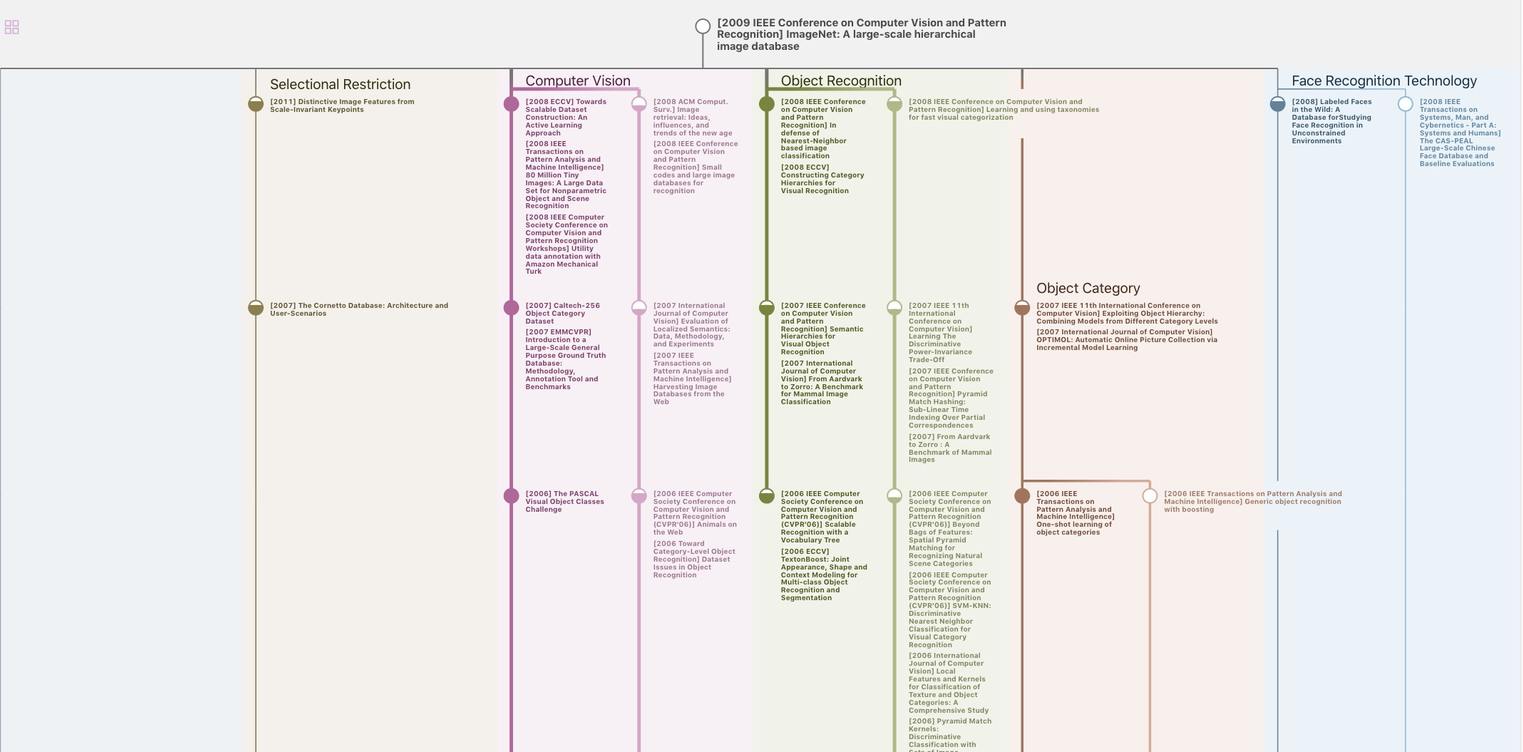
生成溯源树,研究论文发展脉络
Chat Paper
正在生成论文摘要